Prototype Learning for Automatic Check-Out
IEEE TRANSACTIONS ON MULTIMEDIA(2023)
摘要
The basic goal of Automatic Check-Out (ACO) task is to accurately predict the categories and quantities of products selected by customers in the check-out images. However, there is a significant domain gap between the single-product exemplars as training data and the check-out images as testing data. To mitigate the domain gap, we propose a novel method termed as Prototype Learning for Automatic Check-Out (PLACO). In PLACO, prototype learning is designed to reach the goal in two ways. Specifically, in the prototype-based classifier learning module, to fully exploit the invariance of category prototypes, the prototypes obtained from the single-product exemplars are employed to generate classifiers for classifying the proposals of check-out image. On the other side, in prototype alignment module, prototypes for both the single-product exemplar and check-out image domains are entered simultaneously to ensure intra-category compactness and inter-category sparsity. Moreover, to further improve the performance of PLACO, we develop a discriminative re-ranking module to both adjust the predicted scores of product proposals for bringing more discriminative ability in classifier learning and provide a reasonable sorting possibility by considering the fine-grained nature. Experiments are conducted on the large-scale RPC dataset for evaluations. Our PLACO obtains the optimal results in both traditional ACO task setting and incremental task setting.
更多查看译文
关键词
Automatic check-out,prototype learning,classifier learning,prototype alignment,discriminative re-ranking
AI 理解论文
溯源树
样例
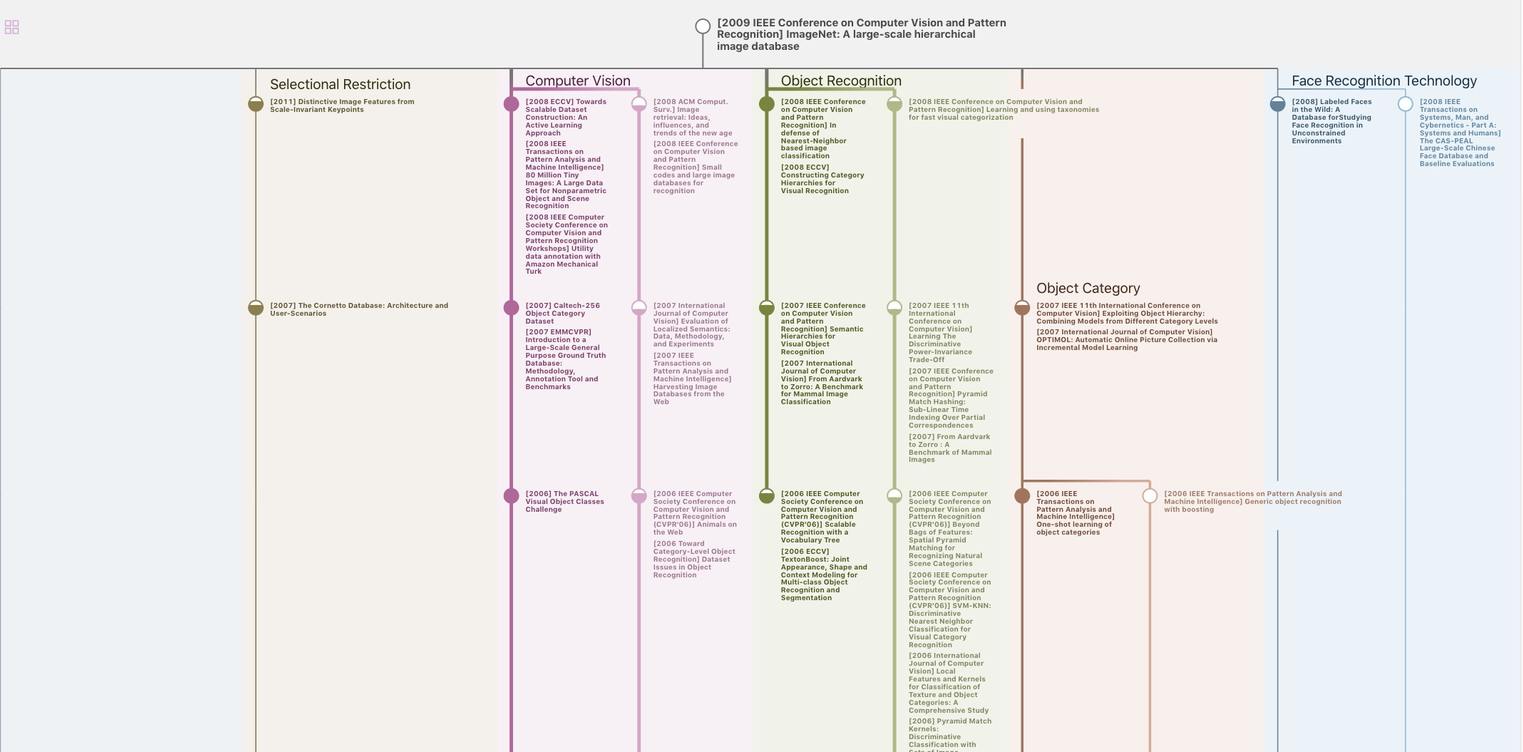
生成溯源树,研究论文发展脉络
Chat Paper
正在生成论文摘要