A Comparative Analysis of Apriori and FP-Growth Algorithms for Market Basket Analysis Using Multi-level Association Rule Mining
Lecture Notes in Management and Industrial EngineeringIndustrial Engineering in the Covid-19 Era(2023)
摘要
Nowadays, many companies have massive amounts of data. Data mining is essential to gain business insights from large amounts of data. Market basket analysis is a data mining technique that analyzes customer buying behavior by discovering relationships between pairs of products purchased together. This analysis helps companies to design a better strategy for business decisions such as marketing and campaign management, store layout optimization, and inventory control. FP-Growth and Apriori are two widely used algorithms for market basket analysis. In this study, Apriori and FP-Growth algorithms are applied for market basket analysis with real-life data from an FMCG retailer. Furthermore, the performance of these algorithms is compared using multilevel association rules mining. Numerical results showed that the FP-Growth has greater performance than Apriori in terms of run time and memory for all levels of product groups. On the other hand, compared to FP-Growth, Apriori is better in terms of generating good candidates. At this point, a hybrid approach can be developed that minimizes the disadvantages of both algorithms.
更多查看译文
关键词
market basket analysis,apriori,association,fp-growth,multi-level
AI 理解论文
溯源树
样例
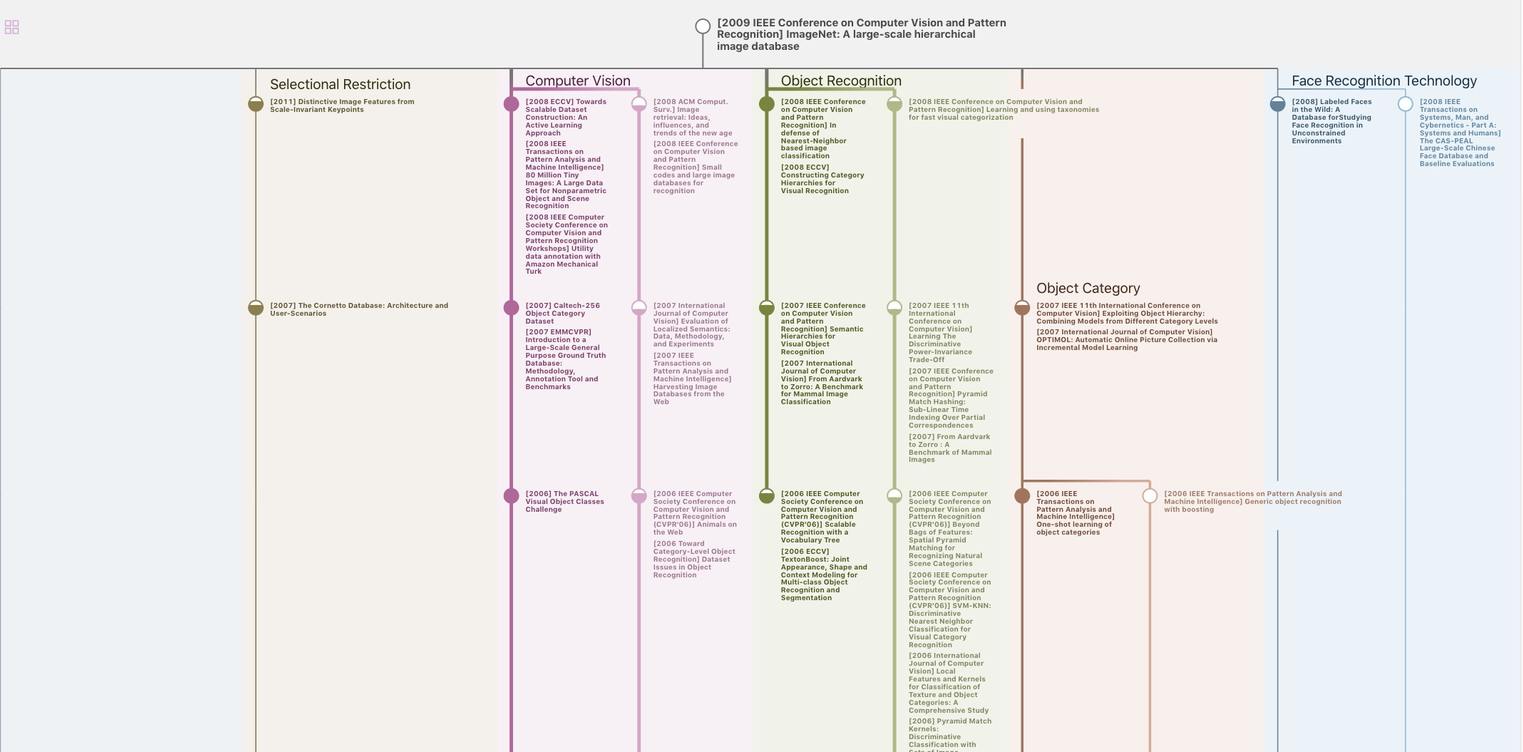
生成溯源树,研究论文发展脉络
Chat Paper
正在生成论文摘要