Preserving Differential Privacy in Deep Learning Based on Feature Relevance Region Segmentation
IEEE TRANSACTIONS ON EMERGING TOPICS IN COMPUTING(2024)
摘要
In the era of Big Data, deep learning techniques provide intelligent solutions for various problems in real-life scenarios. However, deep neural networks depend on large-scale datasets including sensitive data, which causes the potential risk of privacy leakage. In addition, various constantly evolving attack methods are also threatening the data security in deep learning models. Protecting data privacy effectively at a lower cost has become an urgent challenge. This article proposes an Adaptive Feature Relevance Region Segmentation (AFRRS) mechanism to provide differential privacy preservation. The core idea is to divide the input features into different regions with different relevance according to the relevance between input features and the model output. Less noise is intentionally injected into the region with stronger relevance, and more noise is injected into the regions with weaker relevance. Furthermore, we perturb loss functions by injecting noise into the polynomial coefficients of the expansion of the objective function to protect the privacy of data labels. Theoretical analysis and experiments have shown that the proposed AFRRS mechanism can not only provide strong privacy preservation for the deep learning model, but also maintain the good utility of the model under a given moderate privacy budget compared with existing methods.
更多查看译文
关键词
Deep learning,differential privacy,privacy leakage,feature relevance region segmentation
AI 理解论文
溯源树
样例
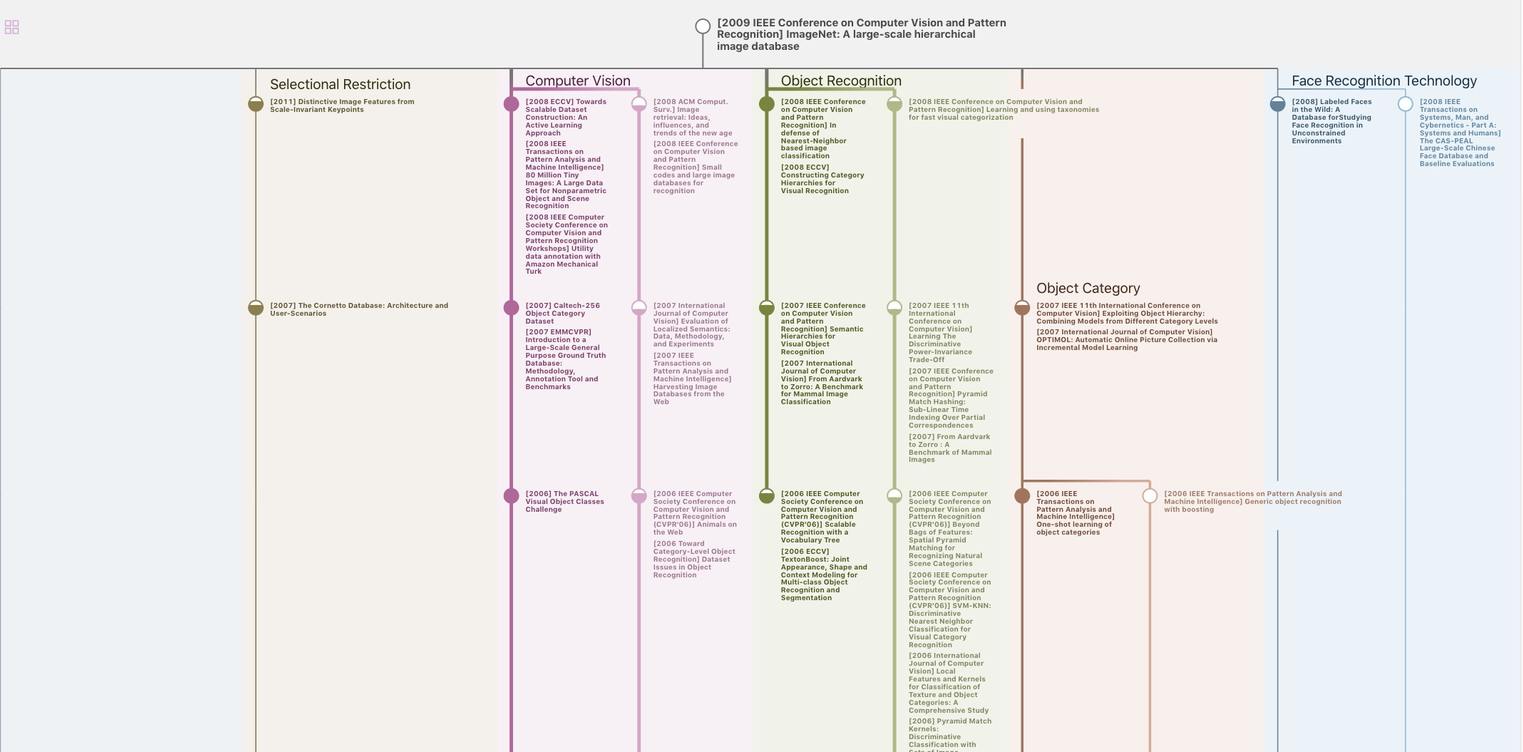
生成溯源树,研究论文发展脉络
Chat Paper
正在生成论文摘要