Assessing the Quality of Differentially Private Synthetic Data for Intrusion Detection
Lecture Notes of the Institute for Computer Sciences, Social Informatics and Telecommunications EngineeringSecurity and Privacy in Communication Networks(2023)
摘要
Supervised learning is effectively adopted in Network Intrusion Detection Systems (IDS) to detect malicious activities in Information Technology (IT) and Operation Technology (OT). Sharing high-quality network data among cyber-security practitioners increases the chance of detecting new threat campaigns by analyzing updated traffic features. As data sharing brings privacy concerns, Differential-Privacy (DP) has emerged as a promising approach to performing privacy-preserving analytics. This paper presents an approach to generating high-quality synthetic network features using a differentially private Generative Adversarial Network (DP-GAN) based on the DoppleGANger https://github.com/fjxmlzn/DoppelGANger toolset. We assess the classification performance of several machine learning (ML) models on a privacy-preserved synthetic dataset derived from the NSL-KDD intrusion dataset. Experiments show ML algorithms achieve high classification accuracy on the synthetic data ( $$95.95\%$$ ) with a low privacy budget ( $$\varepsilon = 6.73$$ ), i.e., low success rates for membership inference attacks. Hence, DP-GAN models offer a promising tool for sharing traffic features with bounded loss of privacy.
更多查看译文
关键词
private synthetic data
AI 理解论文
溯源树
样例
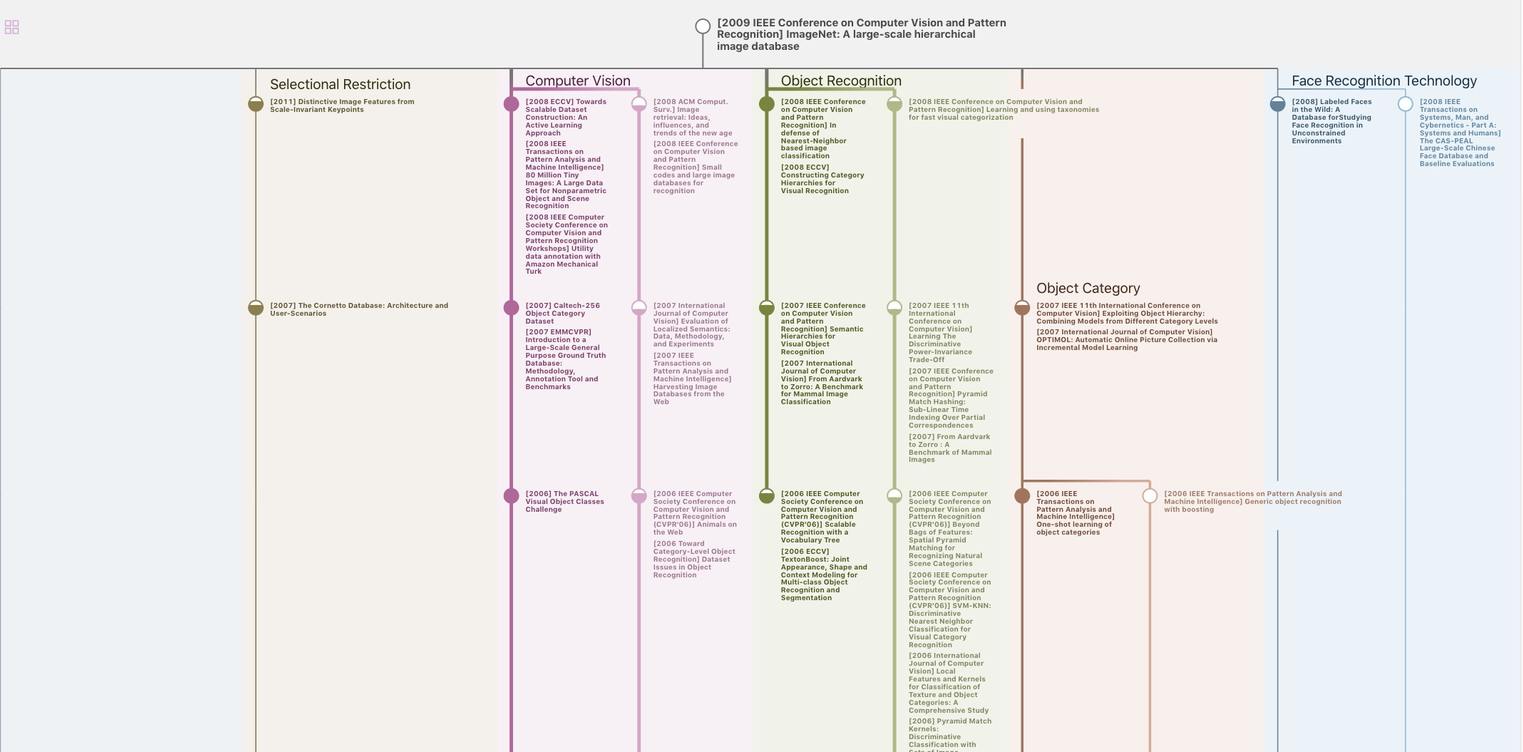
生成溯源树,研究论文发展脉络
Chat Paper
正在生成论文摘要