Inference of fixed bias probe measurements - A machine learning approach
Research Square (Research Square)(2022)
摘要
Abstract A new approach is presented to improve inferences made with Langmuir probe measurements in plasma while providing estimates of uncertainties. The technique makes use of a combination of computer simulations, analytic approximations, and multivariate regressions. It involves training inference models using currents collected by two probes, biased at different fixed potentials relative to a satellite. An advantage with fixed bias probes is their higher temporal and spatial resolution, compared to the more standard mode operation where bias voltages are swept in time. Synthetic data used to train models are constructed from kinetic simulations with assumed satellite and plasma parameters relevant to satellites in Low Earth Orbit (LEO). Simulations are made assuming a truncated Swarm geometry, the two Electric Field Instruments (EFI) Langmuir probes, and different satellite potentials in the range [-3, 0] V. Models are constructed using two approaches, with two-tuples of currents as independent variables. Inference skills are assessed with different metrics, from comparisons between inferred plasma and satellite parameters, and known values used as input in the simulations. The models are then applied to infer the same physical parameters, using Swarm A in situ measured currents, and comparing them with values reported on the Swarm data portal. Inferences are consistent with values reported on the Swarm data portal, although it is not possible at this point to determine if, and if so, to what extent, the simulation-regression approach leads to better inferences.
更多查看译文
关键词
bias probe measurements,machine learning,machine learning approach
AI 理解论文
溯源树
样例
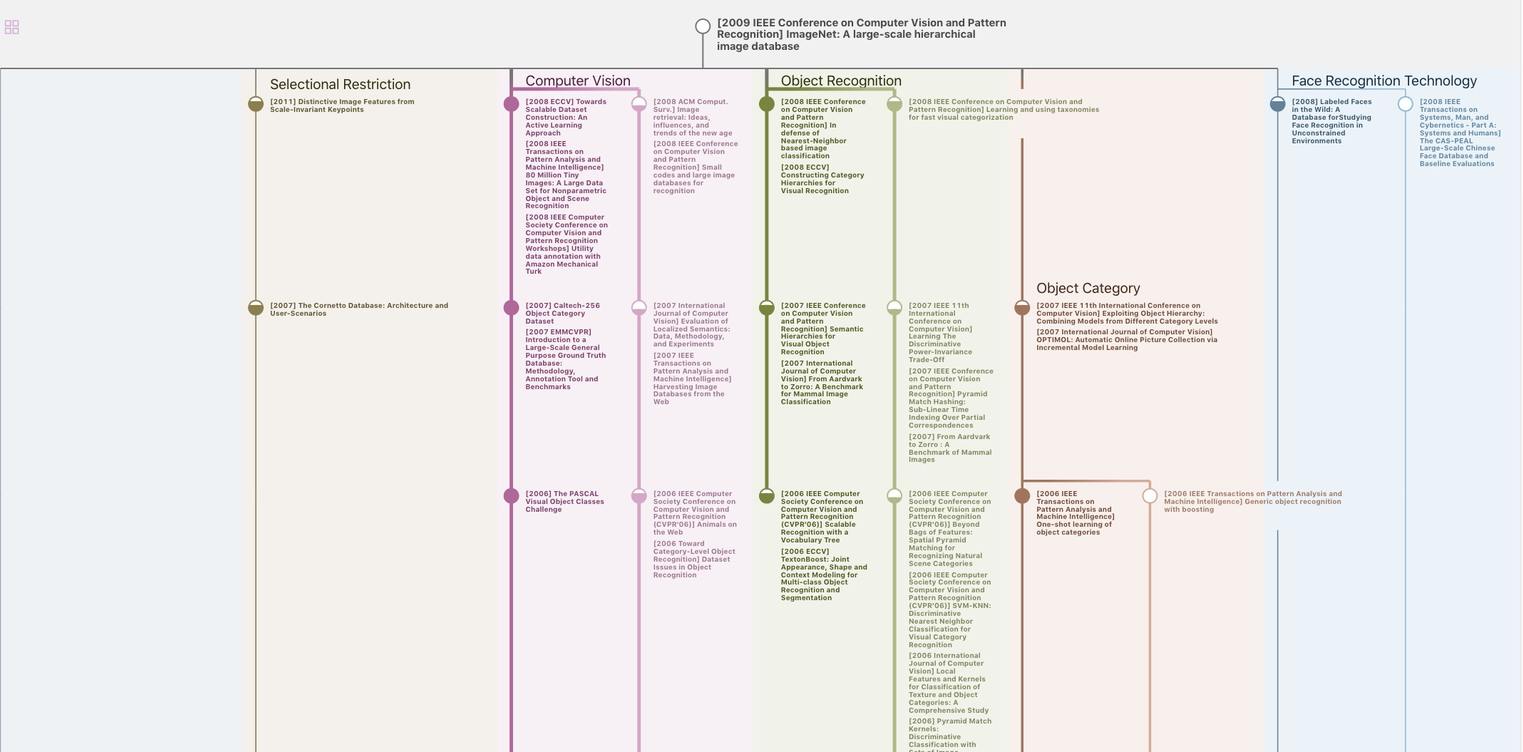
生成溯源树,研究论文发展脉络
Chat Paper
正在生成论文摘要