Quantifying the effects of pressure management for the Williston basin Brine Extraction and Storage Test (BEST) site using machine learning
INTERNATIONAL JOURNAL OF GREENHOUSE GAS CONTROL(2024)
摘要
Active reservoir management (ARM) through brine extraction can reduce pressure buildup during large-scale implementation of carbon capture and storage (CCS) projects. This study used machine learning (ML)-assisted approaches to analyze bottomhole pressure (BHP) responses to various brine injection and extraction scenarios. Field monitoring data were collected over a 2-year operation period at two injection wells and one extraction well (about 400 m away) as part of a Brine Extraction and Storage Test (BEST) in the North Dakota portion of the Williston Basin. Injection activities increased the BHPs at the injection wells by around 0.70 MPa (similar to 100 psi) during the operation period. Extraction activities demonstrated the capability to decrease the BHPs at the injection wells by approximately 0.21-0.34 MPa (30-50 psi) depending on the ratio of the extraction and injection well flow rates (the '' extraction ratio '' - a normalization procedure used in the analysis). The pressure reduction provided by the extraction well equated to 30-50 % of the pressure buildup at the injection well. This work shows how ML analytics can play a key role in estimating reservoir pressure responses for complex injection and extraction activities during pressure management practices of CCS projects.
更多查看译文
关键词
Carbon capture and storage,Pressure management,Bottomhole pressure,Brine extraction,Machine learning
AI 理解论文
溯源树
样例
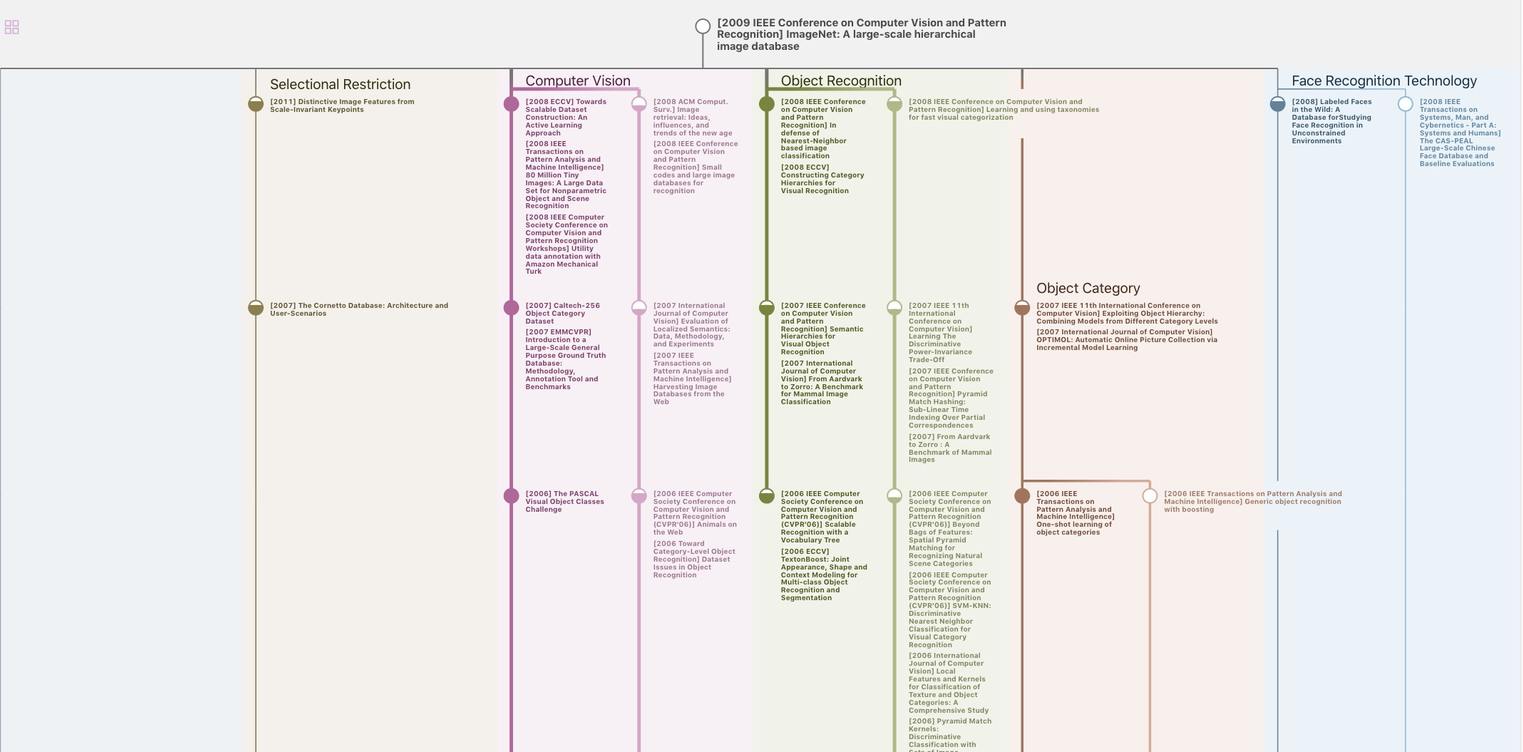
生成溯源树,研究论文发展脉络
Chat Paper
正在生成论文摘要