Enhancing the reliability and accuracy of AI-enabled diagnosis via complementarity-driven deferral to clinicians (CoDoC)
Research Square (Research Square)(2022)
摘要
Abstract Diagnostic AI systems trained using deep learning have been shown to achieve expert-level identification of diseases in multiple medical imaging settings1,2. However, such systems are not always reliable and can fail in cases diagnosed accurately by clinicians and vice versa3. Mechanisms for leveraging this complementarity by learning to select optimally between discordant decisions of AIs and clinicians have remained largely unexplored in healthcare4, yet have the potential to achieve levels of performance that exceed that possible from either AI or clinician alone4. We develop a Complementarity-driven Deferral-to-Clinical Workflow (CoDoC) system that can learn to decide when to rely on a diagnostic AI model and when to defer to a clinician or their workflow. We show that our system is compatible with diagnostic AI models from multiple manufacturers, obtaining enhanced accuracy (sensitivity and/or specificity) relative to clinician-only or AI-only baselines in clinical workflows that screen for breast cancer or tuberculosis. For breast cancer, we demonstrate the first system that exceeds the accuracy of double-reading with arbitration (the “gold standard” of care) in a large representative UK screening program, with 25% reduction in false positives despite equivalent true-positive detection, while achieving a 66% reduction in clinical workload. In two separate US datasets, CoDoC exceeds the accuracy of single-reading by board certified radiologists and two different standalone state-of-the-art AI systems, with generalisation of this finding in different diagnostic AI manufacturers. For TB screening with chest X-rays, CoDoC improved specificity (while maintaining sensitivity) compared to standalone AI or clinicians for 3 of 5 commercially available diagnostic AI systems (5–15% reduction in false positives). Further, we show the limits of confidence score based deferral systems for medical AI, by demonstrating that no deferral strategy could have achieved significant improvement on the remaining two diagnostic AI systems. Our comprehensive assessment demonstrates that the superiority of CoDoC is sustained in multiple realistic stress tests for generalisation of medical AI tools along four axes: variation in the medical imaging modality; variation in clinical settings and human experts; different clinical deferral pathways within a given modality; and different AI softwares. Further, given the simplicity of CoDoC we believe that practitioners can easily adapt it and we provide an open-source implementation to encourage widespread further research and application.
更多查看译文
关键词
diagnosis,clinicians,deferral,ai-enabled,complementarity-driven
AI 理解论文
溯源树
样例
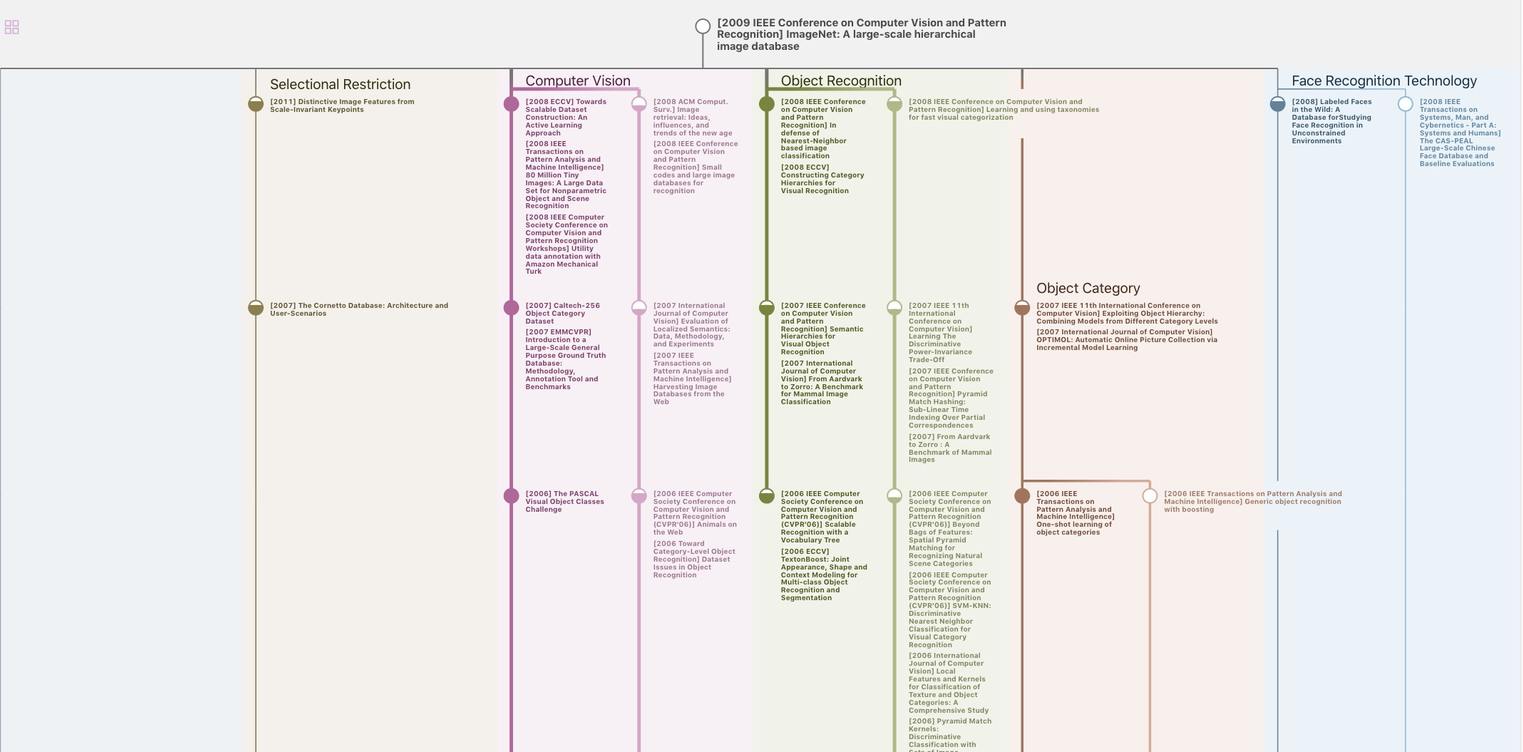
生成溯源树,研究论文发展脉络
Chat Paper
正在生成论文摘要