MOTO: Mobility-Aware Online Task Offloading with Adaptive Load Balancing in Small-Cell MEC
IEEE transactions on mobile computing(2024)
摘要
Mobile edge computing is a promising computing paradigm enabling mobile devices to offload computation-intensive tasks to nearby edge servers. However, within small-cell networks, the user mobilities can result in uneven spatio-temporal loads, which have not been well studied by considering adaptive load balancing, thus limiting the system performance. Motivated by the data analytics and observations on a real-world user association dataset in a large-scale WiFi system, in this paper, we investigate the mobility-aware online task offloading problem with adaptive load balancing to minimize the total computation costs. However, the problem is intractable directly without prior knowledge of future user mobility behaviors and spatio-temporal computation loads of edge servers. To tackle this challenge, we transform and decompose the original task offloading optimization problem into two sub-problems, i.e., task offloading control ( ToC ) and server grouping ( SeG ). Then, we devise an online control scheme, named MOTO (i.e., M obility-aware O nline T ask O ffloading), which consists of two components, i.e., Long Short Term Memory based algorithm and Dueling Double DQN based algorithm, to efficiently solve the ToC and SeG sub-problems, respectively. Extensive trace-driven experiments are carried out and the results demonstrate the effectiveness of MOTO in reducing computational costs of mobile devices and achieving load balancing when compared to the state-of-the-art benchmarks.
更多查看译文
关键词
Mobile edge computing,load balance,mobility-aware task offloading,reinforcement learning
AI 理解论文
溯源树
样例
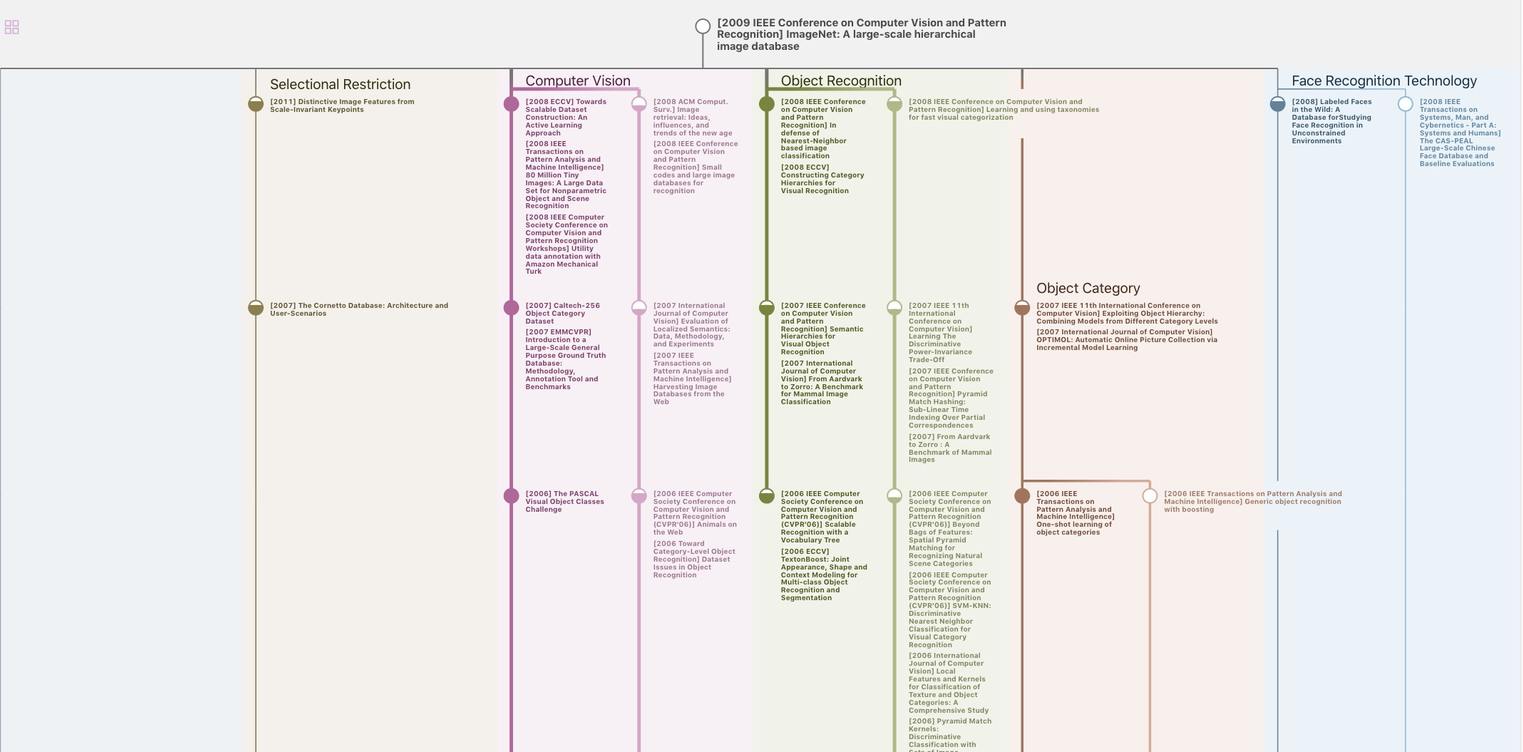
生成溯源树,研究论文发展脉络
Chat Paper
正在生成论文摘要