Source distribution weighted multisource domain adaptation without access to source data
Handbook of Statistics(2023)
Abstract
Unsupervised domain adaptation (UDA) aims to learn a predictive model for an unlabeled domain by transferring knowledge from a separate labeled source domain. Conventional UDA approaches make the strong assumption of having access to the source data during training. This may not be very practical due to privacy, security, and storage concerns. A recent line of work addressed this problem and proposed an algorithm that transfers knowledge to the unlabeled target domain from a single-source model without requiring access to the source data. However, for adaptation purposes, if there are multiple trained source models available to choose from, this method has to go through adapting each and every model individually, to check for the best source. A better question to ask is the following: can we find the optimal combination of source models, with no source data and without target labels, whose performance is no worse than the single best source? The answer to this is given by a recent efficient algorithm (Ahmed et al., 2021) which automatically combines the source models with suitable weights in such a way that it performs at least as good as the best source model. The work provided intuitive theoretical insights to justify their claim and extensive experiments were conducted on several benchmark datasets. In this chapter, we first review this work on multi-source source-free unsupervised domain adaptation followed by analysis of a new algorithm which we propose by relaxing some of the assumptions of this prior work. More specifically, instead of naively assuming source data distribution as uniform, we try to estimate it via a multilayer perceptron (MLP) in order to use this information for effective aggregation of source models.
MoreTranslated text
Key words
Source free adaptation,Multi source adaptation,Unsupervised domain adaptation
AI Read Science
Must-Reading Tree
Example
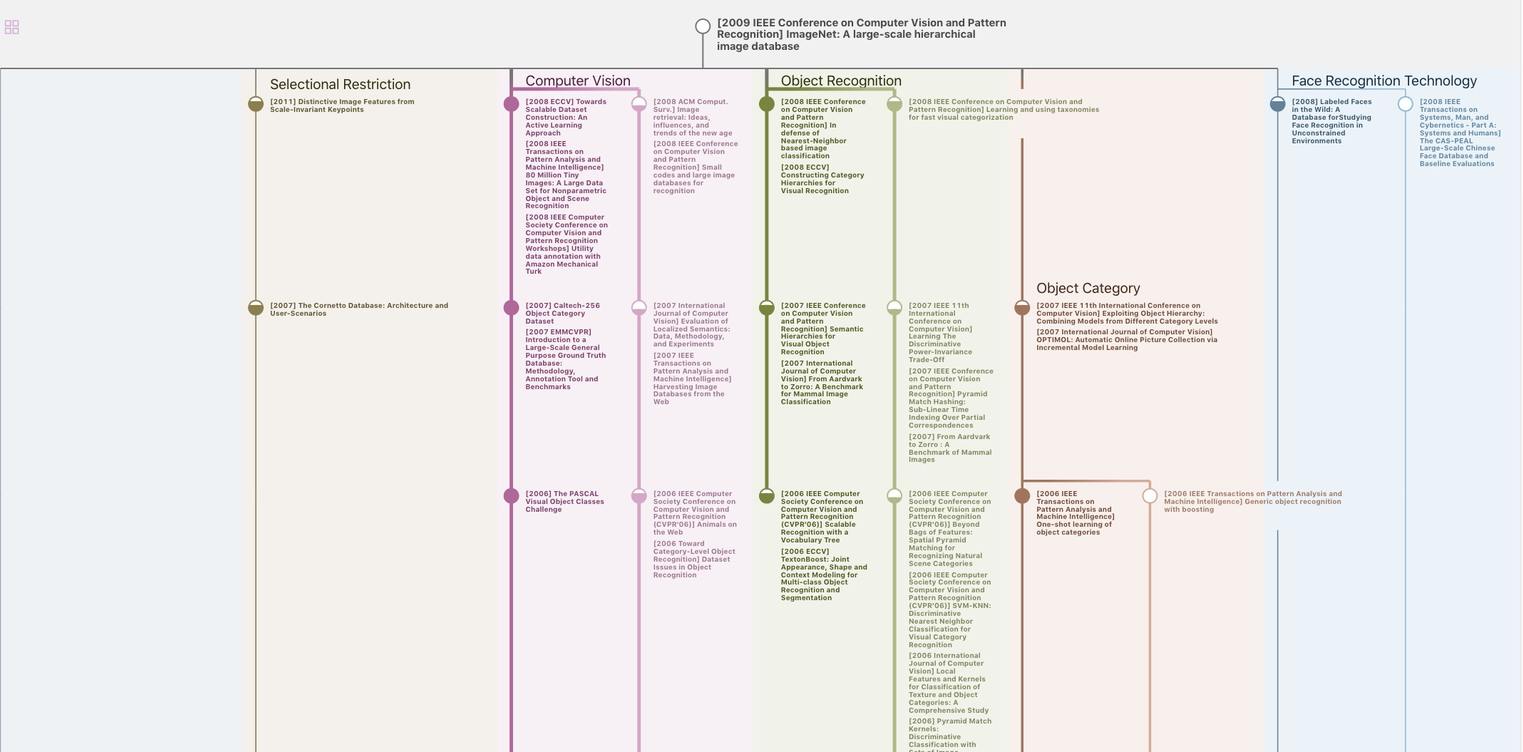
Generate MRT to find the research sequence of this paper
Chat Paper
Summary is being generated by the instructions you defined