Inferring the effects of protein variants on protein–protein interactions with an interpretable deep-learning model
Research Square (Research Square)(2022)
摘要
Abstract Identifying pathogenetic variants and inferring their impact on protein–protein interactions sheds light on their functional consequences on diseases. Limited by the availability of experimental data on the consequences on protein interaction, most existing methods focus on building models to predict changes in protein binding affinity. Here, we introduced MIPPI, an end-to-end, interpretable transformer-based deep learning model that learns features directly from sequences by leveraging the interaction data from IMEx. We trained MIPPI to determine the types of variant impact (increasing, decreasing, disrupting, and no effect) on protein-protein interactions, and demonstrated its accuracy and interpreted the learned attention weights, which correlate with the amino acids interacting with the variant. We further showed the utility of MIPPI in prioritizing de novo mutations associated with complex neurodevelopmental disorders and the potential in determining the pathogenic and driving mutations. Finally, we experimentally validated the functional impact of several variants identified in patients with such disorders. Overall, MIPPI is a versatile, robust, and interpretable model suitable for prediction tasks for mutation impact on protein–protein interaction and the discovery of clinically actionable variants. A webserver for MIPPI is available from https://www.psymukb.net/MIPPI.
更多查看译文
关键词
protein variants,protein interactions,deep-learning deep-learning,model
AI 理解论文
溯源树
样例
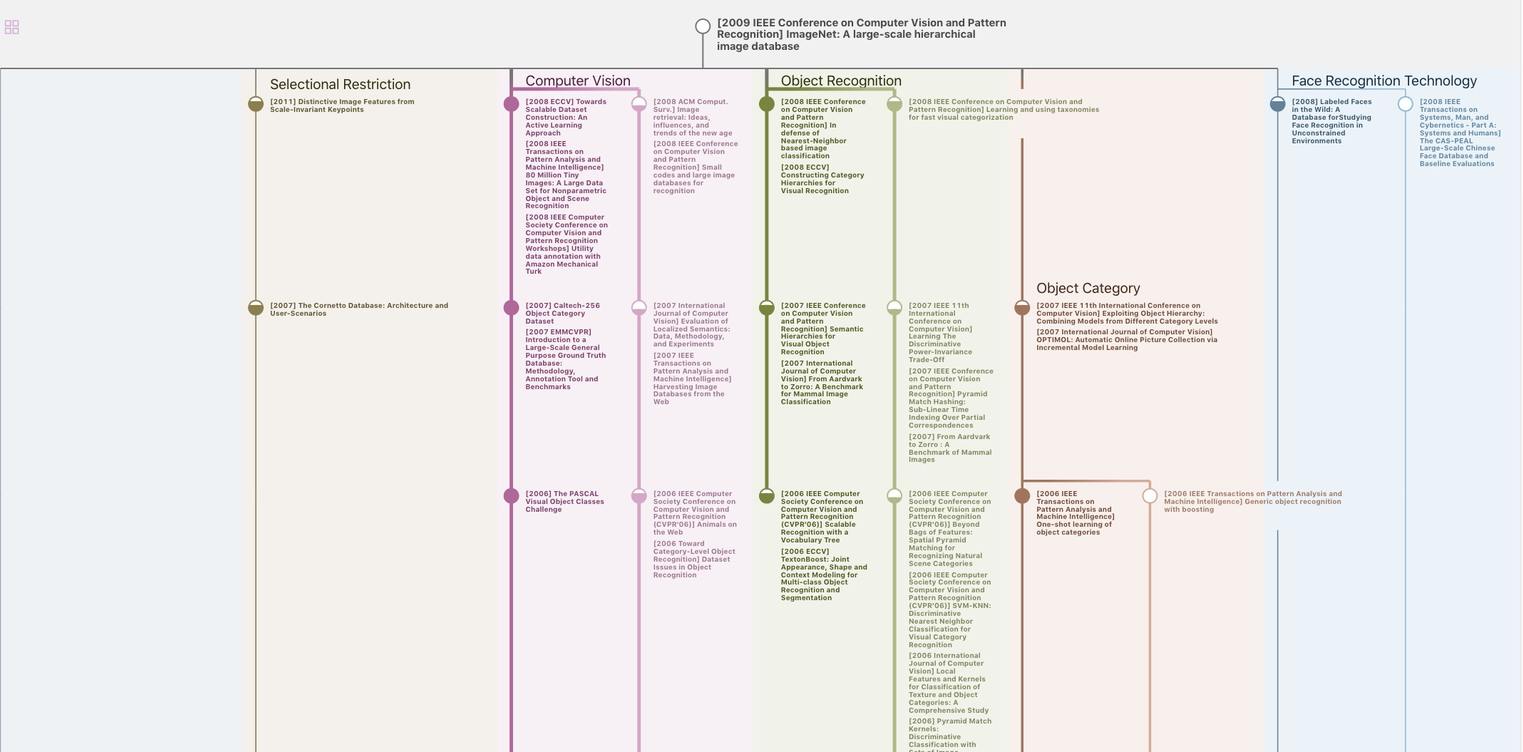
生成溯源树,研究论文发展脉络
Chat Paper
正在生成论文摘要