On Robot Grasp Learning Using Equivariant Models
crossref(2023)
摘要
Abstract Real-world grasp detection is challenging due to the stochasticity in grasp dynamics and the noise in hardware. The desideratum is to adapt real world through end-to-end learning in the real world. However, in this case, end-to-end learning relies on a large amount of expensive robot data samples. In this paper, we investigate the planar grasp function is $\SE(2)$-equivariant and encodes such induced bias using an equivariant convolutional neural network. This induced bias significantly improves sample efficiency of grasp learning and enables end-to-end training from scratch on a physical robot with only $600$ grasp attempts. We term our method Sample Efficient Grasp learning as SEG. On robot grasp learning experiments show after only 1.5 hours, SEG could learn a good grasp function on either depth modality for opaque or RGB modality for transparent object grasping.
更多查看译文
AI 理解论文
溯源树
样例
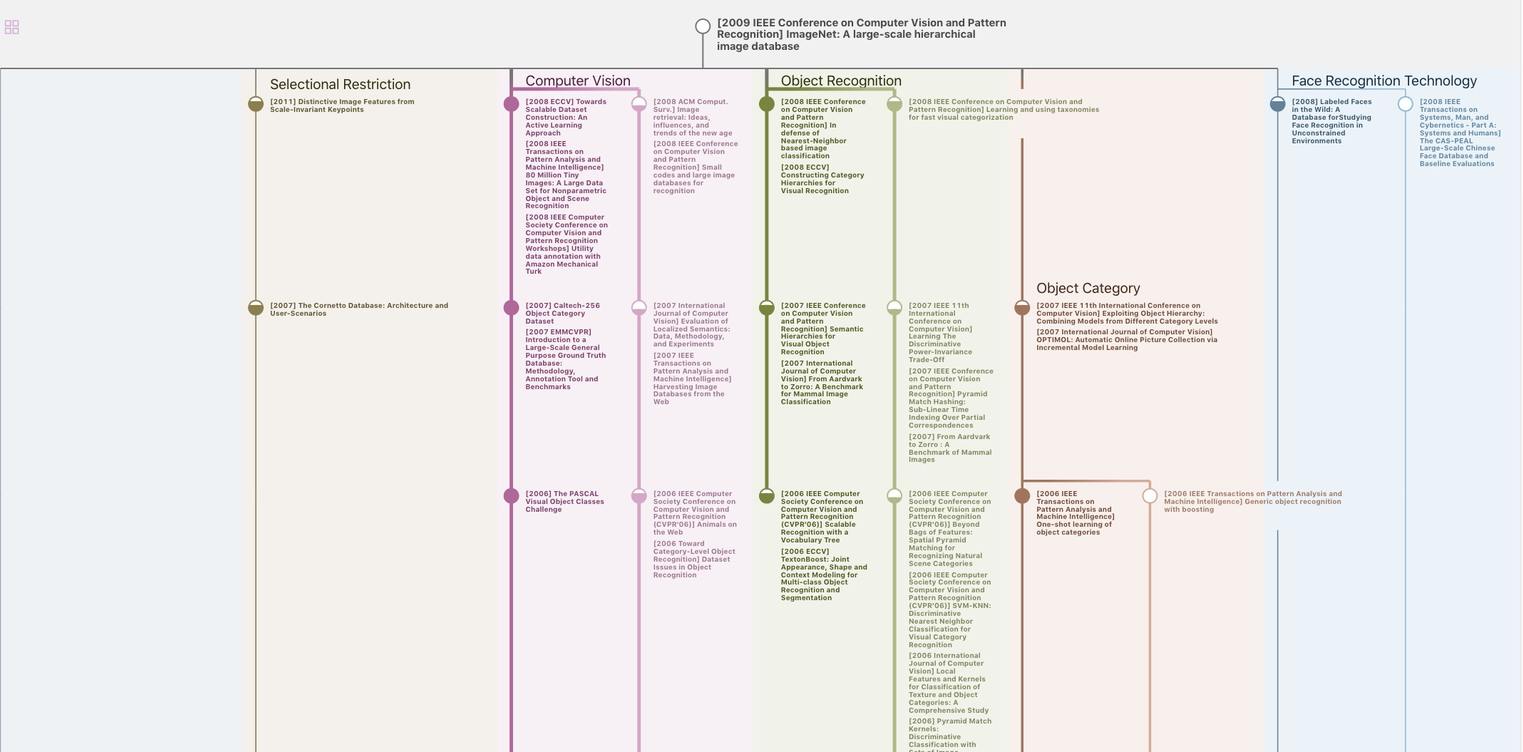
生成溯源树,研究论文发展脉络
Chat Paper
正在生成论文摘要