Accuratede novodetection of somatic mutations in high-throughput single-cell profiling data sets
bioRxiv (Cold Spring Harbor Laboratory)(2022)
摘要
AbstractCharacterization of somatic mutations at single-cell resolution is essential to study cancer evolution, clonal mosaicism, and cell plasticity. However, detection of mutations in single cells remains technically challenging. Here, we describe SComatic, an algorithm designed for the detection of somatic mutations in single-cell transcriptomic and ATAC-seq data sets without requiring matched bulk or single-cell DNA sequencing data. Using >1.5M single cells from 383 single-cell RNAseq and single-cell ATAC-seq data sets spanning cancer and non-neoplastic samples, we show that SComatic detects mutations in single cells, even in differentiated cells from polyclonal tissues not amenable to mutation detection using existing methods. In addition, SComatic permits the estimation of mutational burdens andde novomutational signature analysis at single-cell and cell-type resolution. Notably, using matched exome and single-cell RNAseq data, we show that SComatic achieves a 20 to 40-fold increase in precision as compared to existing algorithms for somatic SNV calling without compromising sensitivity. Overall, SComatic opens the possibility to study somatic mutagenesis at unprecedented scale and resolution using high-throughput single-cell profiling data sets.
更多查看译文
关键词
somatic mutations,high-throughput,single-cell
AI 理解论文
溯源树
样例
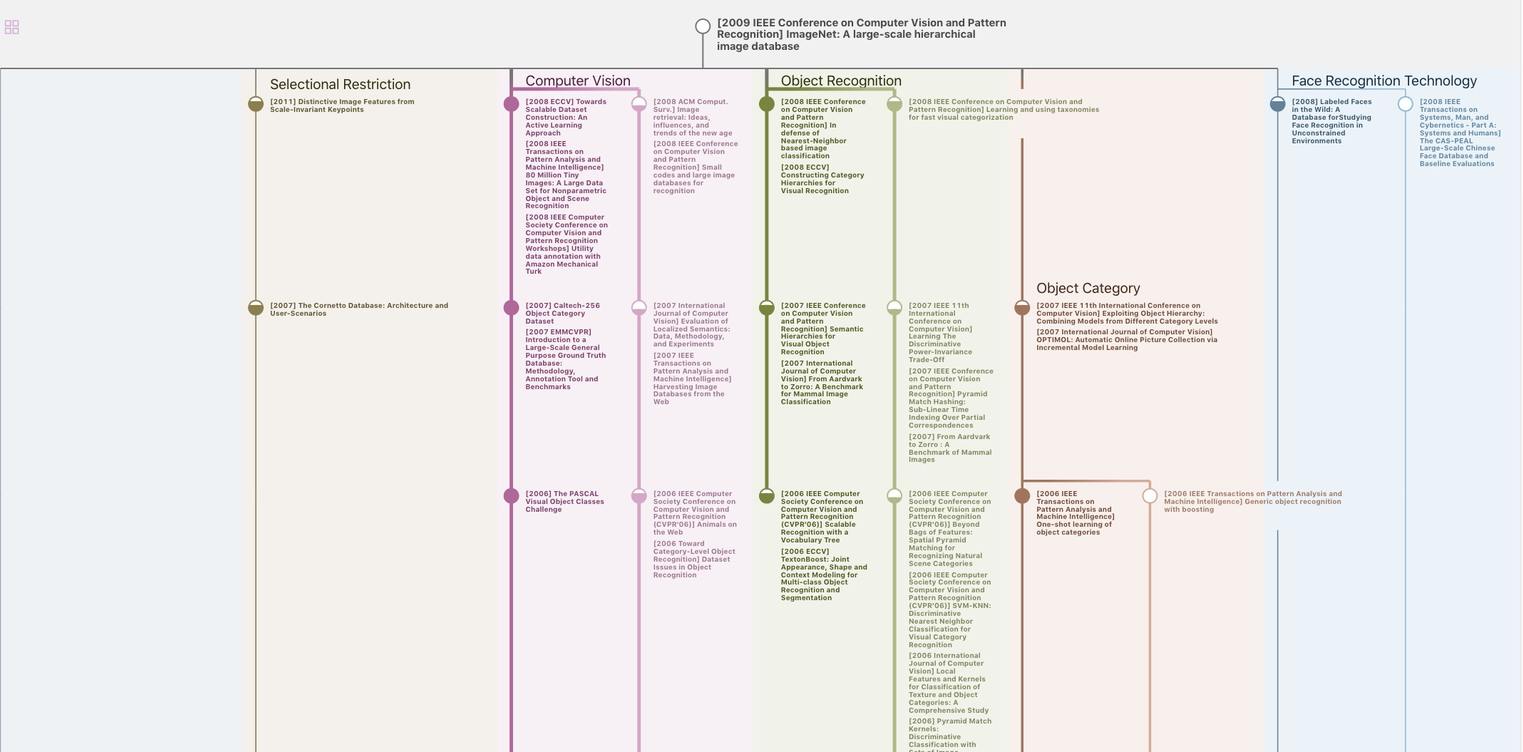
生成溯源树,研究论文发展脉络
Chat Paper
正在生成论文摘要