Graph-Based Non-Sampling for Knowledge Graph Enhanced Recommendation
IEEE Transactions on Knowledge and Data Engineering(2023)
摘要
Knowledge graph (KG) enhanced recommendation, which aims to solve the cold start and explainability in recommender systems, has attracted considerable research interest recently. Existing recommender systems usually focus on implicit feedback such as purchase history without negative feedback. Most of them apply the negative sampling strategy to deal with the implicit feedback data, which may ignore the latent positive user-item interaction. Some other works adopt the non-sampling strategy that treats all non-observed interactions as negative samples and assigns a weight for each negative sample to represent the probability that this sample is a positive sample. However, they use a simple and intuitive weight assignment strategy and cannot catch the latent relationship from all interaction data. To address these problems, we consider graph structure information of both user-item interaction and knowledge graph, and propose a Graph-based Non-Sampling strategy to achieve efficient performance in Knowledge graph enhanced Recommendation (GNSKR). GNSKR utilizes node centrality to significantly improve recommendation performance with low computation cost. Meanwhile, we combine knowledge graph embedding and recommendation task with a local aggregation block, which efficiently catches the high-order connection information in KG enhanced recommendation. Experiments on three datasets show that GNSKR embraces the state-of-the-art with competitive efficiency.
更多查看译文
关键词
Graph neural network,knowledge graph enhanced recommendation,knowledge graph embedding,non-sampling strategy
AI 理解论文
溯源树
样例
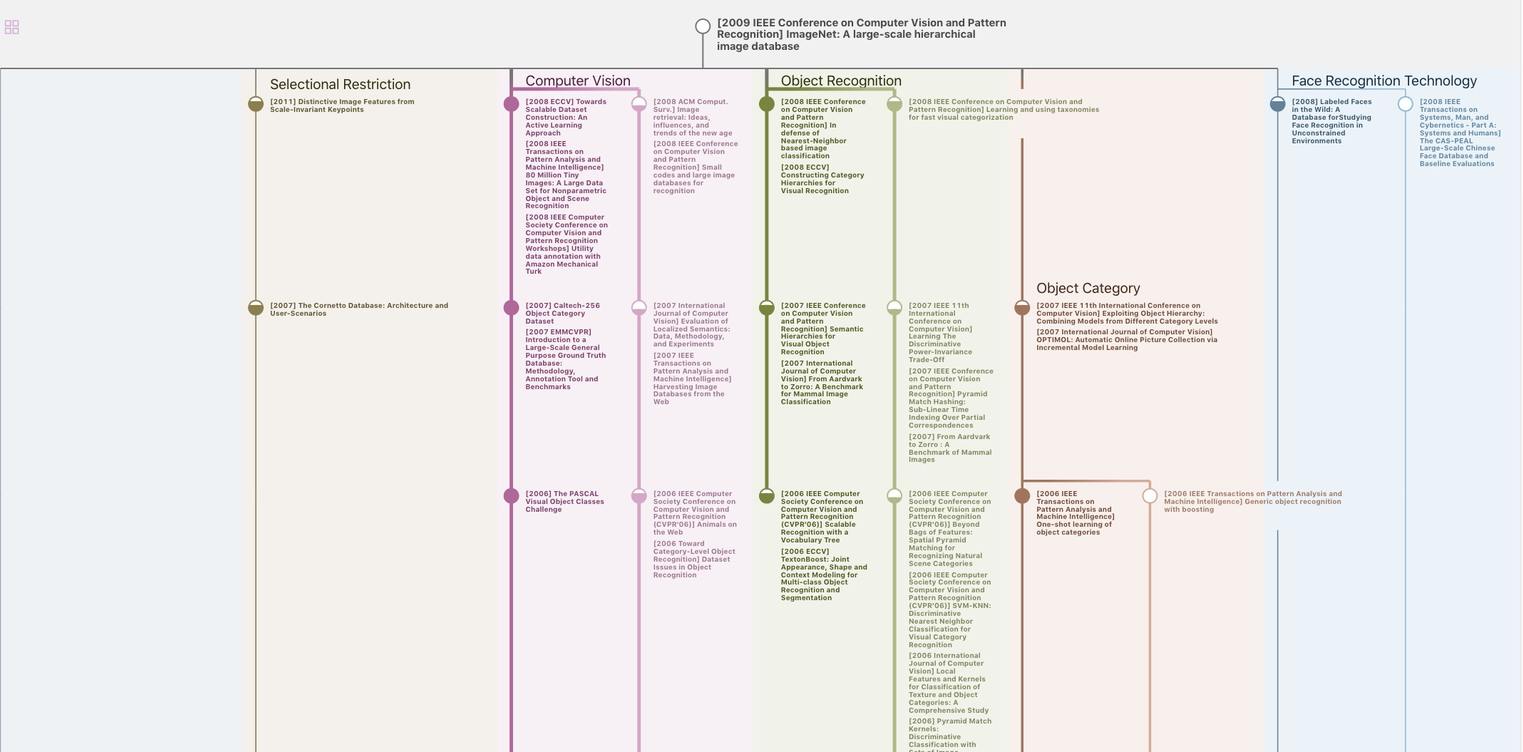
生成溯源树,研究论文发展脉络
Chat Paper
正在生成论文摘要