Multimodal deep learning: An improvement in prognostication or a reflection of batch effect?
Cancer Cell(2023)
摘要
Chen et al. recently reported a novel artificial intelligence (AI) approach for the multimodal fusion (MMF) of genomic and histologic data to more accurately predict the prognosis of 14 cancer types in The Cancer Genome Atlas (TCGA). 1 Chen R.J. Lu M.Y. Williamson D.F. Chen T.Y. Lipkova J. Noor Z. Shaban M. Shady M. Williams M. Joo B. Mahmood F. Pan-cancer integrative histology-genomic analysis via multimodal deep learning. Cancer Cell. 2022; 40: 865-878.e6https://doi.org/10.1016/j.ccell.2022.07.004 Abstract Full Text Full Text PDF PubMed Scopus (20) Google Scholar This is the largest study to date which combines image data and genomic data for outcome prediction in cancer. The authors found that in 12 of the 14 cancer types tested, MMF outperformed attention-based multiple-instance learning (MIL) on histology alone and self-normalized network (SSN) on genomic data alone. They also compared the combined histology/genomic models to Cox models trained on age, sex, and grade, demonstrating the superiority of MMF in prognostication. Such an approach may better characterize cancer biology and prognosis by accurately integrating features derived from both gene expression data and digital histology. Pan-cancer integrative histology-genomic analysis via multimodal deep learningChen et al.Cancer CellAugust 08, 2022In BriefChen et al. present a pan-cancer analysis that uses deep learning to integrate whole-slide pathology images and molecular features to predict cancer prognosis, with multimodal interpretability used to elucidate morphologic and molecular correlates of prognosis. Full-Text PDF Open Access
更多查看译文
关键词
multimodal deep learning,prognostication,deep learning,batch effect
AI 理解论文
溯源树
样例
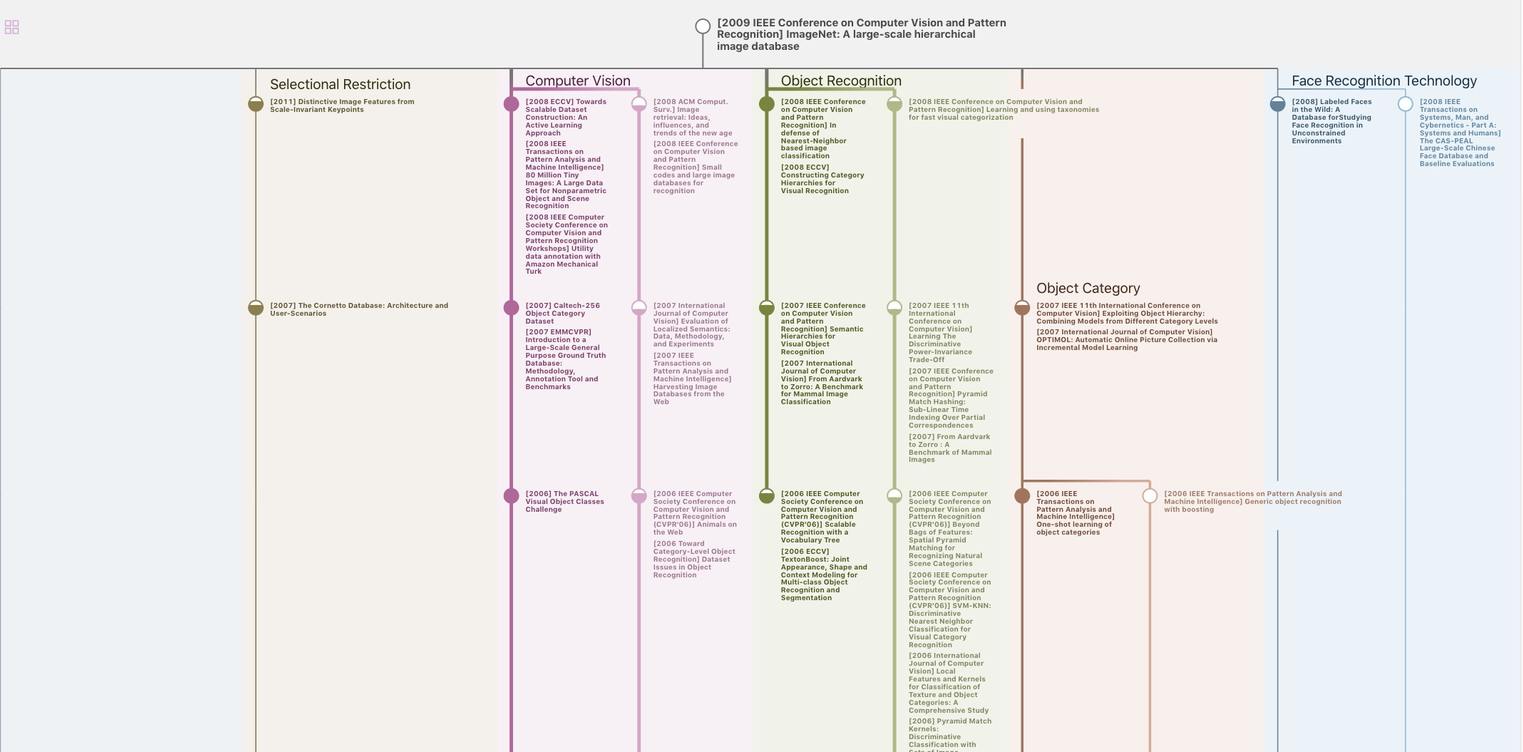
生成溯源树,研究论文发展脉络
Chat Paper
正在生成论文摘要