VAMYOLOX: An Accurate and Efficient Object Detection Algorithm Based on Visual Attention Mechanism for UAV Optical Sensors
IEEE Sensors Journal(2023)
摘要
Unmanned aerial vehicles (UAVs) have been widely used in various fields. As one of the key technologies in improving the autonomous sensing ability of UAV optical sensors, object detection has become a research focus in recent years. Since UAVs usually navigate at different vertical heights, the object scales and sensor field of view change violently, which brings a great difficulty to the optimization of the model. Moreover, when a UAV is flying at low level rapidly, it may cause the motion blur phenomenon on objects that are highly dense in position, leading to great challenge for distinction of these objects. To address the extremely tough problems discussed above, we propose an accurate and efficient object detection algorithm, namely, VAMYOLOX. Based on YOLOX, we first redesigned the classification and regression loss function of the model to better conduct classification and localization under complex motion blur and dense scenes. Then, we increase another prediction head to detect lots of tiny objects to ultimately improve the detection ability of the model for multiscale objects. Finally, we redesigned the neck of the network by introducing the triplet attention module (TAM) to find attention regions in scenes with dense objects and in images that cover a large area, accordingly enhancing the features extracted by the backbone network. Extensive experiments on the VisDrone dataset widely used in the research of UAV image object detection show that VAMYOLOX has achieved the state-of-the-art (SOTA) performance with good interpretability in UAV optical sensors captured scenes. On the VisDrone-DET-test-dev subset, the average precision (AP) of VAMYOLOX is 25.31%, outperforming the previous SOTA model (CornerNet) by 1.88%. On the VisDrone-DET-val subset, the AP of our method is 29.4%, achieving a highly competitive result with previous SOTA method (AMRNet). Not only that, VAMYOLOX achieves a maximum improvement of 2.72% compared to the AP of the baseline model (YOLOX). In addition, compared with other methods, our method has a significant advantage in speed and can meet the needs of different scenarios. The PyTorch code and trained models are available at https://github.com/yangyahu-1994/VAMYOLOX.
更多查看译文
关键词
Object detection, Autonomous aerial vehicles, Optical sensors, Feature extraction, Sensors, Head, Neck, Attention mechanism, deep learning (DL), object detection, optical sensor, unmanned aerial vehicle (UAV) imagery
AI 理解论文
溯源树
样例
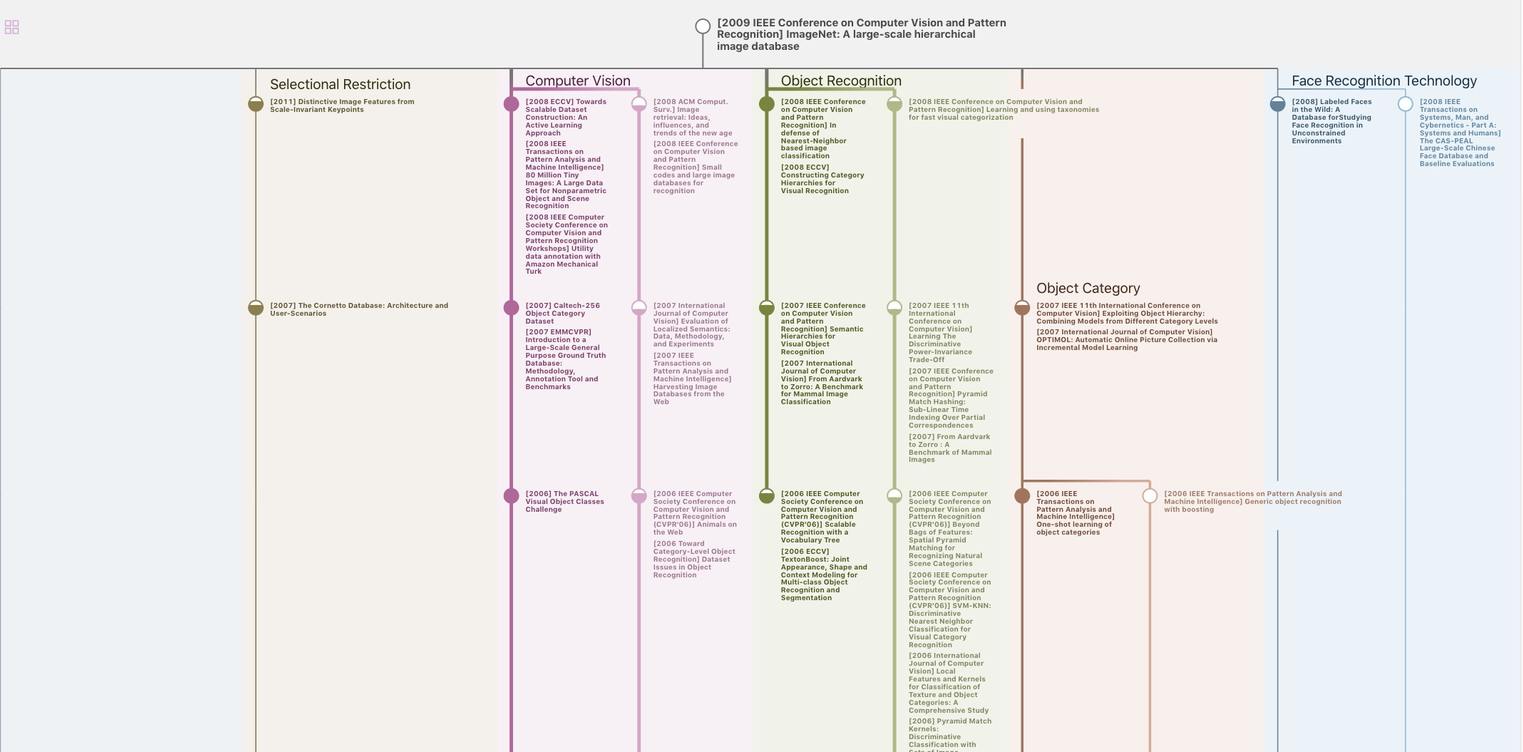
生成溯源树,研究论文发展脉络
Chat Paper
正在生成论文摘要