Modeling Adaptive Expression of Robot Learning Engagement and Exploring Its Effects on Human Teachers
ACM Transactions on Computer-Human Interaction(2023)
摘要
Robot Learning from Demonstration (RLfD) allows non-expert users to teach a robot new skills or tasks directly through demonstrations. Although modeled after human-human learning and teaching, existing RLfD methods make robots act as passive observers without the feedback of their learning statuses in the demonstration gathering stage. To facilitate a more transparent teaching process, we propose two mechanisms of Learning Engagement, Z2O-Mode and D2O-Mode, to dynamically adapt robots' attentional and behavioral engagement expressions to their actual learning status. Through an online user experiment with 48 participants, we find that, compared with two baselines, the two kinds of Learning Engagement can lead to users' more accurate mental models of the robot's learning progress, more positive perceptions of the robot, and better teaching experience. Finally, we provide implications for leveraging engagement expression to facilitate transparent human-AI (robot) communication based on our key findings.
更多查看译文
关键词
Human-robot interaction,learning from demonstration,transparent AI,robot teaching,robot engagement
AI 理解论文
溯源树
样例
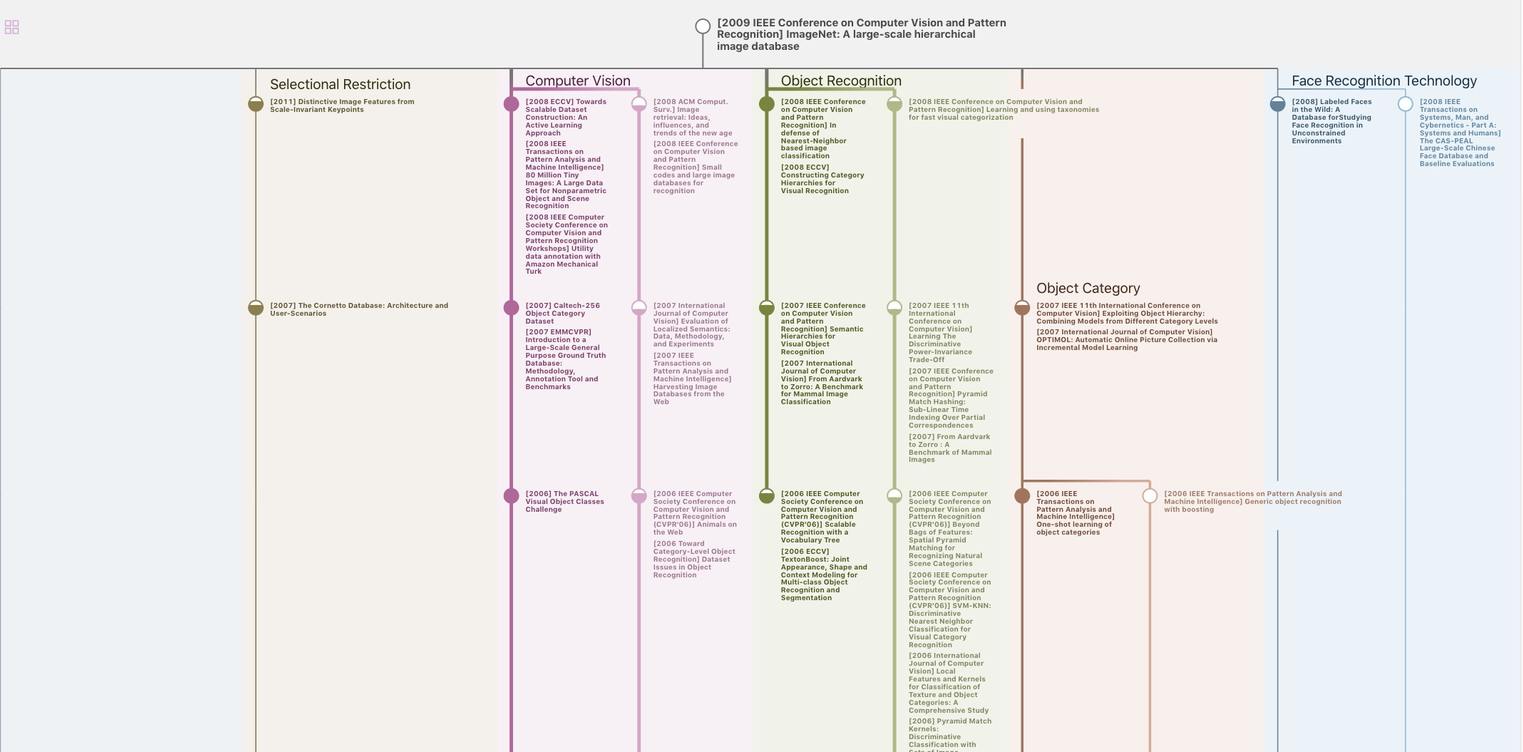
生成溯源树,研究论文发展脉络
Chat Paper
正在生成论文摘要