Singular Value Decomposition (SVD) Co-training: A Semi-supervised Method for Object Detection
Journal of Physics: Conference Series(2022)
摘要
Existing object detection algorithms based on Convolutional Neural Network (CNN) generally rely on supervised learning with a large amount of labeled samples, which is very time-consuming and laborious. To address the problem, we propose a semi-supervised learning method by using Singular Value Decomposition (SVD) co-training. Specifically, this paper uses two mutually independent features which are obtained by SVD to guide the co-training of two detectors in one CNN model, meanwhile an adaptive self-labeling strategy is used to ensure the quality of pseudo labels. To verify the efficacy of SVD co-training, we conducted experiments on GMU, AVD and self-made BHID datasets. The result shows that SVD co-training could achieve the accuracy comparable to full-supervised learning by using only 5% of labeled data.
更多查看译文
关键词
singular value decomposition,svd,detection,co-training,semi-supervised
AI 理解论文
溯源树
样例
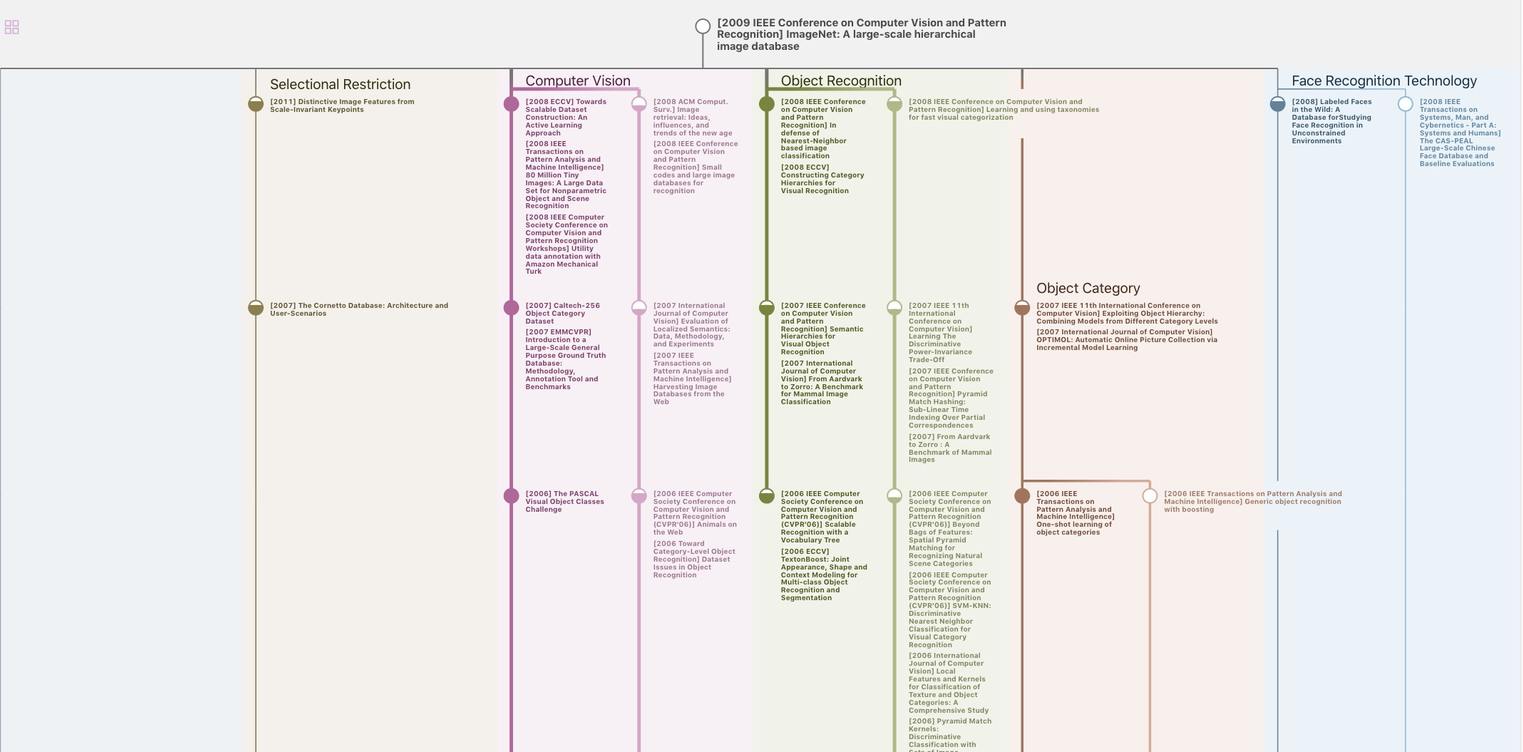
生成溯源树,研究论文发展脉络
Chat Paper
正在生成论文摘要