Deep Learning Assisted High Throughput Screening of Ionic Liquid Electrolytes for Nrr and Co2rr
SSRN Electronic Journal(2023)
摘要
Nonaqueous ionic liquids (ILs) with high solubility of both N2 and CO2 have a greater potential to be used as electrolytes for nitrogen reduction reaction (NRR) and electrocatalytic CO2 reduction reaction (CO2RR) due to the reduced effects of hydrogen evolution reactions (HER) and the high conductivity as well as the low viscosity. However, conventional experimental methods for screening ILs electrolytes are time consuming and labor intensive. In this work, a deep learning-assisted ILs screening approach was investigated to find the ILs electrolytes in practical applications. About 40,000 experimental data were collected for six ILs properties including viscosity, conductivity, melting point, density, N2 solubility and CO2 solubility. Graph neural network (GNN) was used to predict the properties of ILs and exhibited superior performance compared to traditional machine learning models. In addition, the transfer learning (TL) approach was employed to enhance the model prediction on a small dataset. To achieve high-throughput screening, a virtual database was constructed with 2 million ILs structures. Taking the properties of [P6,6,6,14][eFAP], the IL with higher Faradaic conversion efficiency, as the input thresholds, we performed high throughput screening of the virtual dataset, obtained 141 ILs based on the Synthetic Complexity Score (SCScore), in which [B(CN)4]- ILs and [ClO4]- ILs accounted for 29.3% and 15.7%, respectively, and finally identified 8 ILs superior to [P6,6,6,14][eFAP] as ideal electrolytes materials for both NRR and CO2RR that had been reported to be synthesizable. This data-driven model can streamline electrolytes selection and design, accelerating the development of IL electrolytes for electrochemical systems.
更多查看译文
关键词
Deep learning, Transfer learning, High throughput screening, Ionic liquids electrolytes
AI 理解论文
溯源树
样例
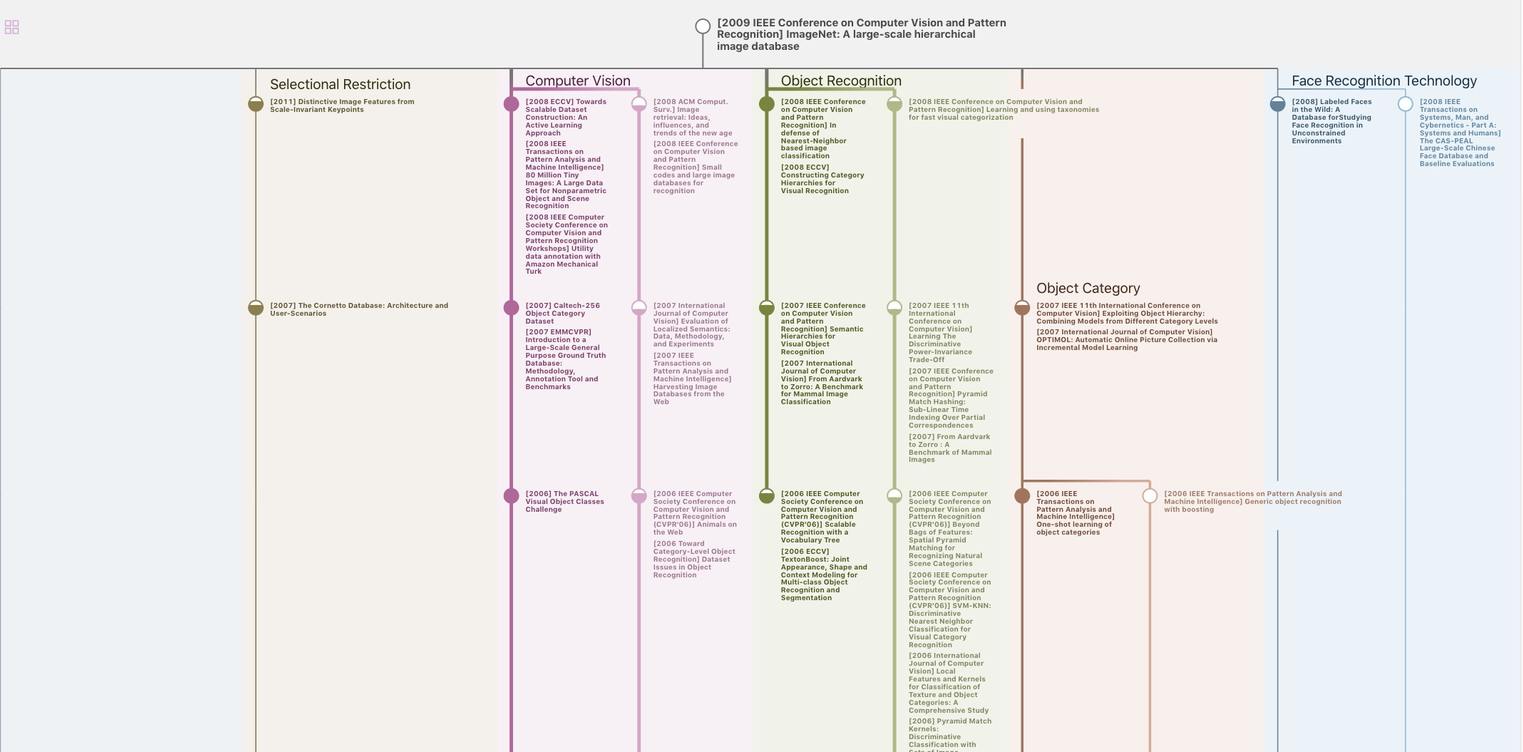
生成溯源树,研究论文发展脉络
Chat Paper
正在生成论文摘要