Using textual similarity to identify legal precedents: appraising machine learning models for administrative courts
SSRN Electronic Journal(2023)
摘要
Abstract Precedent is the cornerstone of the Common law system. Even in jurisdictions that follow Civil law, precedents constrain decisions when case law is sufficiently uniform. A systematic disregard of precedents makes judgments less coherent and the law less just. Nevertheless, relying on precedents can also make courts more efficient, whereas recent advances in natural language processing (NLP) and machine learning (ML) open doors for solutions to automated and reliable identification of similar cases. In this study, we investigated more than a hundred combinations of document representations and textual vectorization models to assess whether pairs of cases identified by the machine satisfy the human notion of similarity. To this point, analogous models have been evaluated using tiny validation samples. We used a statistically significant sample evaluated by legal experts from an administrative court in Brazil, constituting a gold standard sample. We also propose using evaluation metrics that are meaningful to real-world applications and build upon previous works, employing promising solutions and exploring the extraction of concepts and relationships from legal texts. The results demonstrate that such applications can identify a large proportion of similar cases that can be interpreted as legal precedents. Models that rely on more granular representations of text achieved the best performance. In addition, extracting concepts and relations proved to improve the results, while using models that are more complex and difficult to train may not be the best option. These findings can guide the development of recommendation systems to improve efficiency and consistency in law courts and motivate studies that explore other techniques for this purpose.
更多查看译文
关键词
administrative courts,textual similarity,legal precedents,machine learning
AI 理解论文
溯源树
样例
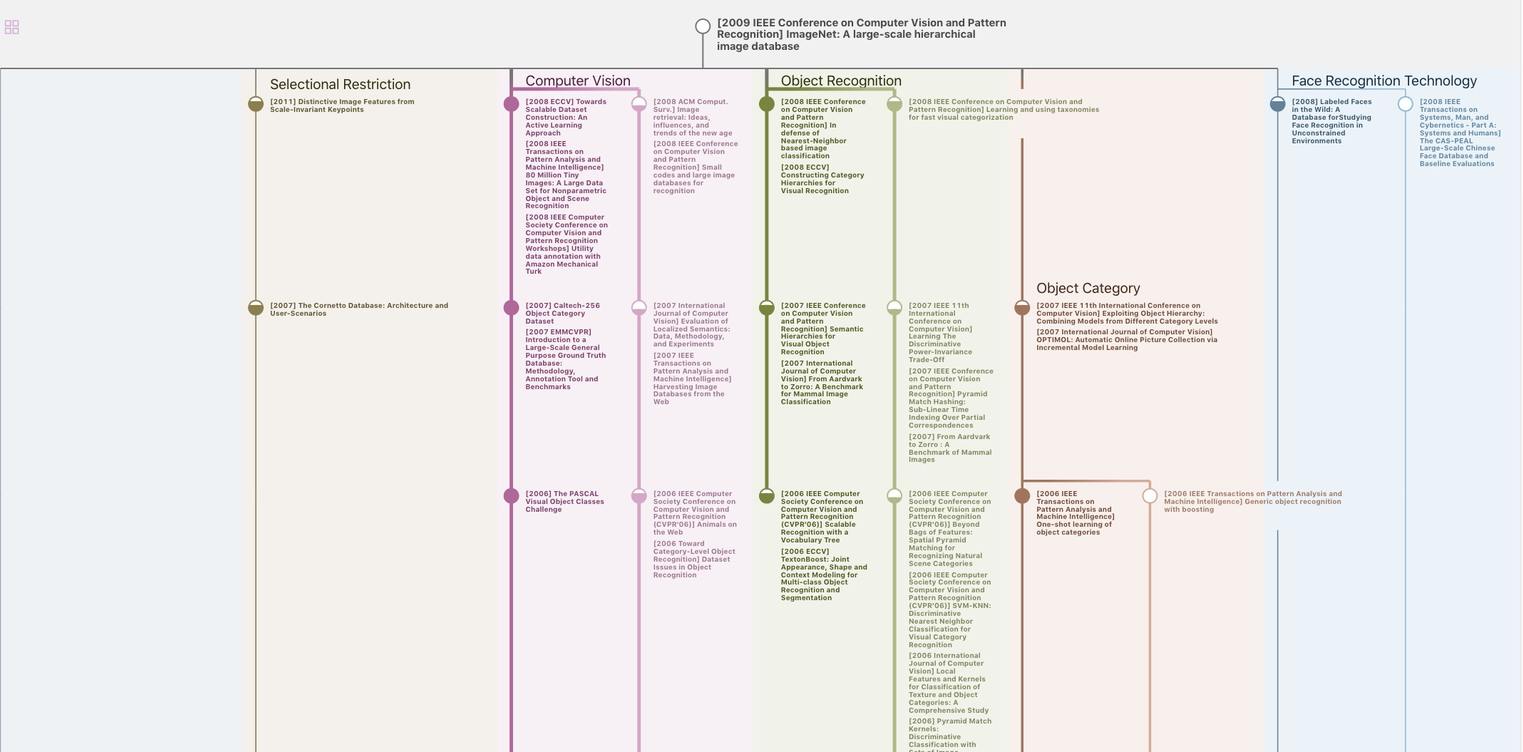
生成溯源树,研究论文发展脉络
Chat Paper
正在生成论文摘要