Enhancing Aspect-Based Sentiment Analysis Using a Dual-Gated Graph Convolutional Network Via Contextual Affective Knowledge
SSRN Electronic Journal(2023)
摘要
The primary goal of aspect-based sentiment analysis is to identify sentiment polarity concerning the given aspect in a sentence. Recent investigations have demonstrated the superior performance of graph convolutional neural network (GCN) on dependency parsing tree. However, these GCN-based models fail to take the given aspect into account when calculating the hidden node representation vector, as well as lack exploration of contextual commonsense knowledge. On the contrary, the gating mechanism enables the interaction of the context and the given aspect to enhance the impact of the given aspect on the context. Nevertheless, such interactions are frequently inadequate resulting in insufficient extraction of sentiment information. This paper proposes a dualgated graph convolutional network via contextual affective knowledge (DGGCN) to address these issues. The core idea is to incorporate GCN into the gating mechanism to enhance GCN to fully aggregate node information while strengthening the concentration on the given aspect. Simultaneously, the incorporation of contextual affective knowledge into graph networks can refine the perception of affective features. Experimental findings on five benchmark datasets reveal that our proposed DGGCN surpasses state-of-the-art methods.
更多查看译文
关键词
contextual affective knowledge,sentiment analysis,convolutional network,aspect-based,dual-gated
AI 理解论文
溯源树
样例
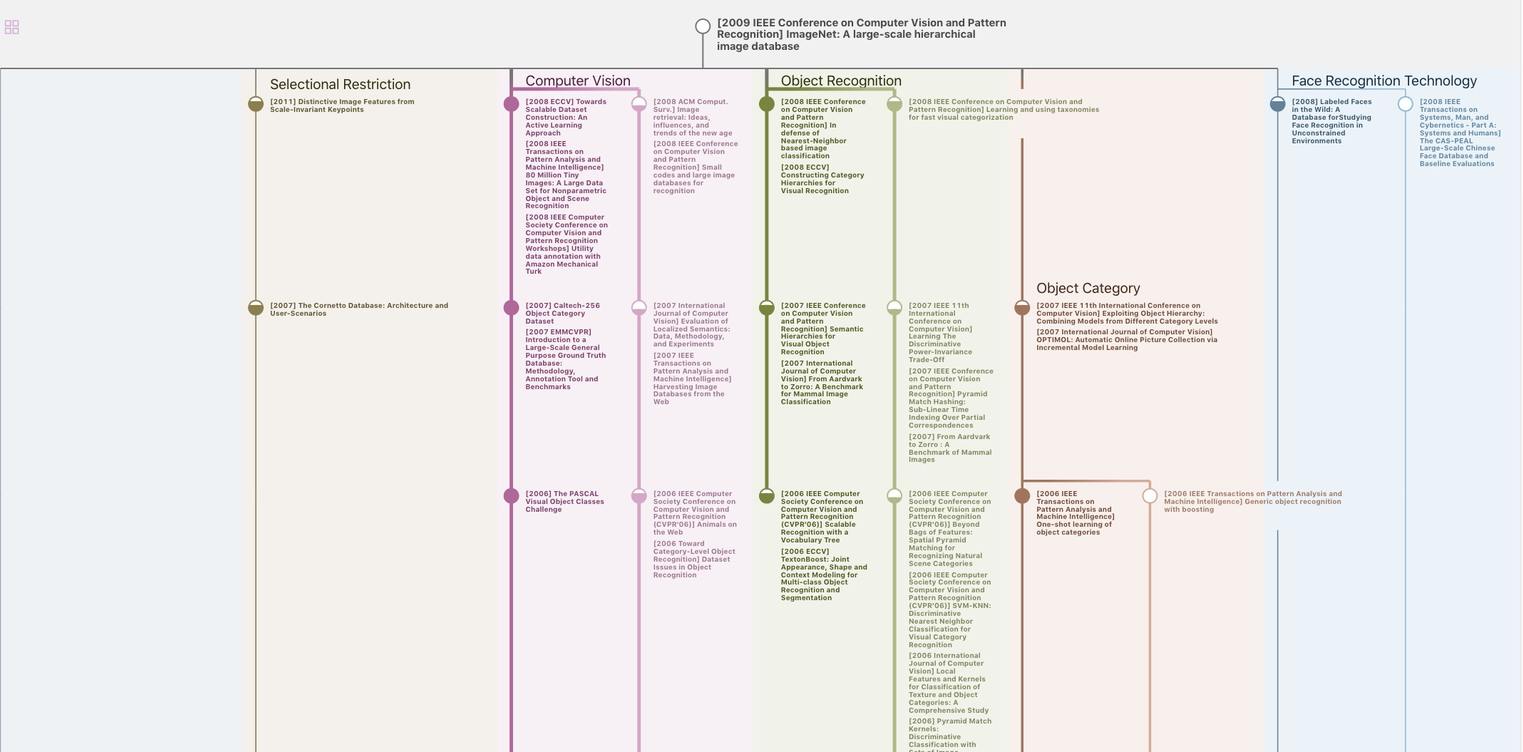
生成溯源树,研究论文发展脉络
Chat Paper
正在生成论文摘要