Modulation signal recognition based on lightweight complex residual attention neural network
2022 2nd International Conference on Computer Science, Electronic Information Engineering and Intelligent Control Technology (CEI)(2022)
摘要
To address the problem of poor recognition performance of real-valued deep learning models in modulation recognition applications and the practical need for lightweight in real applications, this paper proposes a lightweight complex residual attention neural network model. By constructing a complex convolution module, the complex convolution operation of the convolution layer is implemented to extract features in the complex domain of the modulated signal, and a residual attention mechanism is introduced to further select key features for recognition and classification. The experimental results show that compared with the real-valued deep learning model, the proposed model extracts more spatial variability in signal classification features and is easier to recognize, and increases the recognition rate by 10% when the signal-to-noise ratio is greater than OdB. In addition, the resource consumption of the model is reduced. Compared with the basic model, the model parameters are reduced by 99%, and the floating-point operation is reduced by 56%.
更多查看译文
关键词
modulation signal recognition,lightweight complex residual attention,neural network
AI 理解论文
溯源树
样例
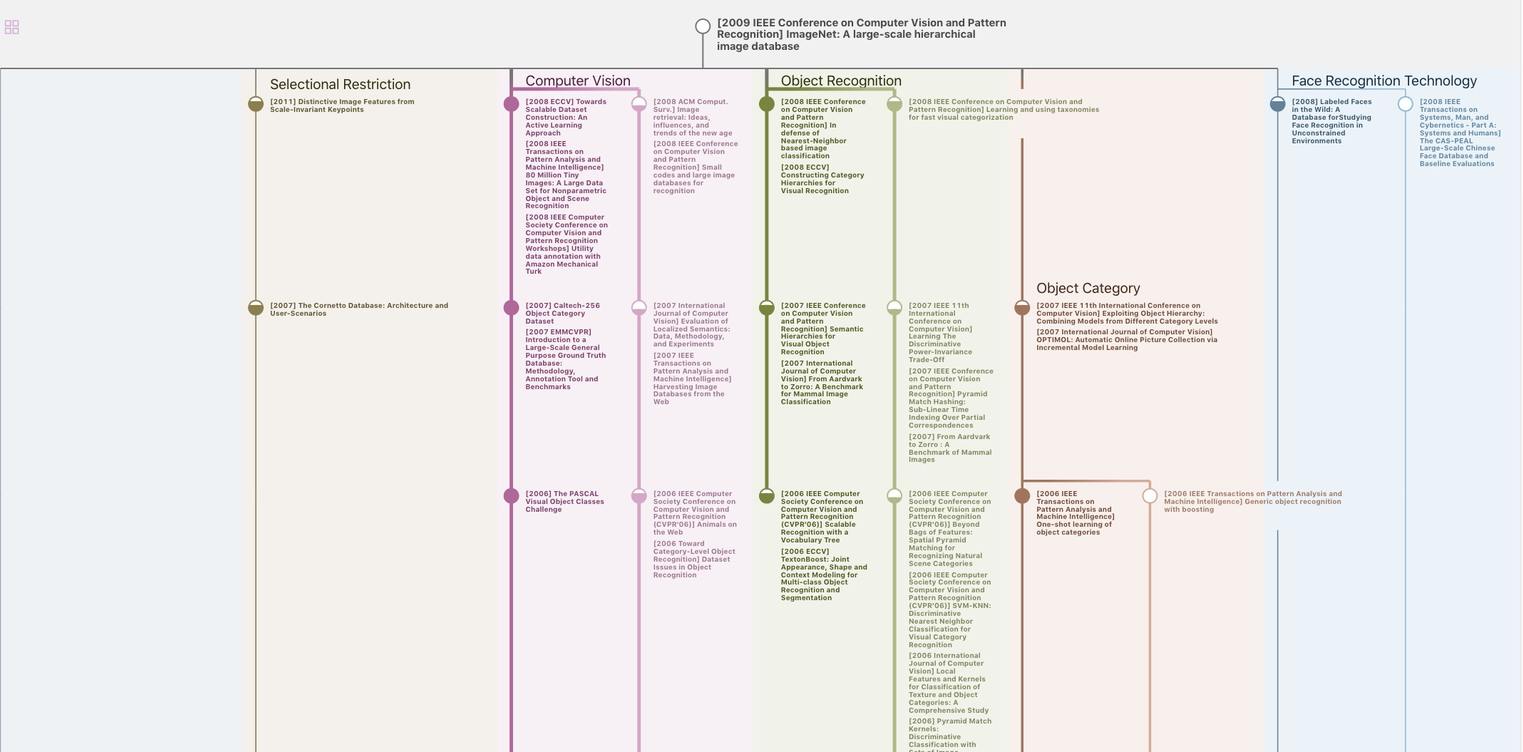
生成溯源树,研究论文发展脉络
Chat Paper
正在生成论文摘要