Modeling faults using 2D and 3D seismic simulation 
crossref(2023)
摘要
<p>In the oil and gas business, 2D and 3D seismic data have become crucial for visualizing and comprehending subsurface morphology and boundaries. In addition, the extensive sampling of 2D and 3D surveys can occasionally make it possible to map reservoir quality and hydrocarbon distribution with clearly indicated constraints and produce outstanding structural images. Here, we avoid frequent mistakes and use 2D and 3D seismic data to map and analyze basic structures and fault lines to build 2D and 3D base fault models for the South Ionian and South Crete areas. This work also emphasizes key ideas and ideas that enable the comparison of various maps to select, interpret, and simulate specific locations that contain hydrocarbon traps. This work also emphasizes key theories and concepts that enable the comparison of various maps to select, interpret, and simulate specific locations that contain hydrocarbon traps. These ideas and ideas include time structure, amplitude, and coherence. The field is inside the block in the West sector of Crete Island and extends around 80.220 km<sup>2</sup>. We demonstrate the existence of a significant fault that splits five horizons of the area of interest based on all the chosen horizons, created maps, and dominating fault construction models (2D and 3D). From the study, we conclude that the application of horizon features in 2D and 3D visualization may easily supply enormous amounts of data to explain the trapping process of faults.</p> <p>References:</p> <p>1. Jonas Köpping, Craig Magee, Alexander R. Cruden, Christopher A.-L. Jackson, James R. Norcliffe; The building blocks of igneous sheet intrusions: Insights from 3-D seismic reflection data. Geosphere 2022;18 (1): 156–182. doi: https://doi.org/10.1130/GES02390.1</p> <p>2. Lotfi, M., Javaherian, A., Varnousfaderani, S. and Amindavar, H., 2022. Mapping channel boundaries in seismic data based on an improved partial area effect. Journal of Petroleum Science and Engineering, 212, p.110263.</p> <p>3. Dariia Voloshchuk, Antonios J. Konstantaras, Alexandra Moshou, Nataliia Kasianova, Irina Skorniakova, Panagiotis Argyrakis, Nikolaos S. Petrakis; Neural network modeling of seismic behaviour of the Hellenic Arc: Strengths and Limitations, International Journal of Advanced Technology and Engineering Exploration, Vol X(XX) ISSN (Print): 2394-5443   ISSN (Online): 2394-7454</p> <p>4. Aliramezani, M., Koch, C. and Shahbakhti, M., 2022. Modeling, diagnostics, optimization, and control of internal combustion engines via modern machine learning techniques: A review and future directions. Progress in Energy and Combustion Science, 88, p.100967.</p> <p>5. Konstantaras, A., Petrakis, N. S., Frantzeskakis, T., Markoulakis, E., Kabassi, K., Vardiambasis, I. O., Theodoros Kapetanakis., Alexandra Moshou and Maravelakis, E. (2021). Deep learning neural network seismic big-data analysis of earthquake correlations in distinct seismic regions. International Journal of Advanced Technology and Engineering Exploration, 8(84), 1410-1423.</p> <p>6. Konstantaras, A. (2020). Deep learning and parallel processing spatio-temporal clustering unveil new ionian distinct seismic zone. Informatics, 7(4)</p> <p>7. Konstantaras, A. J. (2016). Expert knowledge-based algorithm for the dynamic discrimination of interactive natural clusters. Earth Science Informatics, 9(1), 95-100.</p> <p>8. <span id="divtagdefaultwrapper">Konstantaras A., M.R. Varley, F. Vallianatos, G. Collins, P. Holifield. Recognition of electric earthquake precursors using neuro-fuzzy methods: methodology and simulation results. Proc. IASTED Int. Conf. Signal Processing, Pattern Recognition and Applications (SPPRA 2002), Crete, Greece, 303-308, 2002.</span></p> <p> </p>
更多查看译文
AI 理解论文
溯源树
样例
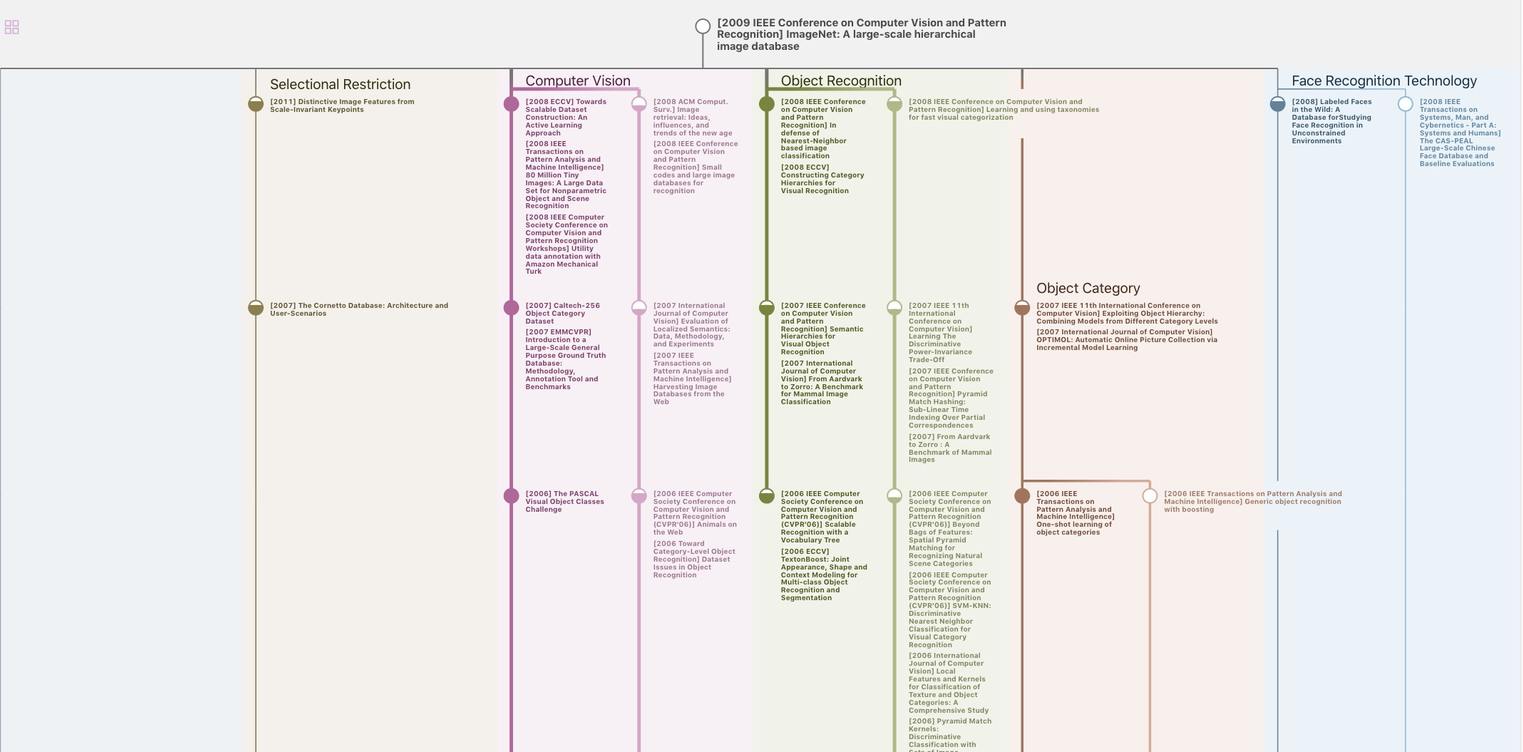
生成溯源树,研究论文发展脉络
Chat Paper
正在生成论文摘要