Discovery of Customized Dispatching Rule for Single-Machine Production Scheduling Using Deep Reinforcement Learning
PROCEEDINGS OF ASME 2022 INTERNATIONAL DESIGN ENGINEERING TECHNICAL CONFERENCES AND COMPUTERS AND INFORMATION IN ENGINEERING CONFERENCE, IDETC-CIE2022, VOL 2(2022)
摘要
A dispatching rule has become one of the most widely used approaches in producing scheduling due to its low time complexities and the ability to respond to dynamic changes in production. However, there is no one dispatching rule that dominates the others for the performance measure of interest. By modelling the selection of a dispatching rule to transit from one production state to another using a Markov decision process, current methods involving reinforcement learning make use of a predefined list of dispatching rules, which may limit the optimization of a specified performance measure. Greater flexibility can be achieved by creating customized dispatching rules through the important selection of production parameters for the performance measure in question. Using parameters obtained readily within the digital twin setting, this paper investigates the application of deep reinforcement learning to select customized dispatching rules formed by weighted combinations of production parameters on a single machine production scheduling problem. Due to the curse of dimensionality of storing Q values for all possible production states in a Q-table, a deep Q network is trained for the dynamic selection of the customized dispatching rules. Preliminary results show its effectiveness in minimizing total tardiness and outperform well-known existing dispatching rules.
更多查看译文
关键词
Artificial intelligence,intelligent manufacturing,data/information modeling,customized dispatching rule,deep reinforcement learning
AI 理解论文
溯源树
样例
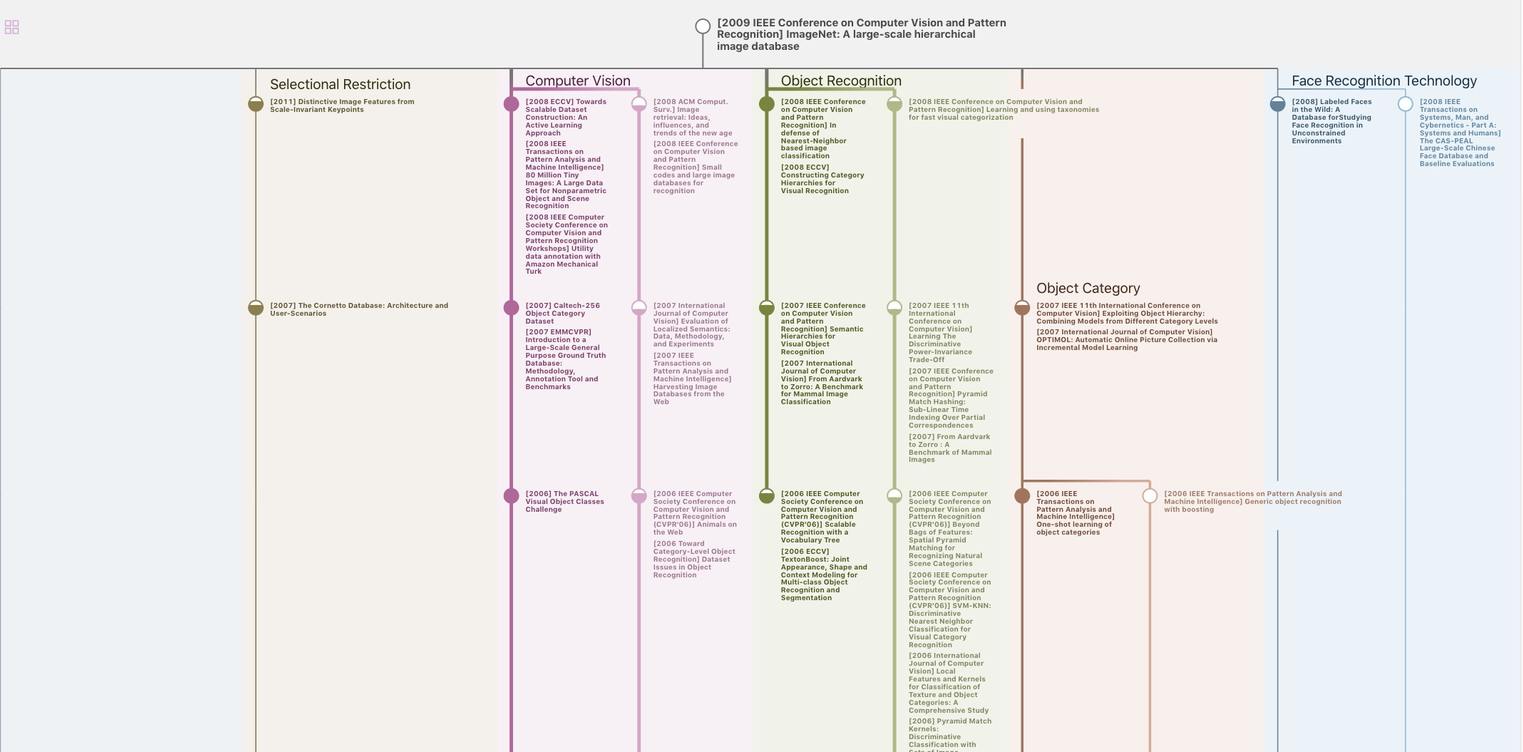
生成溯源树,研究论文发展脉络
Chat Paper
正在生成论文摘要