UCL: Unsupervised Curriculum Learning for Utility Pole Detection from Aerial Imagery
2022 International Conference on Digital Image Computing Techniques and Applications (DICTA)(2022)
摘要
This paper introduces a machine learning-based approach for detecting electric poles, an essential part of power grid maintenance.With the increasing popularity of deep learning, several such approaches have been proposed for electric pole detection.However, most of these approaches are supervised, requiring a large amount of labeled data, which is time-consuming and labor-intensive.Unsupervised deep learning approaches have the potential to overcome the need for huge amounts of training data.This paper presents an unsupervised deep learning framework for utility pole detection.The framework combines Convolutional Neural Network (CNN) and clustering algorithms with a selection operation.The CNN architecture for extracting meaningful features from aerial imagery, a clustering algorithm for generating pseudo labels for the resulting features, and a selection operation to filter out reliable samples to fine-tune the CNN architecture further.The fine-tuned version then replaces the initial CNN model, thus improving the framework, and we iteratively repeat this process so that the model learns the prominent patterns in the data progressively.The presented framework is trained and tested on a small dataset of utility poles provided by "Mention Fuvex" (a Spanish company utilizing long-range drones for power line inspection).Our extensive experimentation demonstrates the progressive learning behavior of the proposed method and results in promising classification scores with significance test having p -value < 0.00005 on the utility pole dataset.
更多查看译文
AI 理解论文
溯源树
样例
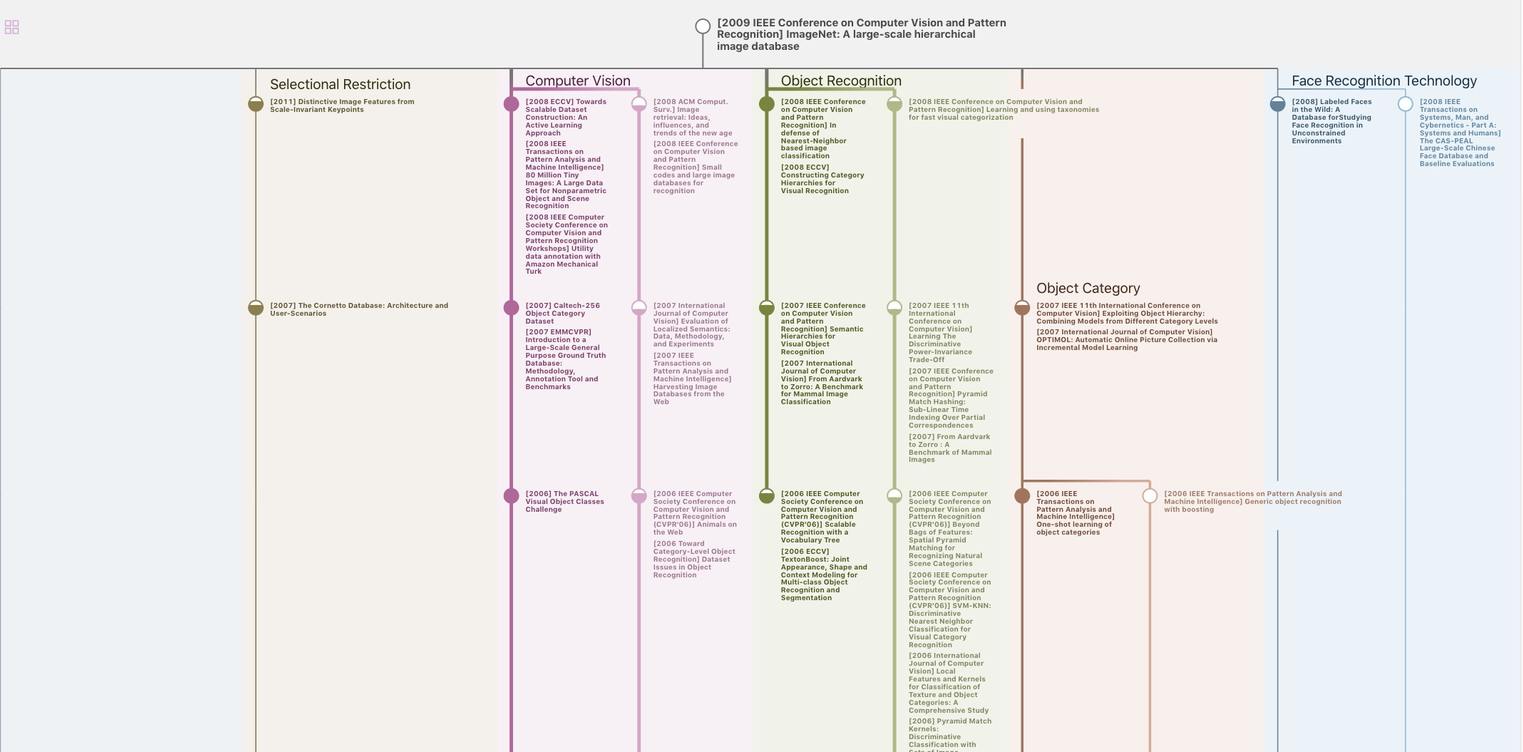
生成溯源树,研究论文发展脉络
Chat Paper
正在生成论文摘要