Learning a Compact Spatial-Angular Representation for Light Field
IEEE TRANSACTIONS ON MULTIMEDIA(2023)
摘要
The recent emergence of light field technology has led to new opportunities for immersive visual communication that has a need for high spatial and angular resolution, both of which contribute to a large image storage footprint and high-latency transmission. Task-driven downsampling methods have been proposed as a solution, and have shown improvements in single-image restoration. However, they are inevitable to disregard light field's intrinsic properties in the corresponding tasks. In this paper, we propose a light-field-specific task-driven downsampling framework, called the LFCrNet. The LFCrNet operates on a learning-based decreasing and increasing resolution in an end-to-end manner in order to utilize a cross-view asymmetric sampling technique. In detail, it separates raw data into disparity and non-disparity patterns by measuring pixel-wise residuals between the sub-aperture central view and auxiliary views. Then, a chain of 3-D deformable residual blocks (DRBs) is used to extract disparity features and manage these features regard of their intrinsic property individually. Afterwards, they are compacted into spatio-angular domains through a 3-D deformable downsampler (3-DDS). The non-disparity information is integrated into a separate pipeline that leverages spatial similarity across multiple light field views. This technique is capable of preserving specific occlusion components, and subsequently, restoring them using a learning-based upscaling method to generate a high-quality reconstruction. In general, our method has shown superior performance on multiple open-source datasets by a significant margin.
更多查看译文
关键词
Electronic mail,Superresolution,Sun,feature extraction,image reconstruction,spatial resolution,super-resolution,task analysis
AI 理解论文
溯源树
样例
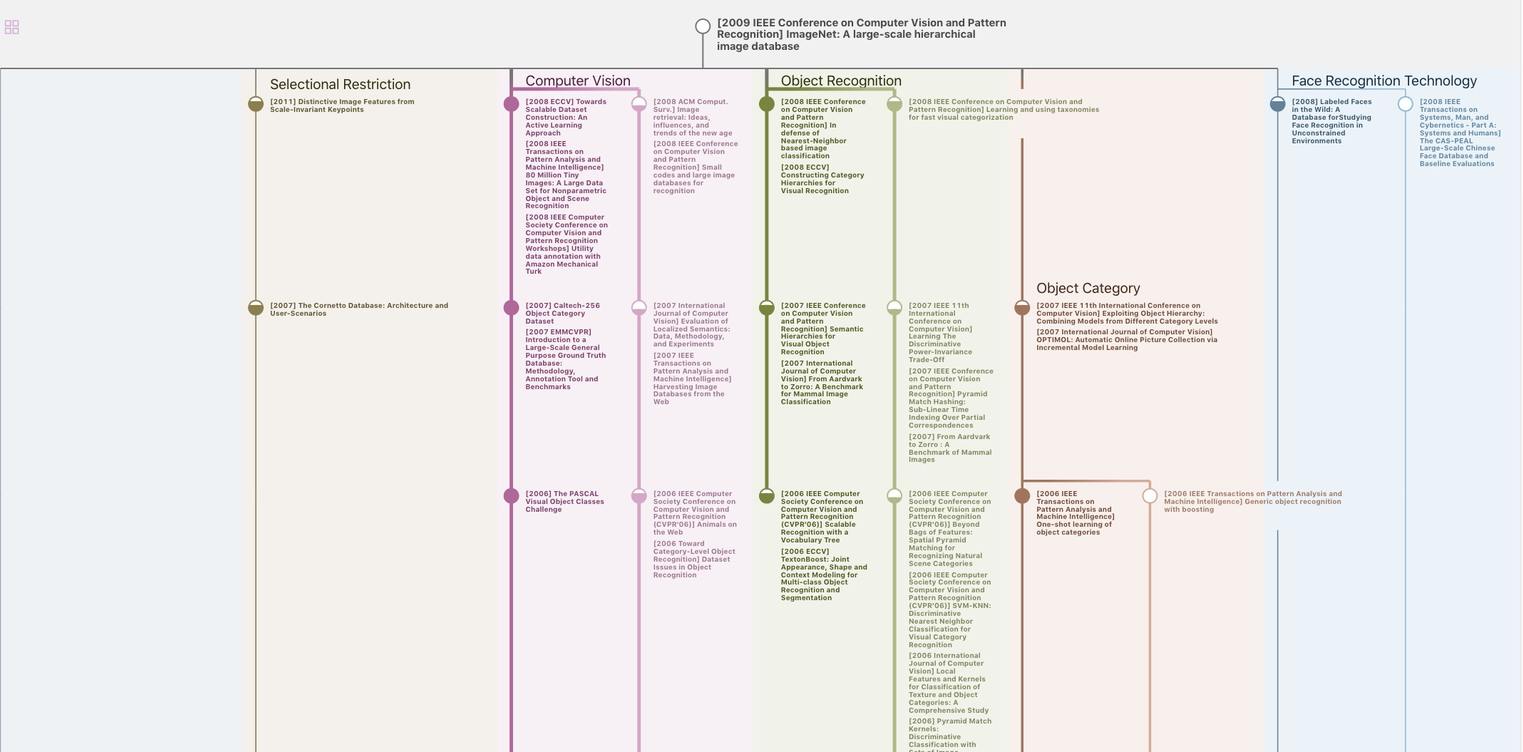
生成溯源树,研究论文发展脉络
Chat Paper
正在生成论文摘要