Region Purity-Based Local Feature Selection: A Multiobjective Perspective
IEEE Transactions on Evolutionary Computation(2023)
摘要
In contrast to the traditional feature selection (FS), local FS (LFS) partitions the whole sample space and obtains the feature subset for each local region. However, most existing LFS algorithms lack a problem-specific objective function and instead simply apply the distance-like objective function, which limits their classification performance. In addition, obtaining a good LFS model is essentially a multiobjective optimization problem. Therefore, in this article, we propose a region purity (RP)-based LFS (RP-LFS) where, besides the proportion of the selected features and region-based distance metric, we design a novel objective function, RP, from the perspective of combining local features with classifiers. To solve the RP-LFS, an improved nondominated sorting genetic algorithm III is proposed. Specifically, a network-inspired crossover operator and a quick bit mutation are applied, which can improve the ability to search for better solutions. A regional feature sharing strategy between different local models is developed, which can preserve more effective features. Experimental studies on 11 UCI datasets and nine high-dimensional datasets validate the effectiveness of our proposed RP. In comparison with various state-of-the-art FS and LFS algorithms, RP-LFS can achieve very competitive classification accuracy while obtaining a reduced feature subset size.
更多查看译文
关键词
Local feature selection (LFS),multiobjective optimization,region purity (RP),regional feature sharing strategy (RFSS)
AI 理解论文
溯源树
样例
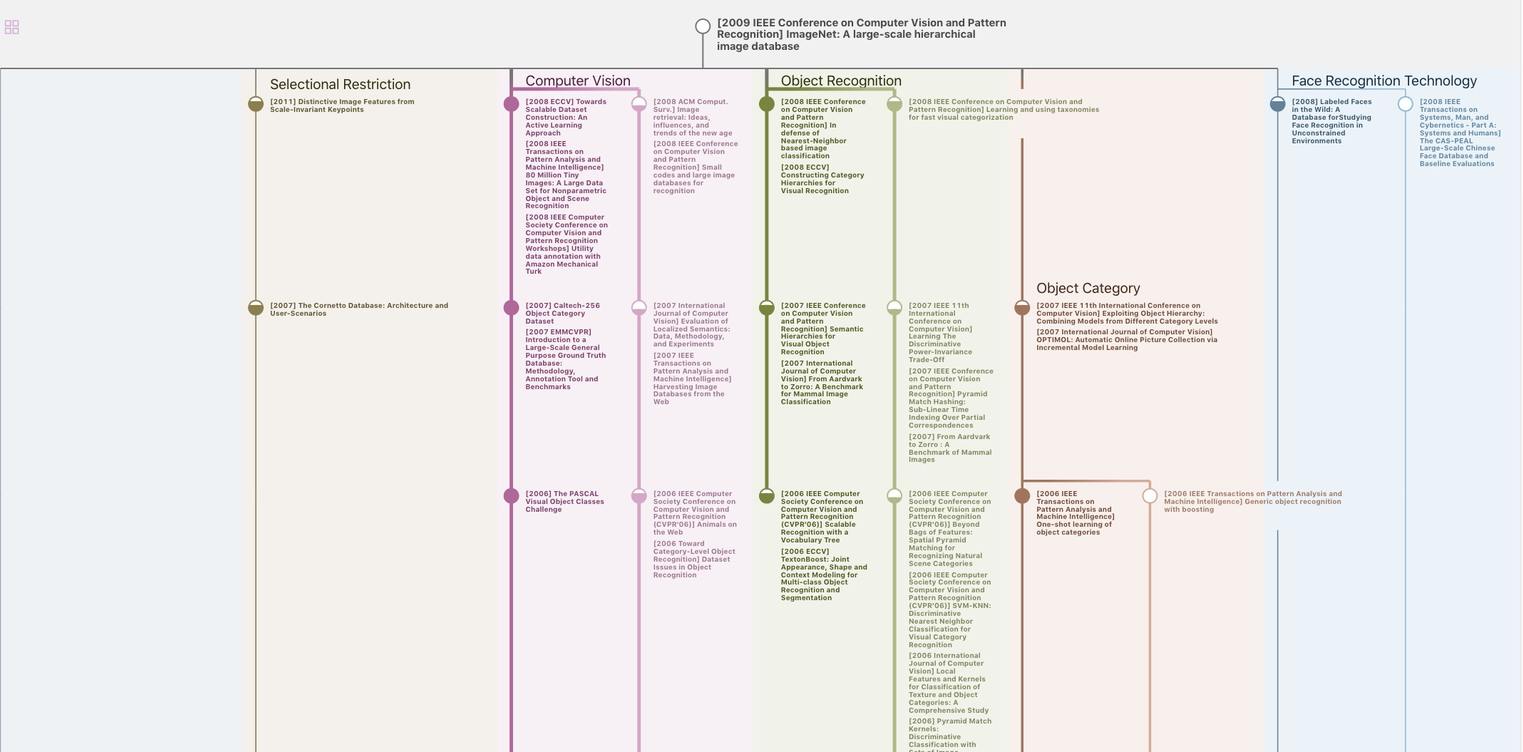
生成溯源树,研究论文发展脉络
Chat Paper
正在生成论文摘要