A Probabilistic Contrastive Framework for Semi-Supervised Learning
IEEE TRANSACTIONS ON MULTIMEDIA(2023)
摘要
Semi-supervised learning is a common way that investigates how to improve performance of a visual learning model, while data annotation is far from sufficient. Recent works in semi-supervised deep learning have successfully applied consistency regularization, which encourages a model to maintain consistent predictions for different perturbed versions of an image. However, most of such methods ignore the category correlation of image features, especially when exploiting strong augmentation methods for unlabeled images. To address this problem, we propose PConMatch, a model that leverages a probabilistic contrastive learning framework to separate the features of strongly-augmented versions from different classes. A semi-supervised probabilistic contrastive loss is designed, which takes both labeled and unlabeled samples into account and develops an auxiliary module to generate a probability score to measure the model prediction confidence for each sample. Specifically, PConMatch first generates a pair of weakly-augmented versions for each labeled sample, and produces a weakly-augmented version and a corresponding pair of strongly-augmented versions for each unlabeled sample. Second, a probability score module is proposed to assign pseudo-labeling confidence scores to strongly-augmented unlabeled images. Finally, the probability score of each sample is further passed to the contrastive loss, combining with consistency regularization to enable the model to learn better feature representations. Extensive experiments on four publicly available image classification benchmarks demonstrate that the proposed approach achieves state-of-the-art performance in image classification. Several rigorous ablation studies are conducted to validate the effectiveness of the method.
更多查看译文
关键词
Task analysis,Visualization,Predictive models,Semantics,Probabilistic logic,Image classification,Annotations,Semi-supervised learning,contrastive learning,image classification,consistency regularization,data augmen- tation,Pseudo-labeling
AI 理解论文
溯源树
样例
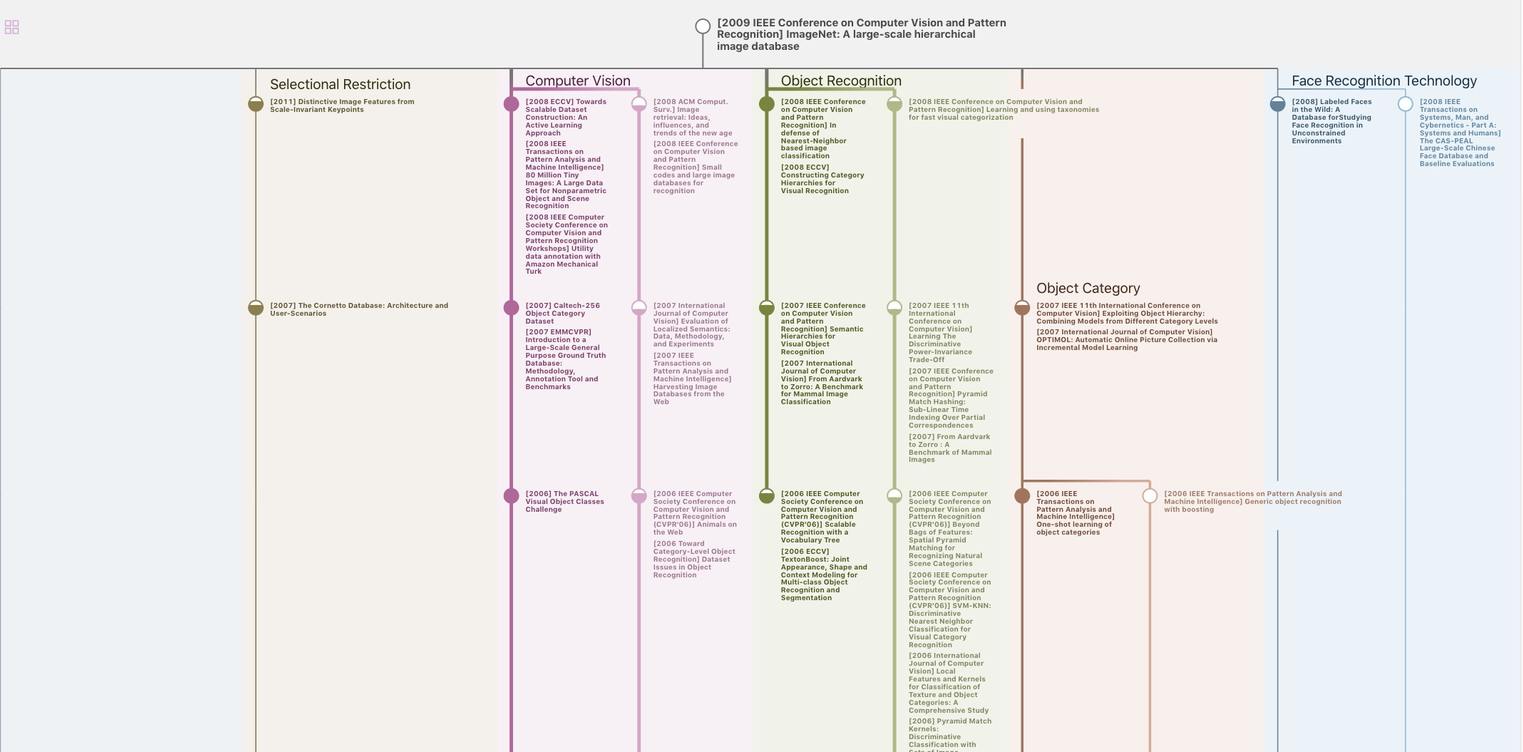
生成溯源树,研究论文发展脉络
Chat Paper
正在生成论文摘要