Optimizing the Design of Anode-Free Sodium Batteries Using Machine Learning Methods
ECS Meeting Abstracts(2022)
摘要
The anode-free sodium battery has been demonstrated as a practical next-generation battery due to (1) the electrochemical stability of Na metal in standard electrolytes, (2) comparable or greater energy density than commercial Li-ion, and (3) the ability to leverage standard industrial Li-ion manufacturing techniques to scale production [1-2]. However, a key challenge in this system centers on the design of a thin coating, called a nucleation layer, on the negative terminal current collector that guides the reversible nucleation and growth of sodium metal. Whereas researchers have highlighted Coulombic efficiencies exceeding 99.9% with sodium metal, understanding and controlling this heterogeneous nucleation process is a critical barrier to the practical design of stable, high performance sodium batteries. In this work, we discuss our efforts in using a Bayesian optimization algorithm to accelerate the search for the optimal properties of a thin nucleation layer in a sodium metal half-cell to optimize anode-free cell performance. Using this sequential design approach, we are able to quickly and concurrently optimize multiple interacting properties of the nucleation layer that affect reversible sodium deposition, such as carbon material composition, oxygen content, and surface area to yield a best performing architecture for an anode-free sodium cell. Further, I will also discuss the important role of pressure on the nucleation and growth of sodium metal, emphasizing the need for better understanding at the interface between mechanics and electrochemistry for anode-free lithium and sodium cells. References [1] A.P. Cohn, N. Muralidharan, R. Carter, K. Share, and C.L. Pint, “Anode-free sodium battery through in situ plating of sodium metal,” Nano Lett. 17, 1296 – 1301 (2017). [2] A.P. Cohn, T. Metke, J. Donohue, N. Muralidharan, K. Share, and C.L. Pint, “Rethinking sodium-ion anodes as nucleation layers for anode-free batteries,” J. Mater. Chem. A 6, 23875 – 23884 (2018).
更多查看译文
关键词
machine learning methods,machine learning,sodium,anode-free
AI 理解论文
溯源树
样例
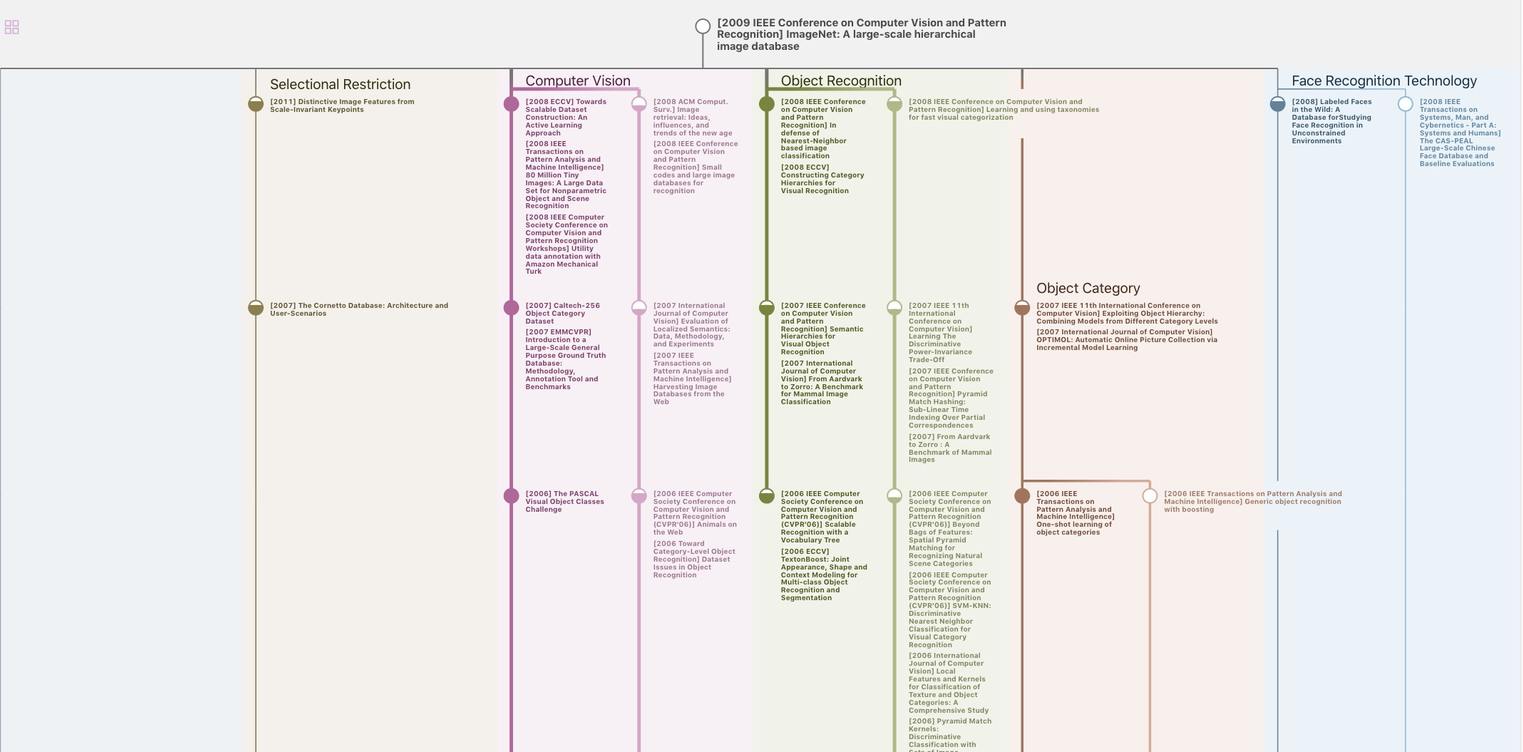
生成溯源树,研究论文发展脉络
Chat Paper
正在生成论文摘要