Scalable Kernel $K$-Means with Randomized Sketching: from Theory to Algorithm
IEEE transactions on knowledge and data engineering(2023)
Abstract
Kernel $k$ -means is a fundamental unsupervised learning in data mining. Its computational requirements are typically at least quadratic in the number of data, which are prohibitive for large-scale scenarios. To address these issues, we propose a novel randomized sketching approach SKK based on the circulant matrix. SKK projects the kernel matrix left and right according to the proposed sketch matrices to obtain a smaller one and accelerates the matrix-matrix product by the fast Fourier transform based on the circulant matrix, which can greatly reduce the computational requirements of the approximate kernel $k$ -means estimator with the same generalization bound as the exact kernel $k$ -means in the statistical setting. In particular, theoretical analysis shows that taking the sketch dimension of $\sqrt{n}$ is sufficient for SKK to achieve the optimal excess risk bound with only a fraction of computations, where $n$ is the number of data. The extensive experiments verify our theoretical analysis, and SKK achieves the state-of-the-art performances on 12 real-world datasets. To the best of our knowledge, in randomized sketching, this is the first time that unsupervised learning makes such a significant breakthrough.
MoreTranslated text
Key words
Kernel k-means,randomized sketching,statistical and computational trade-offs,excess risk bound
AI Read Science
Must-Reading Tree
Example
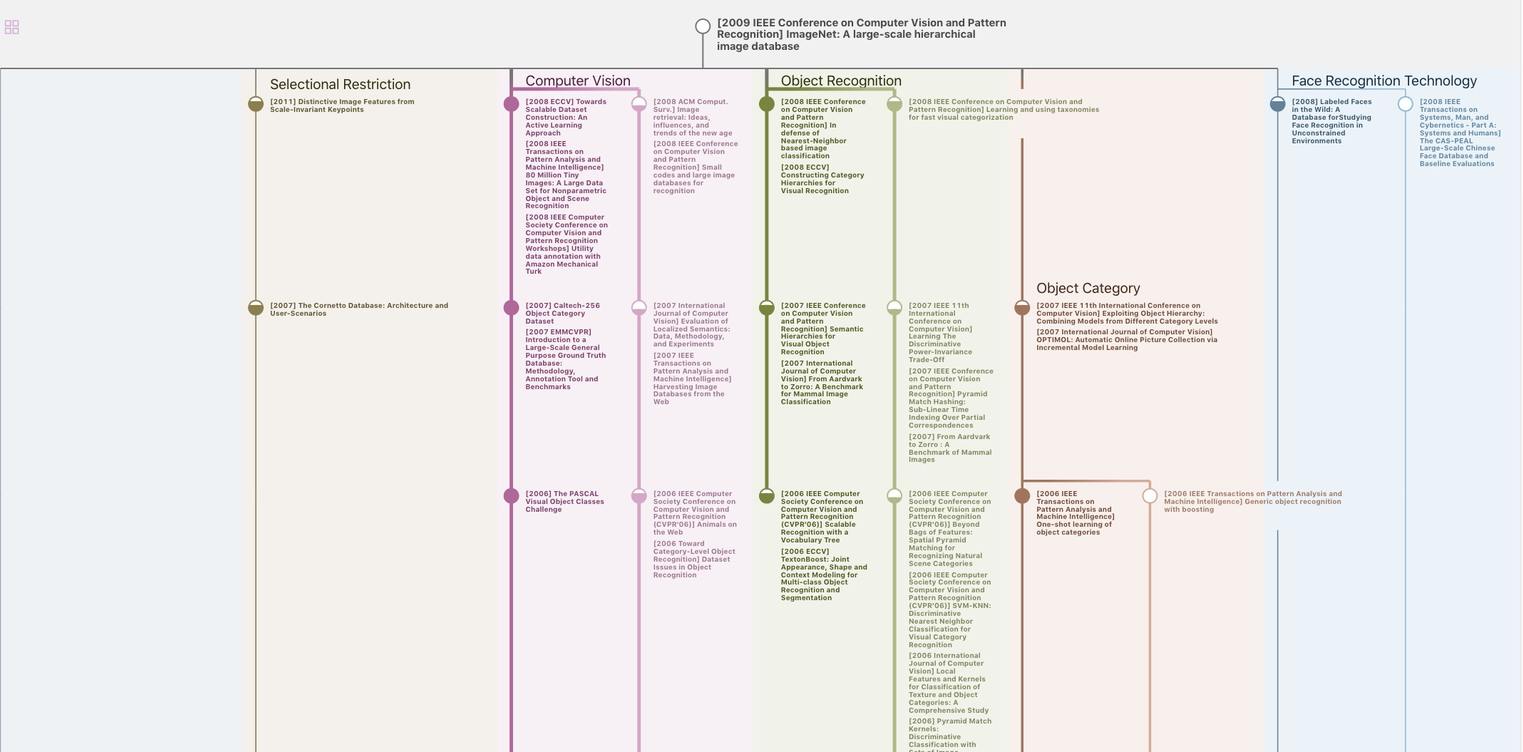
Generate MRT to find the research sequence of this paper
Chat Paper
Summary is being generated by the instructions you defined