X-Processes: Process Model Discovery with the Best Balance Among Fitness, Precision, Simplicity, and Generalization Through a Genetic Algorithm
SSRN Electronic Journal(2023)
摘要
Although process model discovery has been largely investigated over the past two decades, existing process discovery methods are not yet considered fully satisfactory. A particular issue is the difficulty of discovering process models with a good balance among recall (or fitness), precision, generalization, and simplicity, notably for real-world event logs. This article revisits the genetic algorithms-based X-Processes process discovery method, discussing the results of using a fitness function calculated through a harmonic mean that considers the four main metrics of process model quality used in process mining — recall, precision, generalization and simplicity. Although genetic algorithms have been used to discover process models, such methods have limitations, as do other non-genetic algorithm-based methods. X-Processes was tested with two harmonic mean options — one based on recall and precision only and another based on the four quality metrics. X-Processes showed superior effectiveness when compared to state-of-the-art process discovery methods, including one also based on genetic algorithms. The experimental results obtained for both harmonic mean options, using 12 real-world event logs, show that the overall quality of all process models discovered by X-Processes is superior to that of the baseline methods. While its execution time is longer than the other compared process discovery methods, X-Processes emerges as a solution when the need for a higher quality process model outweighs the hunger for agility.
更多查看译文
关键词
Process mining,Automated process discovery,Genetic algorithms,Fitness,Recall,Precision,Accuracy,Generalization,Simplicity
AI 理解论文
溯源树
样例
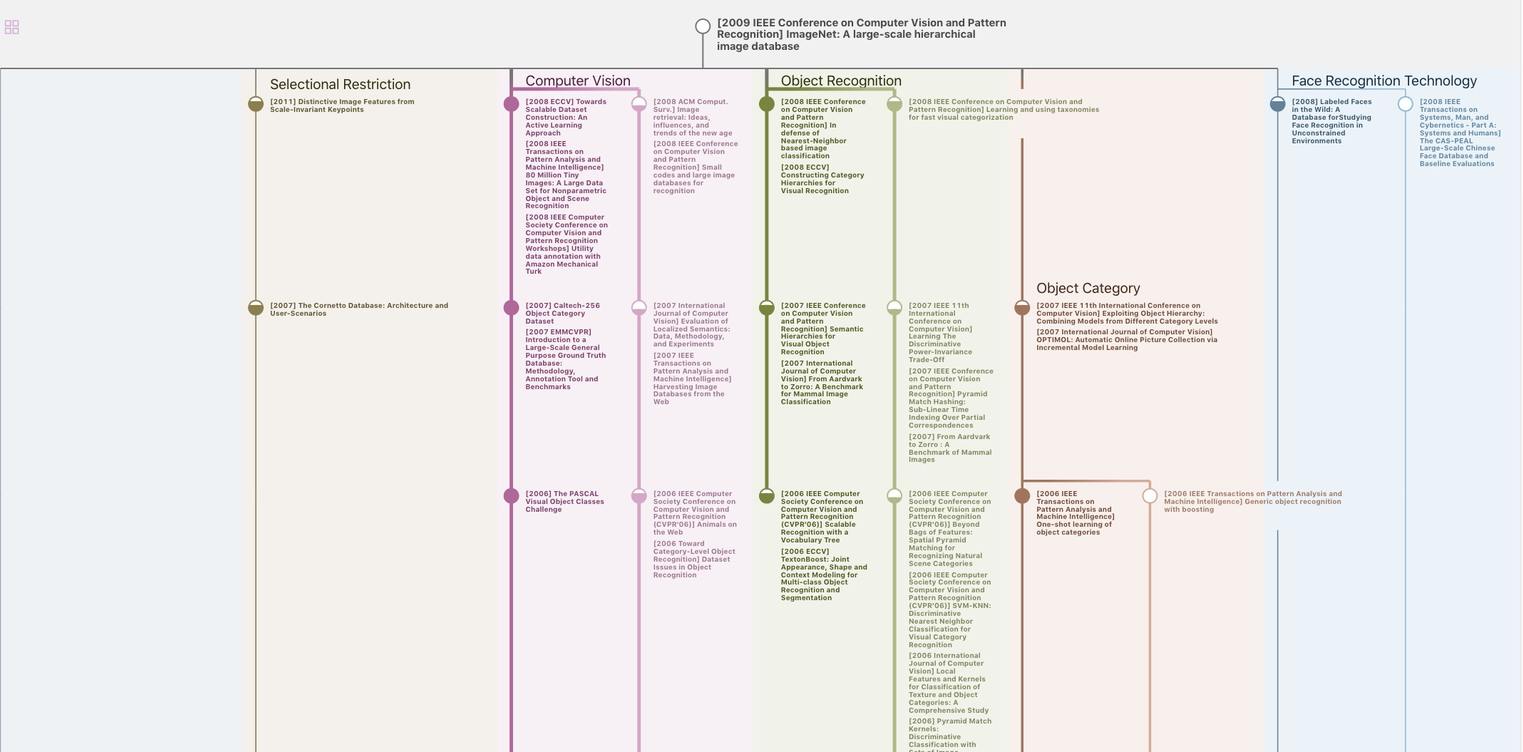
生成溯源树,研究论文发展脉络
Chat Paper
正在生成论文摘要