Classification-Directed Conceptual Structure Design Based on Topology Optimization, Deep Clustering, and Logistic Regression
Volume 3A: 48th Design Automation Conference (DAC)(2022)
摘要
Abstract The structure design of mechanical parts and components has been a significant theme of design automation. Today, topology optimization techniques have become relevant for effectively embodying optimal geometries of structures. However, their optimality is restricted to a particular category through design conditions, parameters, and optimization settings. When viewing the structure design as conceptual design, identifying the optimal category is essential rather than precise details. The category means configuration, morphology, or form rather than shape or geometry. This paper proposes a conceptual structure design framework for overcoming this gap. The framework considers that conceptual design results from classifying potentially possible geometries and identifying the best appropriate category from them. In detail, a topology optimization technique generates diverse optimal geometries under various settings of conditions and parameters, a deep clustering technique, i.e., the variational deep embedding, clusters them into several categories, and a logistic regression technique retrieves the criteria that distinct respective categories as design knowledge. A designer can interactively identify the relevant criteria that lead to the optimal structure for the design requirement by simultaneously revealing and refining those criteria under the retrieved knowledge. This paper applies the framework to a simple bridge design problem to demonstrate its validity and possibilities.
更多查看译文
AI 理解论文
溯源树
样例
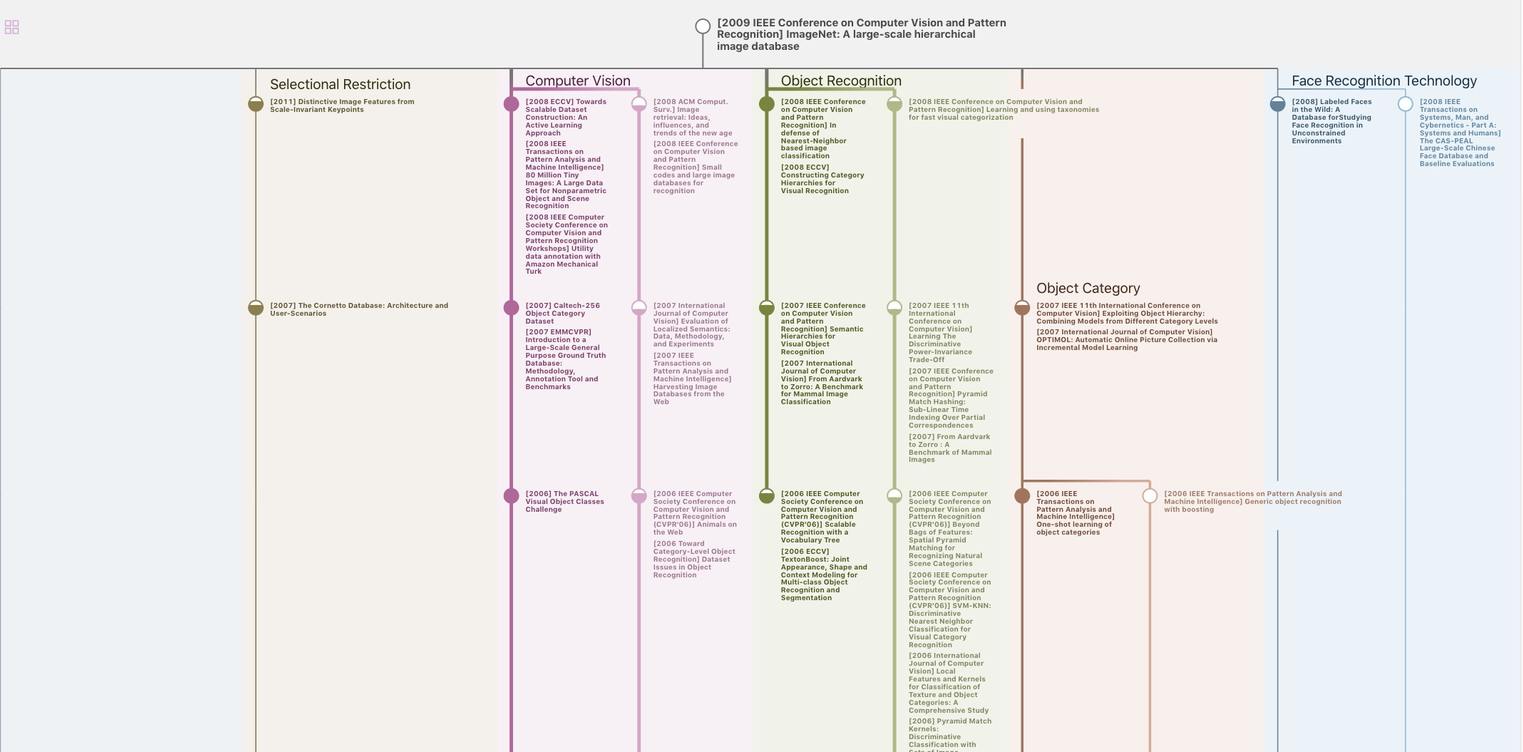
生成溯源树,研究论文发展脉络
Chat Paper
正在生成论文摘要