Edge Intelligence for Real-Time IoT Service Trust Prediction
IEEE Transactions on Services Computing(2023)
摘要
Mobile Edge Computing (MEC)-based Internet of Things (IoT) systems generate trust information in a real-time and distributed manner. Predicting trustworthiness of IoT services in such an MEC environment requires new prediction strategies that cater for the aforementioned characteristics of trust information. More importantly, it is imperative to investigate how the real-time trust information could be effectively integrated into trust prediction strategies in order to capture the ever-evolving nature of trustworthiness of IoT services. In turn, such a strategy allows IoT service consumers to derive more relevant and accurate trust-based decisions. To that end, our work models trust prediction in MEC-based IoT systems as an online regularized finite-sum problem in a distributed MEC environment with a given MEC topology. We then adopt the Online Alternating Direction Method (OADM) to effectively train trust prediction models in parallel over the distributed MEC environment. OADM allows splitting the aforementioned finite-sum problem into multiple sub-problems that correspond to different local MEC environments. These sub-problems can then be solved iteratively within each local MEC environment by using the local trust data therein. This can avoid the movement of data across the core networks of mobile network providers. Experiments on real-world and synthetic datasets demonstrate the effectiveness and scalability of the proposed method.
更多查看译文
关键词
Trust,Internet of Things,mobile edge computing,machine learning,online learning
AI 理解论文
溯源树
样例
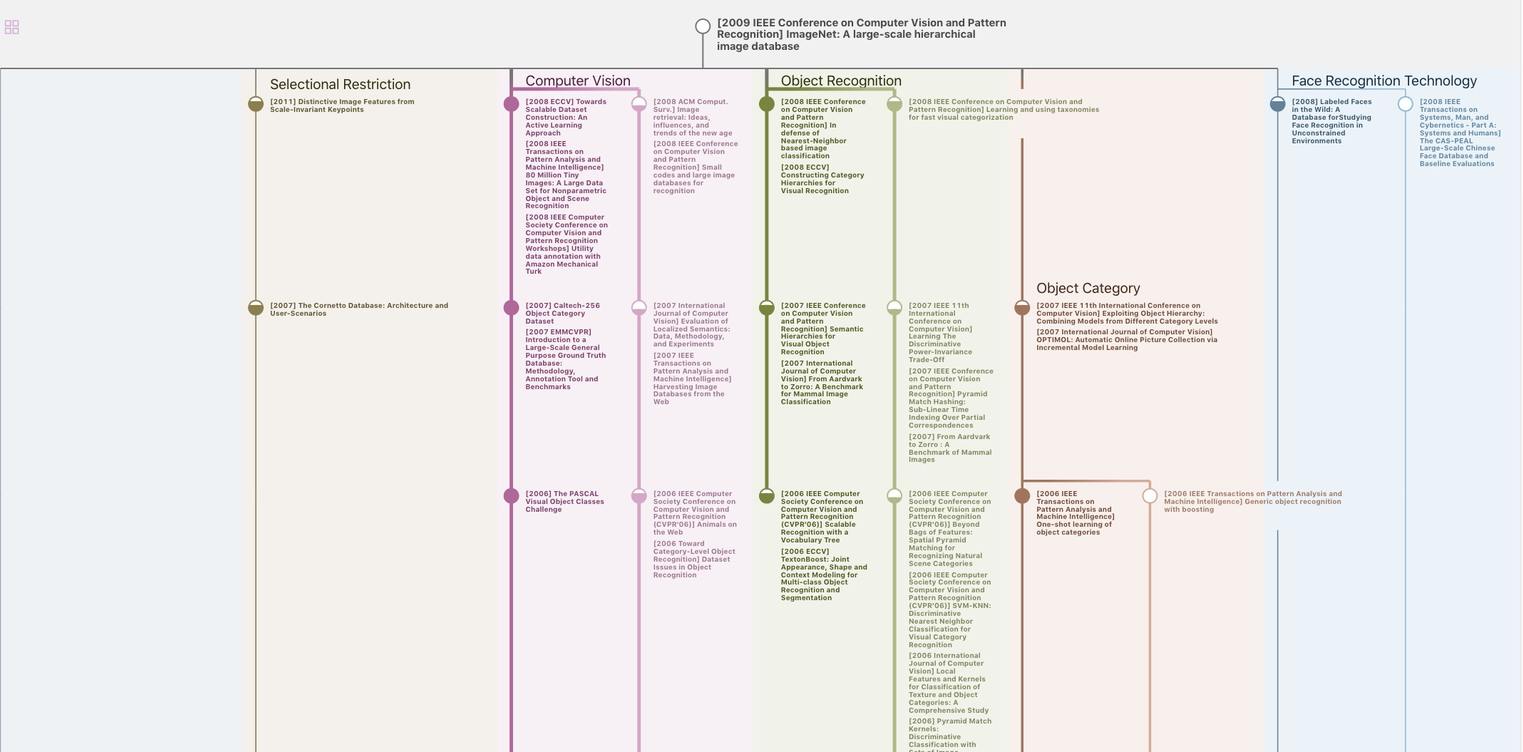
生成溯源树,研究论文发展脉络
Chat Paper
正在生成论文摘要