Dual-space Hierarchical Learning for Goal-guided Conversational Recommendation
NEUROCOMPUTING(2024)
摘要
Proactively and naturally guiding the dialog from the non -recommendation context (e.g., Chit-chat) to the recommendation scenario (e.g., Music) is crucial for the Conversational Recommender System (CRS). Prior studies mainly focus on planning the next dialog goal (e.g., chat on a movie star) conditioned on the previous dialog. However, we find the dialog goals can be simultaneously observed at different levels, which can be utilized to improve CRS. In this paper, we propose Dual -space Hierarchical Learning (DHL) to leverage multi -level goal sequences and their hierarchical relationships for conversational recommendation. Specifically, we exploit multi -level goal sequences from both the representation space and the optimization space. In the representation space, we propose the hierarchical representation learning where a cross attention module derives mutually enhanced multi -level goal representations. In the optimization space, we devise the hierarchical weight learning to reweight lower -level goal sequences, and introduce bi-level optimization for stable update. Additionally, we propose a soft labeling strategy to guide optimization gradually. Experiments on two real -world datasets verify the effectiveness of our approach. Code and data are available here.
更多查看译文
关键词
Conversational recommender system,Data mining,Bi-level optimization
AI 理解论文
溯源树
样例
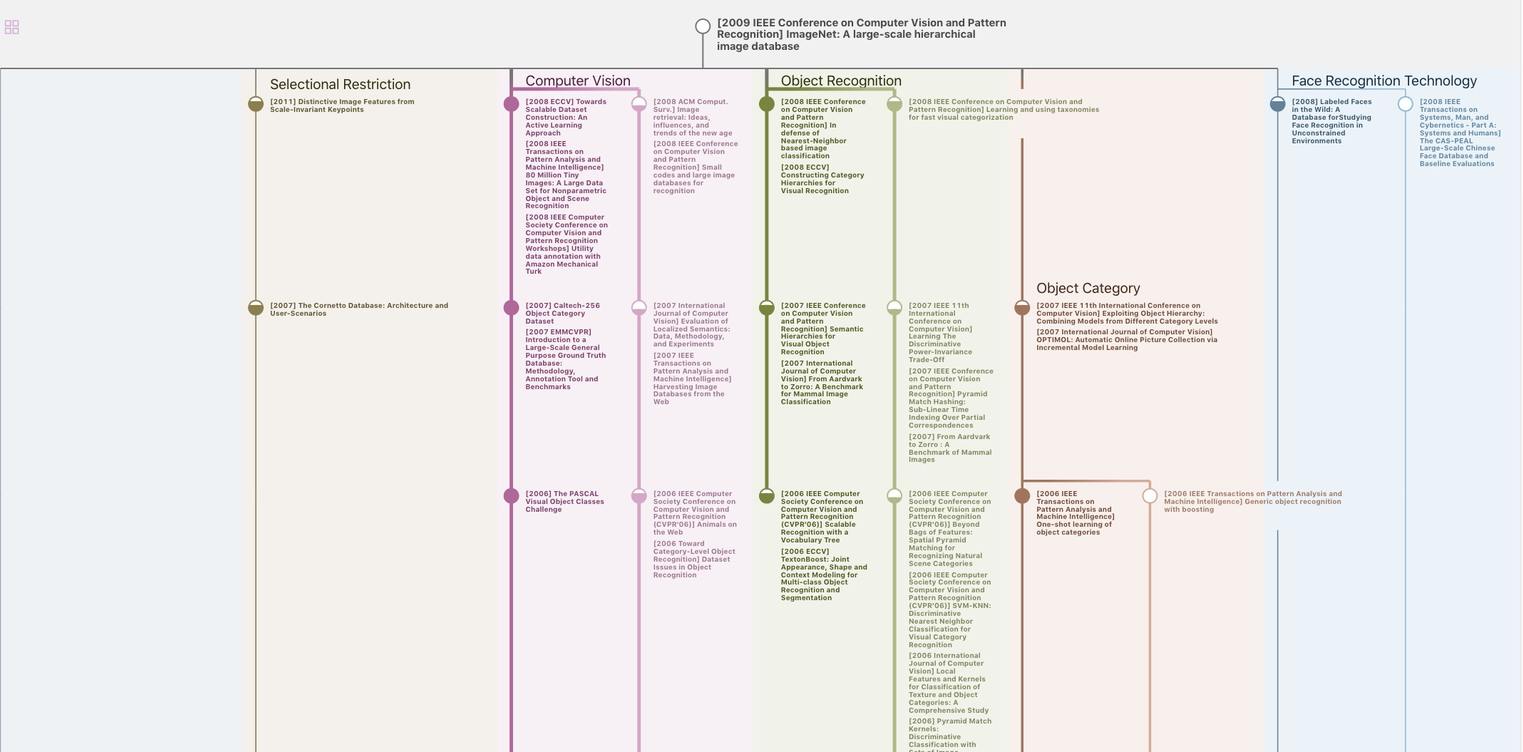
生成溯源树,研究论文发展脉络
Chat Paper
正在生成论文摘要