ReSQ: Reinforcement Learning-Based Queue Allocation in Software-Defined Queuing Framework
Journal of networking and network applications(2022)
摘要
With the evolution of 5G networks, the demand for Ultra-Reliable Low Latency Communications (URLLC) services is increasing. Software-Defined Networking (SDN) offers flexible network management to prioritize URLLC services coexisting with best-effort traffic. Utilizing the network programmability feature of SDN, Software-Defined Queueing (SDQ) framework selects the optimal output port queue on forwarding devices and routing path for incoming traffic flows to provide deterministic Quality of Service (QoS) support required for URLLC traffic. However, in the existing SDQ framework, the selections of optimal queue and path are done manually by observing the traffic type of each incoming flow, the available bandwidth of each potential routing path, and the status of output port queues of each forwarding device on each potential routing path. The static allocations of path and queue for each flow are inefficient to provide a deterministic QoS guarantee for a high volume of incoming traffic which is typical in 5G networks. The limited buffer availability on the forwarding devices is another constraint regarding optimal queue allocation that ensures an end-to-end (E2E) delay guarantee. To address these challenges, in this paper, we extend the SDQ framework by automating queue management with a reinforcement learning (RL)-based approach. The proposed queue management approach considers diverse QoS demands as well as a limited buffer on the forwarding devices and performs prioritized queue allocation. Our approach also includes a hash-based flow grouping to handle a high volume of traffic having diverse latency demands and a path selection mechanism based on available bandwidth and hop count. The simulation result shows that the proposed scheme ReSQ reduces the QoS violation ratio by 10.45% as compared to the baseline scheme that selects queues randomly.
更多查看译文
AI 理解论文
溯源树
样例
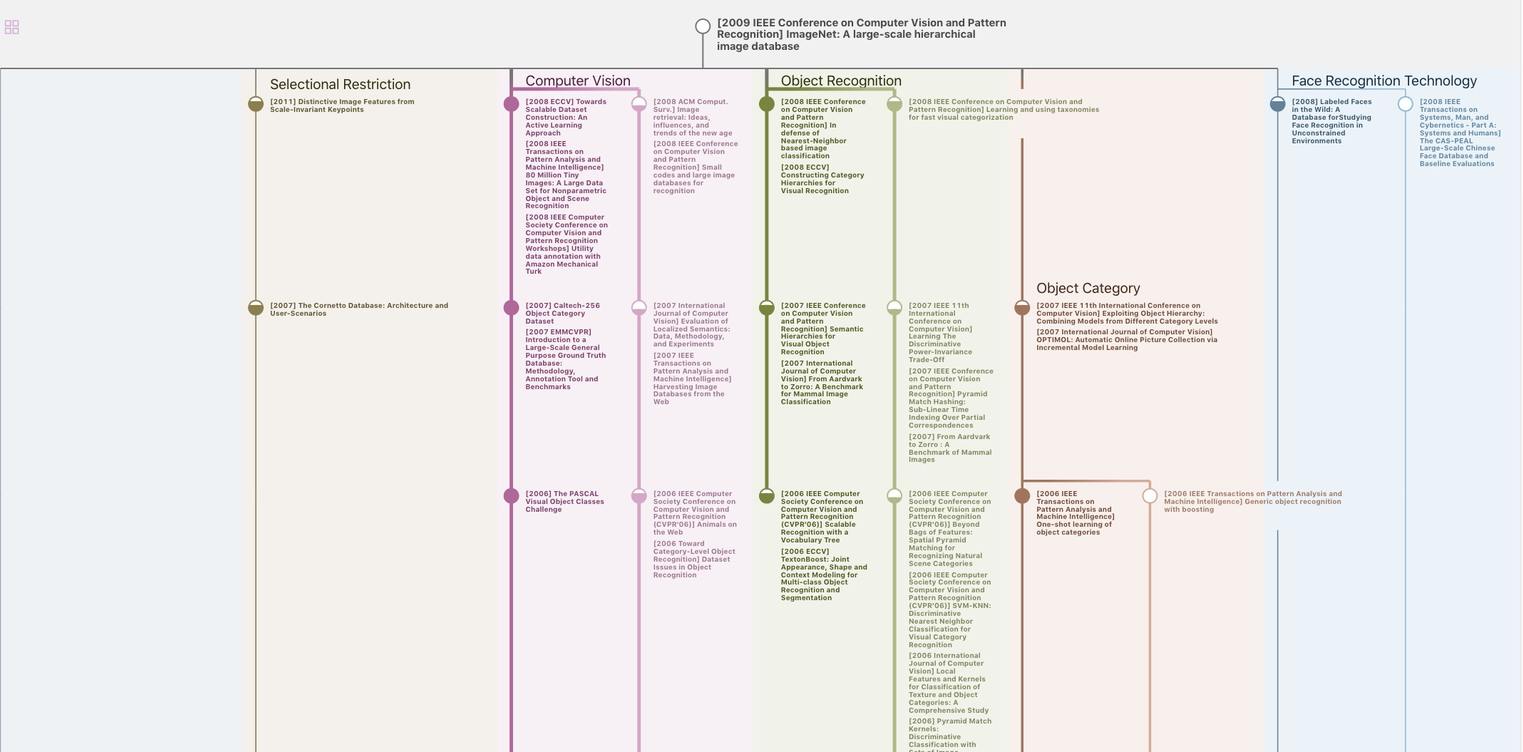
生成溯源树,研究论文发展脉络
Chat Paper
正在生成论文摘要