Probabilistic Machine Learning to Improve Generalisation of Data-Driven Turbulence Modelling
SSRN Electronic Journal(2023)
摘要
A probabilistic machine learning model is introduced to augment the $k-\omega\ SST$ turbulence model in order to improve the modelling of separated flows and the generalisability of learnt corrections. Increasingly, machine learning methods have been used to leverage experimental and high-fidelity data, improving the accuracy of the Reynolds Averaged Navier Stokes (RANS) turbulence models widely used in industry. A significant challenge for such methods is their ability to generalise to unseen geometries and flow conditions. Furthermore, heterogeneous datasets containing a mix of experimental and simulation data must be efficiently handled. In this work, field inversion and an ensemble of Gaussian Process Emulators (GPEs) is employed to address both of these challenges. The ensemble model is applied to a range of benchmark test cases, demonstrating improved turbulence modelling for cases with separated flows with adverse pressure gradients, where RANS simulations are understood to be unreliable. Perhaps more significantly, the simulation reverted to the uncorrected model in regions of the flow exhibiting physics outside of the training data.
更多查看译文
关键词
probabilistic machine learning,machine learning,modelling,generalisation,data-driven
AI 理解论文
溯源树
样例
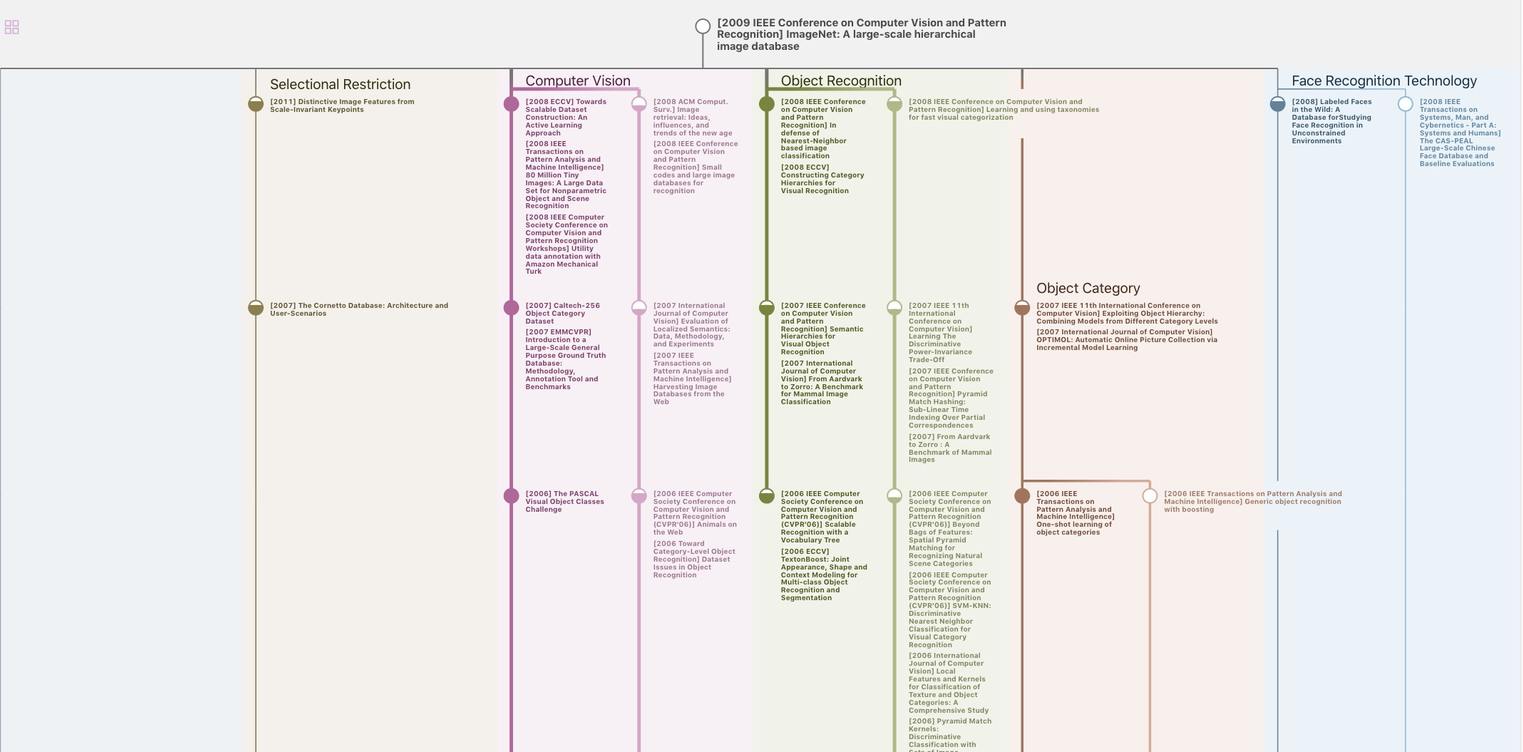
生成溯源树,研究论文发展脉络
Chat Paper
正在生成论文摘要