Text embedding models yield high-resolution insights into conceptual knowledge from short multiple-choice quizzes
crossref(2023)
摘要
We develop a mathematical framework, based on natural language processing models, for tracking and characterizing the acquisition of conceptual knowledge. Our approach embeds each concept in a high-dimensional representation space, where nearby coordinates reflect similar or related concepts. We test our approach using behavioral data from participants who answered small sets of multiple-choice quiz questions, interleaved between watching two course videos from the Khan Academy platform. We apply our framework to the videos' transcripts and the text of the quiz questions to quantify the content of each moment of video and each quiz question. We use these embeddings, along with participants' quiz responses, to track how the learners' knowledge changed after watching each video. Our findings show how a small set of quiz questions may be used to obtain rich and meaningful high-resolution insights into what each learner knows, and how their knowledge changes over time as they learn.
更多查看译文
AI 理解论文
溯源树
样例
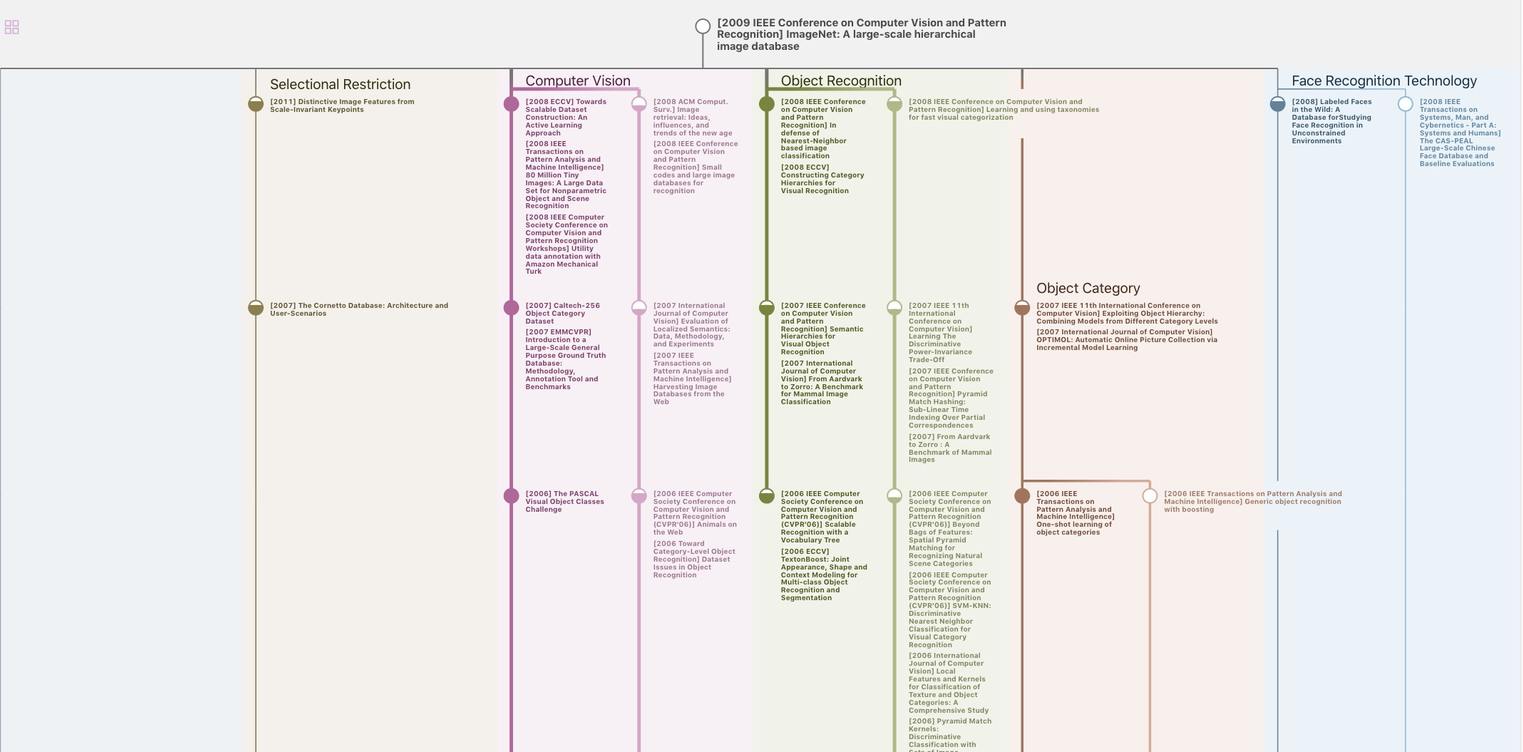
生成溯源树,研究论文发展脉络
Chat Paper
正在生成论文摘要