Correcting PM2.5 data from low-cost sensors using machine learning techniques
crossref(2023)
摘要
<p>Low-cost sensors (LCSs) used for measuring air quality have become popular because of their portability, affordability, and ease of operation. However, LCS data often have accuracy and bias issues that need to be addressed before using them for research. LCSs are, therefore, collocated with reference-grade instruments, and various statistical and machine learning (ML) approaches are used to correct the observed bias in data. In this study, collocation experiments were conducted in Bengaluru, India, for about nine months (December 2021 to August 2022). We used nine PM<sub>2.5</sub> LCSs that were collocated with a beta attenuation monitor (BAM), which is certified by the United States Environmental Protection Agency (USEPA). Hourly averaged data from LCSs and BAM were used to train various ML correction models. The LCSs included in the study—Airveda, Atmos, Prana Air, BlueSky, Aurassure, Aerogram, PurpleAir, and Prkruti—are widely available in the Indian market. The ML models include support vector regression (SVR), decision tree (DT), random forest (RF), and eXtreme gradient boosting (XGBoost). For the LCSs used in the study, a total of 170 ML models were built to identify the best-performing correction model for each sensor. Model performances were evaluated based on the following metrics: mean absolute error (MAE), root mean square error (RMSE), and normalised RMSE (NRMSE). During the study period, the average hourly BAM concentration was ~32 µg/m<sup>3</sup>. Hourly averaged PM<sub>2.5</sub> from LCSs and BAM exhibited a linear relationship. The NRMSE values of the raw (uncorrected) LCSs PM<sub>2.5</sub> with respect to BAM PM<sub>2.5</sub> varied between 0.26 and 0.89 across various sensors. The Plantower-based LCSs (Atmos I, PurpleAir, and Aerogram) performed better, characterised by the lowest RMSE/NRMSE values. SVR was found to be the best-performing model for most of the sensors in correcting raw LCSs PM<sub>2.5 </sub>data. The NRMSE of the ML models’ corrected LCSs PM<sub>2.5</sub> was reduced by 46% to 74% across various sensors compared to the uncorrected LCSs PM<sub>2.5</sub>. As a case study, we also added black carbon (BC) data to our ML models, but no significant change (improvement by 6% RMSE) in performance was observed.</p>
更多查看译文
AI 理解论文
溯源树
样例
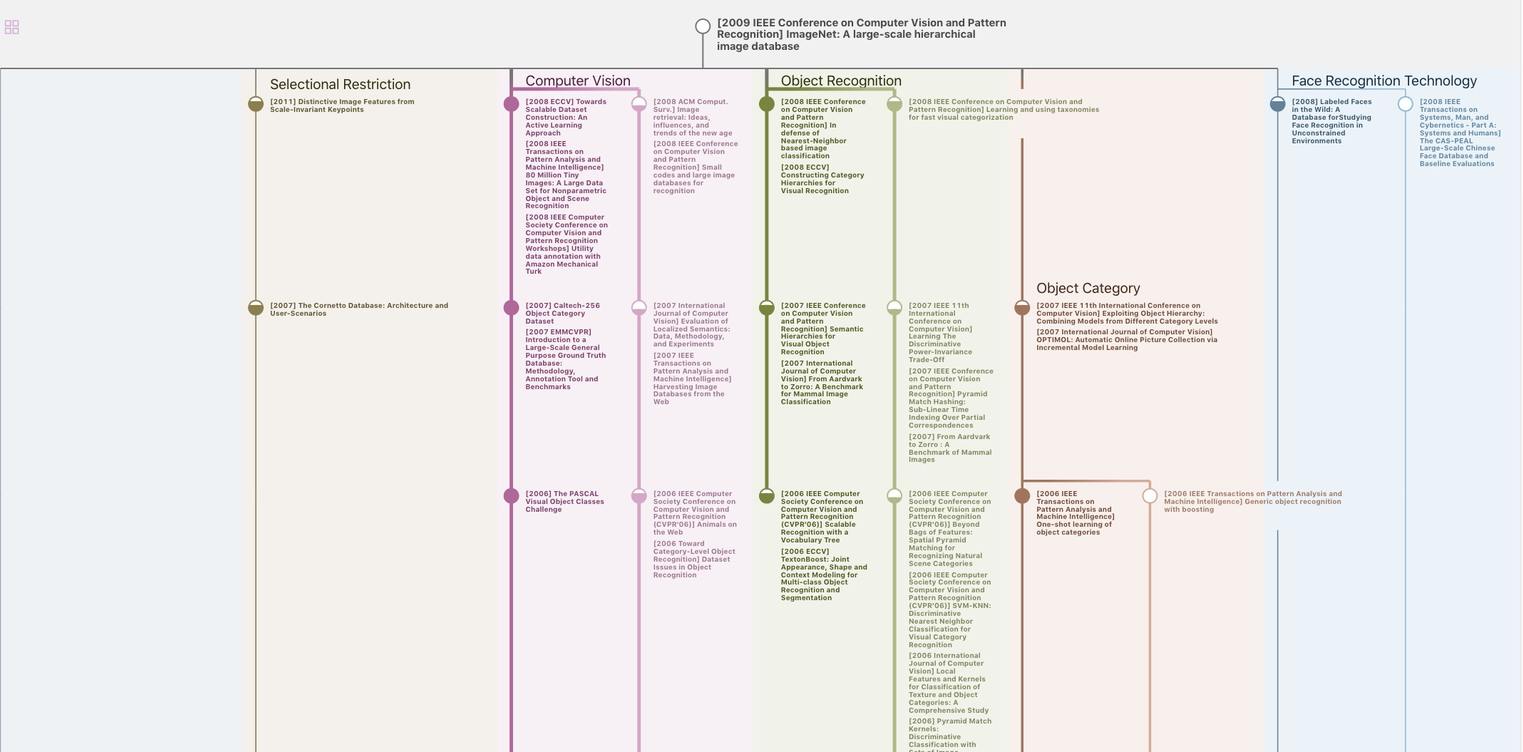
生成溯源树,研究论文发展脉络
Chat Paper
正在生成论文摘要