Selective Kernel Prototypical Network for Few-shot Motor Fault Diagnosis with Unseen Faults
2022 Global Reliability and Prognostics and Health Management (PHM-Yantai)(2022)
摘要
Motor fault diagnosis has been paid increasing attention in the academia and industry. Data-driven methods have achieved great success with massive experimental data to train the model. In industrial scenes, data sparsity is a nonnegligible problem restricting the application of these methods. Not enough data can be accessible in faulty conditions and very limited data may be obtained for some less frequently occurring faults. To tackle this few-shot learning problem, a prototypical network based on selective kernel network is proposed in this paper. One-dimensional selective kernel network is used to enhance the embedding learning ability of prototypical network based on its dynamic convolutional kernel selection mechanism. The effectiveness and superiority of the proposed method are verified on motor experimental data. Results show that the proposed method can achieve high diagnosis accuracy on unseen faults which have very few samples.
更多查看译文
关键词
motor,few-shot learning,fault diagnosis,prototypical network,selective kernel
AI 理解论文
溯源树
样例
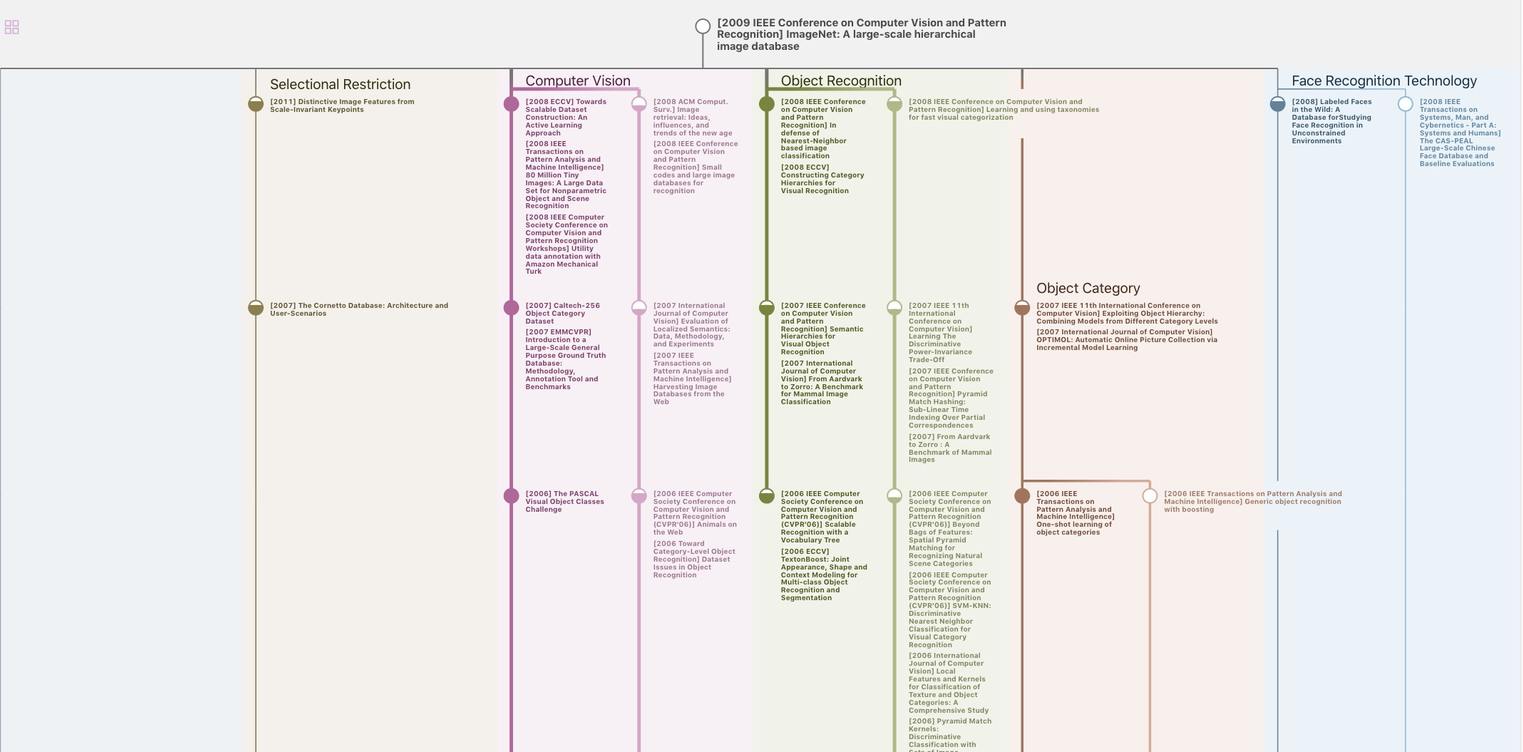
生成溯源树,研究论文发展脉络
Chat Paper
正在生成论文摘要