Explainability of Image Classifiers for Targeted Adversarial Attack
2022 IEEE 19th India Council International Conference (INDICON)(2022)
摘要
Deep neural networks outperform their contemporary peer models for computer vision tasks. These models are complex and challenging to interpret for practitioners. As more and more learning algorithms are deployed in real-world applications, model interpretability has become quite essential. Incidentally, Model interpretability is quite relevant to the case of deep neural networks as they can fall prey to adversarial attacks crafted by adversaries. In this paper, we launch an iterative targeted attack using a set of image classes on base architectures and interpret the results by applying an explanation algorithm before and after the attack. This process leads us to some valuable conclusions regarding the effects of the attack on the explanation methods and how explanation methods can be made to have more adversarial robustness.
更多查看译文
关键词
targeted adversarial attack,image classifiers,explainability
AI 理解论文
溯源树
样例
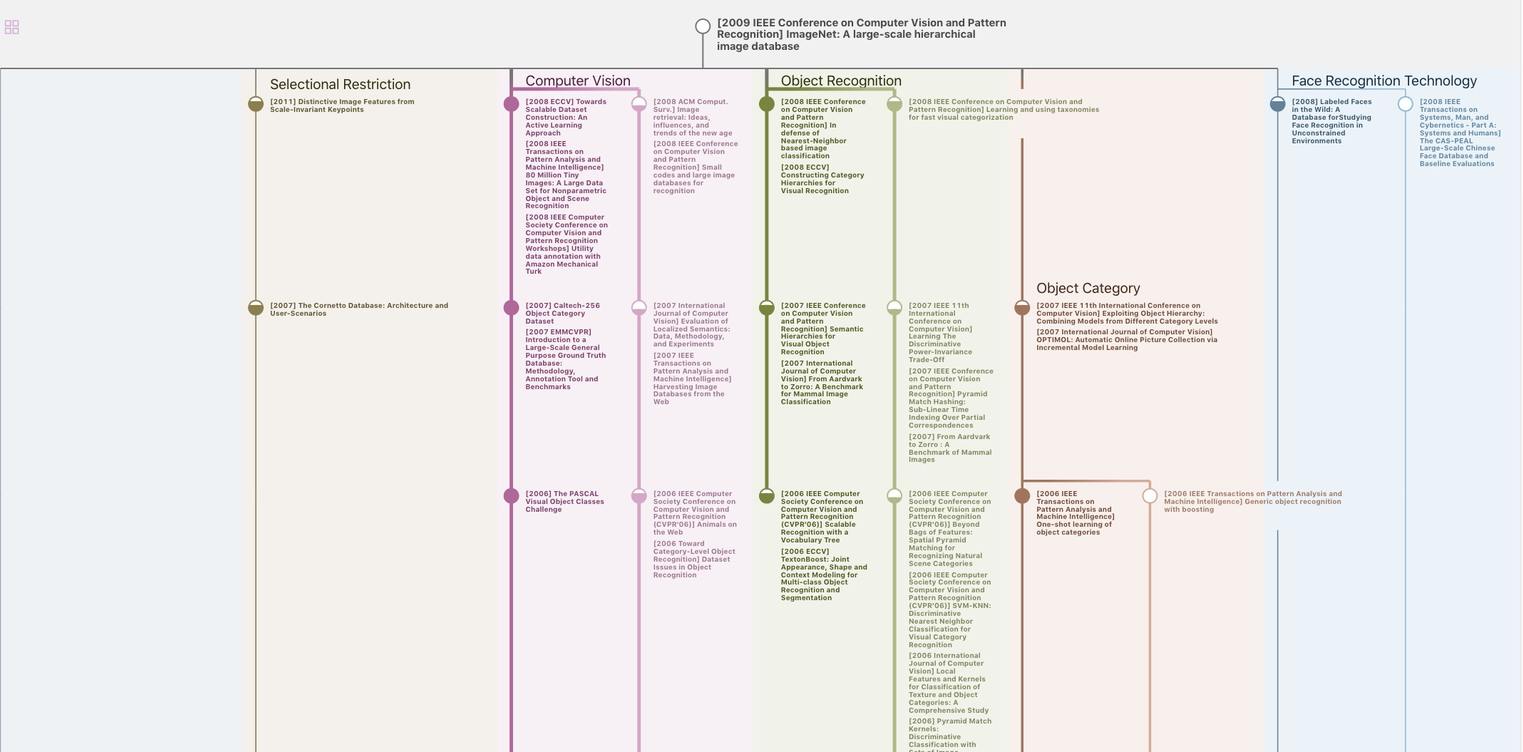
生成溯源树,研究论文发展脉络
Chat Paper
正在生成论文摘要