Power Quality Disturbance Detection Based on Improved Robust Random Cut Forest
2022 IEEE/IAS Industrial and Commercial Power System Asia (I&CPS Asia)(2022)
摘要
Many distribution network disturbances exhibit unique electrical signatures which can be observed from voltage and current waveforms. With the continuous enhancement of Power Quality Data (PQD) acquisition capabilities, it is feasible to continuously monitor the operating status of power nodes from a more detailed perspective. In the past decade, an increasing amount of high-quality PQD has been collected accumulating a massive and unique high-resolution power grid data asset. However, how to obtain valuable information, such as Power Quality Disturbance Events (PQDEs) from massive PQD remains challenging in the research community. In this paper, a reliable PQDEs detection method is proposed based on the improved Robust Random Cut Forest (RRCF). This method achieves accurate detection of PQDEs through the adaptive improvement of pre-filtering based on Ensemble Empirical Mode Decomposition (EEMD) and redundant interpolation. Numerical test results on the synthetic PQD and the realistic pollution experiment of a silicone rubber insulator in a salt fog chamber demonstrate the reliability, efficiency, and scalability of the proposed approach in practical online detection.
更多查看译文
关键词
power quality, Robust Random Cut Forest (RRCF), abnormality detection, big data, arcing
AI 理解论文
溯源树
样例
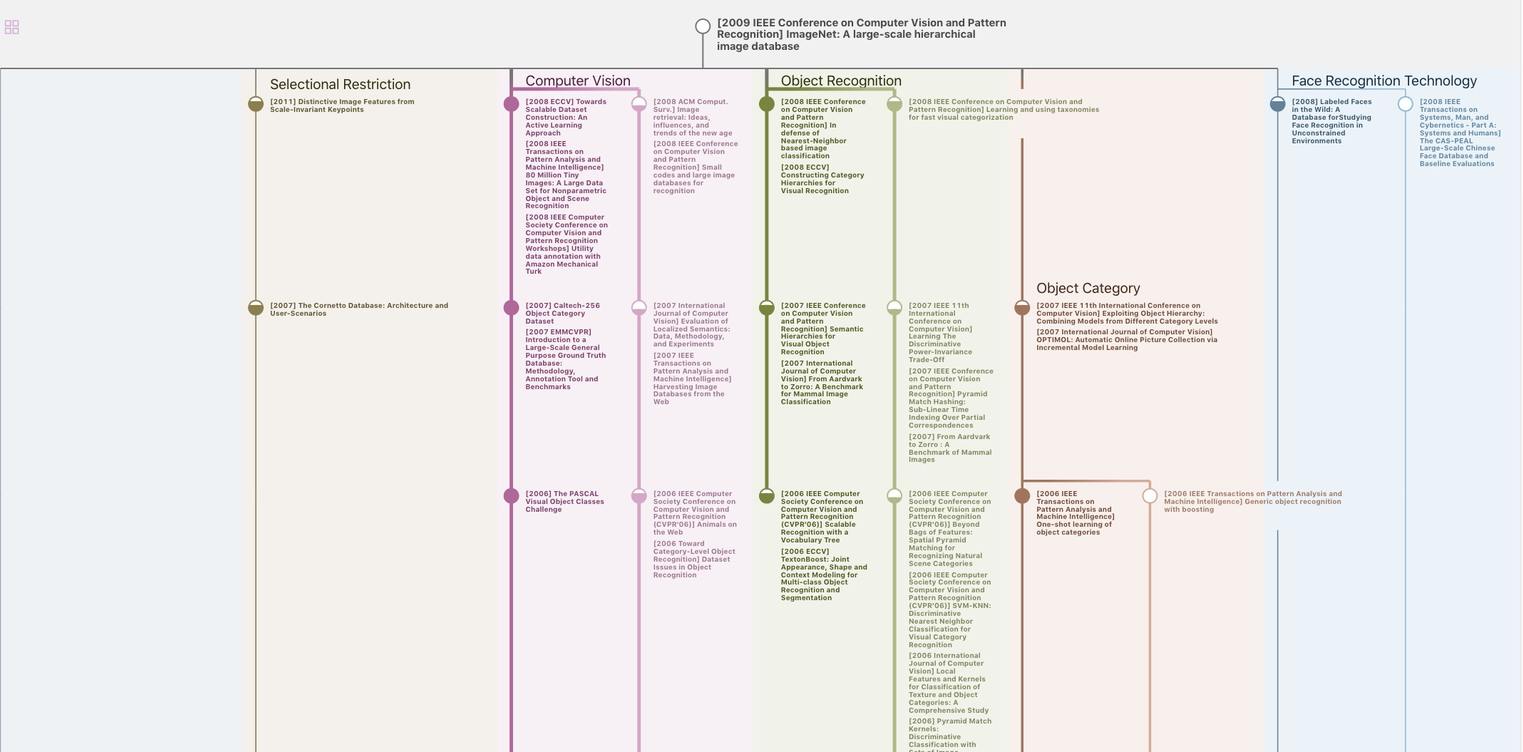
生成溯源树,研究论文发展脉络
Chat Paper
正在生成论文摘要