Multi-Population Evolution based Dynamic Constrained Multiobjective Optimization under Diverse Changing Environments
IEEE Transactions on Evolutionary Computation(2023)
摘要
Dynamic constrained multiobjective optimization involves irregular changes in the distribution of the true Pareto-optimal fronts, drastic changes in the feasible region caused by constraints, and the movement directions and magnitudes of the optimal distance variables due to diverse changing environments. To solve these problems, we propose a multi-population evolution based dynamic constrained multiobjective optimization algorithm. In this algorithm, we design a tribe classification operator to divide the population into different tribes according to a feasibility check and the objective values, which is beneficial for driving the population toward the feasible region and Pareto-optimal fronts. Meanwhile, a population selection strategy is proposed to identify promising solutions from tribes and exploit them to update the population. The optimal values of the distance variables vary differently with dynamic environments, thus, we design a dynamic response strategy for solutions in different tribes that estimates their distances to approach the Pareto-optimal fronts and regenerates a promising population when detecting environmental changes. In addition, a scalable generator is designed to simulate diverse movement directions and magnitudes of the optimal distance variables in real-world problems under dynamic environments, obtaining a set of improved test problems. Experimental results show the effectiveness of test problems, and the proposed algorithm is impressively competitive with several chosen state-of-the-art competitors.
更多查看译文
关键词
Dynamic constrained multiobjective optimization,tribe classification operator,population selection,dynamic response
AI 理解论文
溯源树
样例
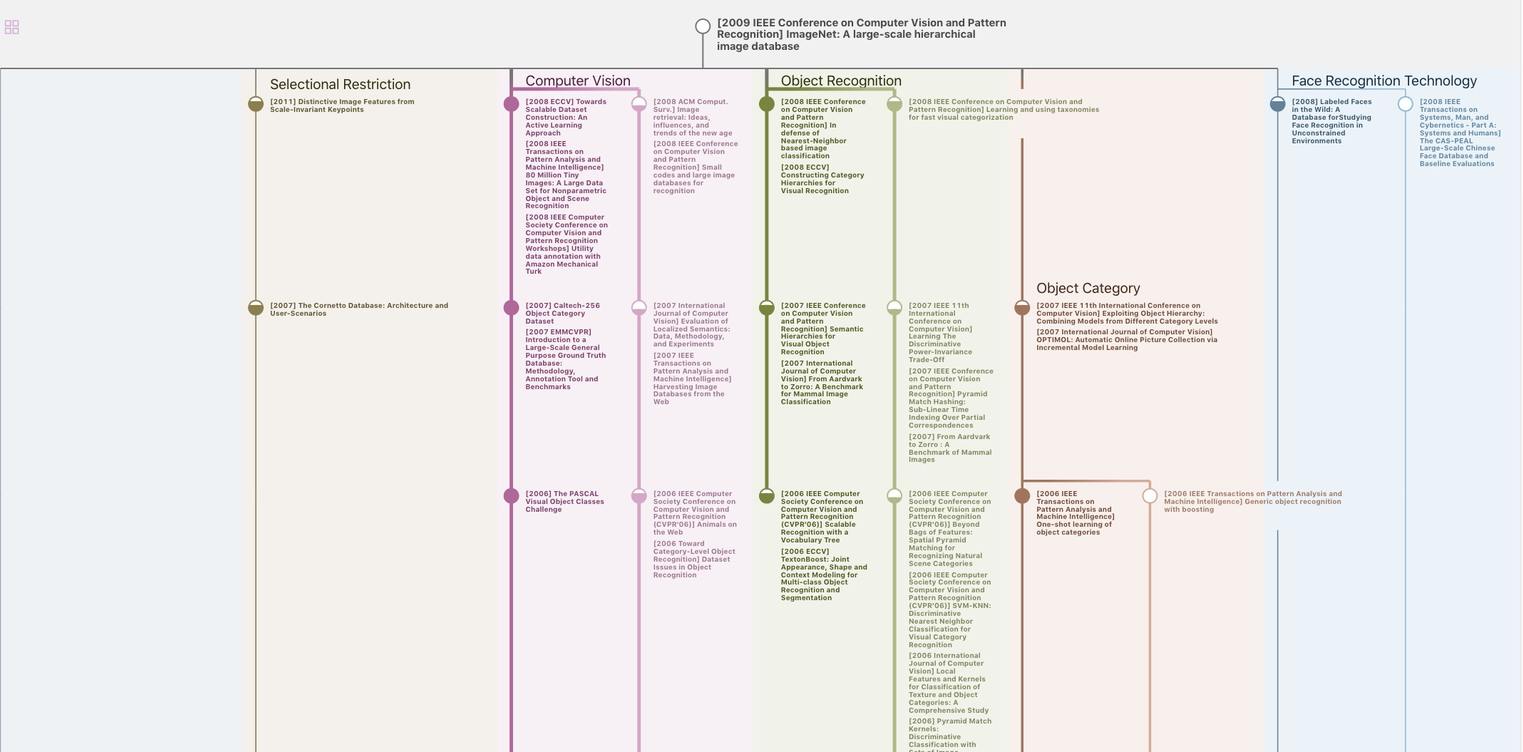
生成溯源树,研究论文发展脉络
Chat Paper
正在生成论文摘要