Light-Weight Learning-Based Depth Estimation From A Single Image
ATHENA Research Book, Volume 1(2022)
摘要
Estimating the depth of a scene from a single image is impossible due to scale ambiguity, but recent deep learning approaches have demonstrated to produce faithful estimates anyways by learning the typical scale of objects from implicit clues in the scene. In this paper, we present a new encoderdecoder architecture for single image depth reconstruction that yields state-ofthe-art results with considerably less parameters than competitors. Our architecture incorporates a modified inception module tailored to our application which allows us to use a relatively lightweight architecture with fewer learnable parameters than state-of-the-art single image depth reconstruction networks. We train and evaluate on the known NYU RGB-D benchmark dataset, and show generalization of our method by evaluating the pretrained NYU model on the iBims dataset. Our results are in the same order of magnitude as stateof-the-art competitors, even though we trained on a different resolution.
更多查看译文
AI 理解论文
溯源树
样例
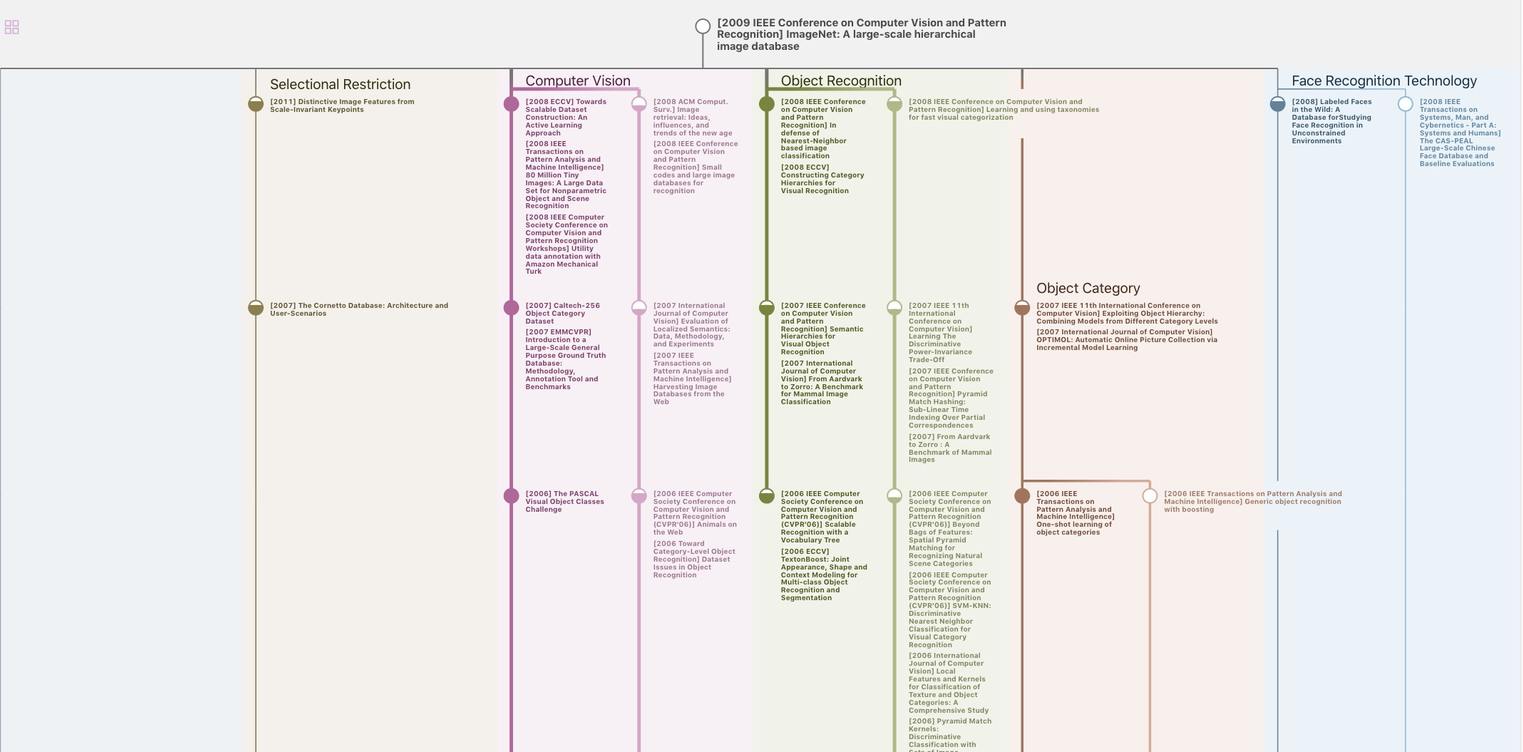
生成溯源树,研究论文发展脉络
Chat Paper
正在生成论文摘要