Towards identification of dominant hydrological mechanisms in ungauged catchments
crossref(2023)
摘要
<p>Modelling hydrological processes in ungauged catchments is a major challenge in environmental sciences and engineering. An ungauged catchment is a catchment that lacks streamflow data suitable for traditional modelling methods. Predicting streamflow in ungauged catchments requires some form of extrapolation ("regionalisation") from other "similar" catchments, with variables of interest being flow "indices" or "signatures", such as quantiles of the flow duration curve, etc.</p> <p>Another major question in hydrology is the estimation of model structure that reflects the hydrological processes relevant to the catchment of interest. This question is intimately tied to process representation. To paraphrase a common saying, all models are wrong, but some model mechanisms (process representations) might be useful. Our previous study contributed a Bayesian framework for the identification of individual model mechanisms from streamflow data.</p> <p>In this study we extend the mechanism identification method to operate in ungauged basins based on regionalized flow indices. Candidate mechanisms and model structures are generated, and then the "dominant" (more a posterior probable) model mechanisms are identified using statistical hypothesis testing. As part of the derivation, it is assumed that the error in the regionalization of flow indices dominates the structural error of the hydrological model.</p> <p>The proposed method is illustrated with real data and synthetic experiments based on 92 catchments from northern Spain, from which 16 catchments are treated as ungauged. We use 624 model structures from the flexible hydrological model framework FUSE. Flow indices are regionalised using random forest regression in principal component (PC) space; we select the first 4 leading indices in PC space. The case study set up includes an experiments using real data (where the true mechanisms are unknown) and a set of synthetic experiments with different error levels (where the “true” mechanisms are known).</p> <p>Across the real and synthetic experiments, routing is usually among the most identifiable processes, whereas the least identifiable processes are percolation and unsaturated zone processes. The precision, i.e. the probability of making an identification (whether correct or not), remains stable at around 25%. In the synthetic experiments we can calculate the (conditional) reliability of the identification method, i.e. the probability that, when the method makes an identification, the true mechanism is identified. The conditional reliability varies from 60% to 95% depending on the magnitude of the combined regionalization and hydrological error. Our study contributes perspectives on hydrological mechanism identification under data-scarce conditions; we discus limitations and opportunities for improvement.</p> <p> </p> <p>Prieto, C., N. Le Vine, D. Kavetski, F. Fenicia, A. Scheidegger, and C. Vitolo (2022) An Exploration of Bayesian Identification of Dominant Hydrological Mechanisms in Ungauged Catchments, <em>Water Resources Research</em>, <em>58</em>(3), e2021WR030705, doi: https://doi.org/10.1029/2021WR030705.</p>
更多查看译文
AI 理解论文
溯源树
样例
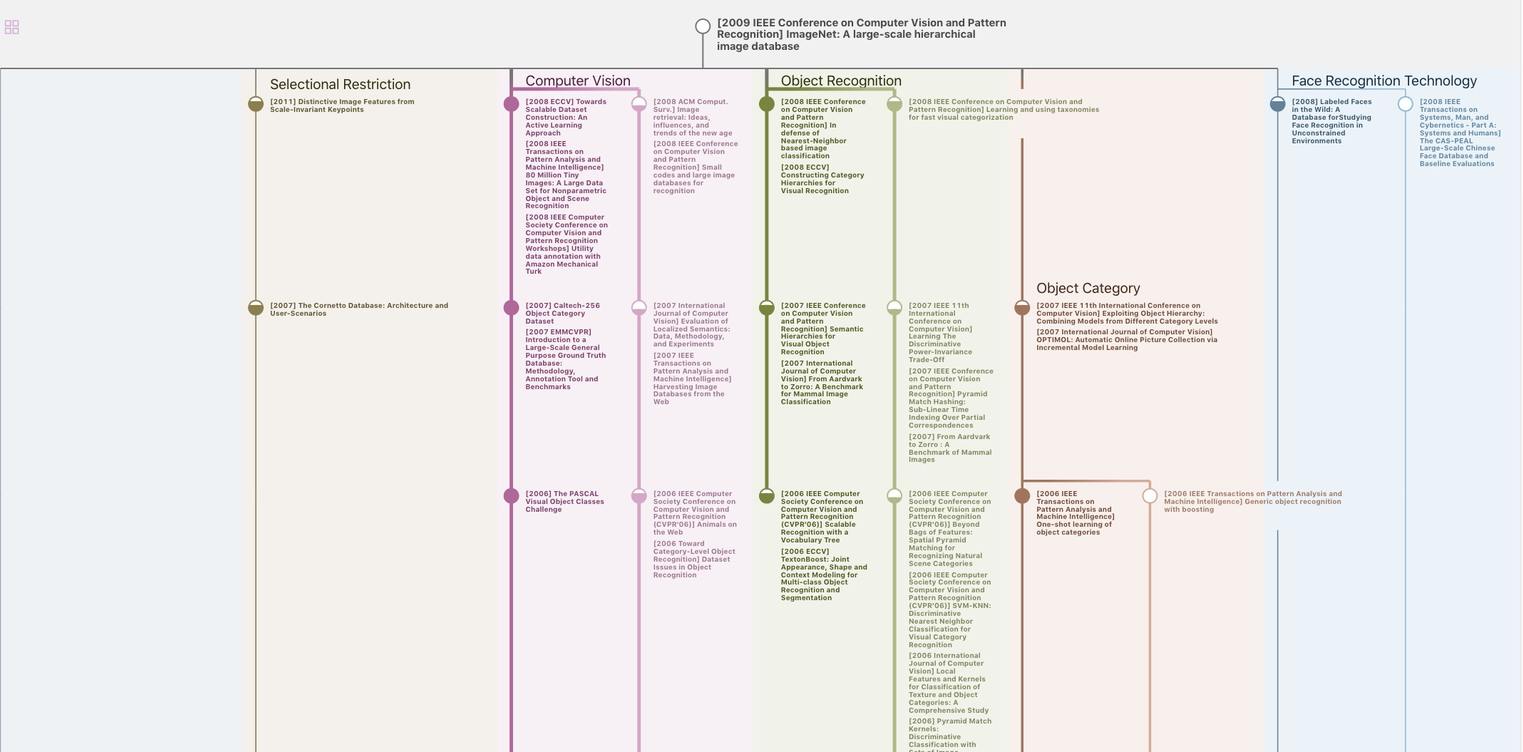
生成溯源树,研究论文发展脉络
Chat Paper
正在生成论文摘要