Offline-Online Actor-Critic.
IEEE Transactions on Artificial Intelligence(2024)
摘要
Offline–online reinforcement learning (RL) can effectively address the problem of missing data (commonly known as transition) in offline RL. However, due to the effect of distribution shift, the performance of policy may degrade when an agent moves from offline to online training phases. In this article, we first analyze the problems of distribution shift and policy performance degradation in offline–online RL. Then, in order to alleviate these problems, we propose a novel RL algorithm offline–online actor–critic (O2AC) algorithm. In O2AC, a behavior clone constraint term is introduced into the policy objective function to address the distribution shift in offline training phase. In addition, in online training phase, the influence of the behavior clone constraint term is gradually reduced, which alleviates the policy performance degradation. Experiments show that O2AC outperforms existing offline–online RL algorithms.
更多查看译文
关键词
Actor–critic,behavior clone (BC) constraint,distribution shift,offline–online reinforcement learning (RL),policy performance degradation
AI 理解论文
溯源树
样例
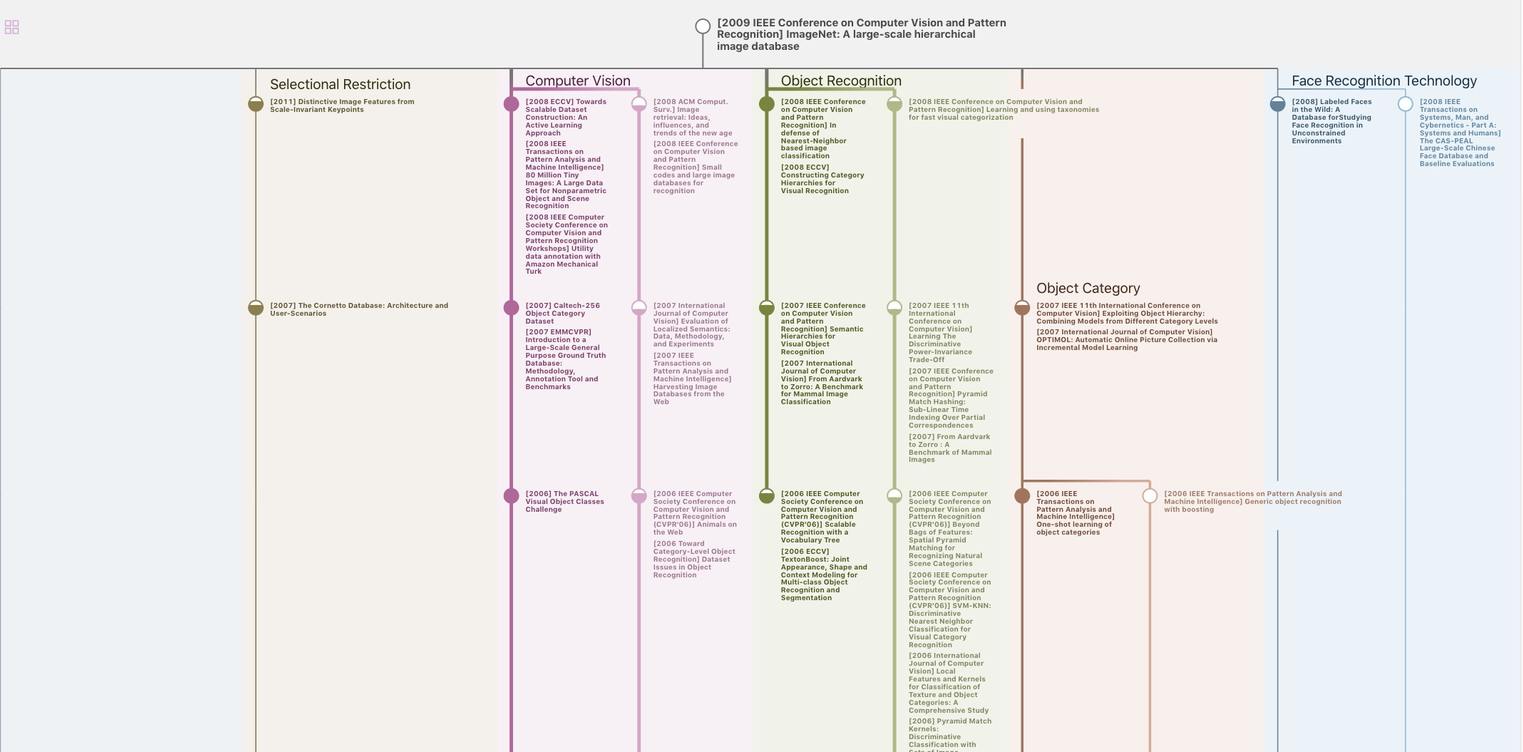
生成溯源树,研究论文发展脉络
Chat Paper
正在生成论文摘要