Differentiable Neural Architecture Search for Domain Adaptation in Fault Diagnosis
SSRN Electronic Journal(2023)
摘要
In recent years, the application of deep transfer learning in fault diagnosis has gained considerable traction. Notably, the utilization of domain adaptation methods to address the challenges of unsupervised transfer learning has emerged as a focal point of research inquiry. However, prevailing investigations primarily emphasize the construction of loss functions, leaving the design of network structures reliant on manual intervention. To overcome this limitation, a novel approach employing differentiable neural architecture search is proposed. This method facilitates the automatic exploration of network structures that are well-suited for domain adaptation in fault diagnosis. The proposed method explores two key components within the Inception search space: the arrangement of Inception blocks and the feature mask. By stacking Inception blocks, a comprehensive network architecture is constructed, while the feature mask selectively incorporates domain-relevant features. Moreover, to enhance search efficiency, simultaneous exploration and adaptation of multiple target domains are undertaken, thereby expanding the potential application domains of domain adaptation. The effectiveness of the proposed method is evaluated using 3 public datasets through a series of experiments, which demonstrate that this method exhibits a remarkable capacity for generating networks of satisfied performance.
更多查看译文
关键词
Fault diagnosis,Domain adaptation,Convolution neural network,Differentiable neural architecture search
AI 理解论文
溯源树
样例
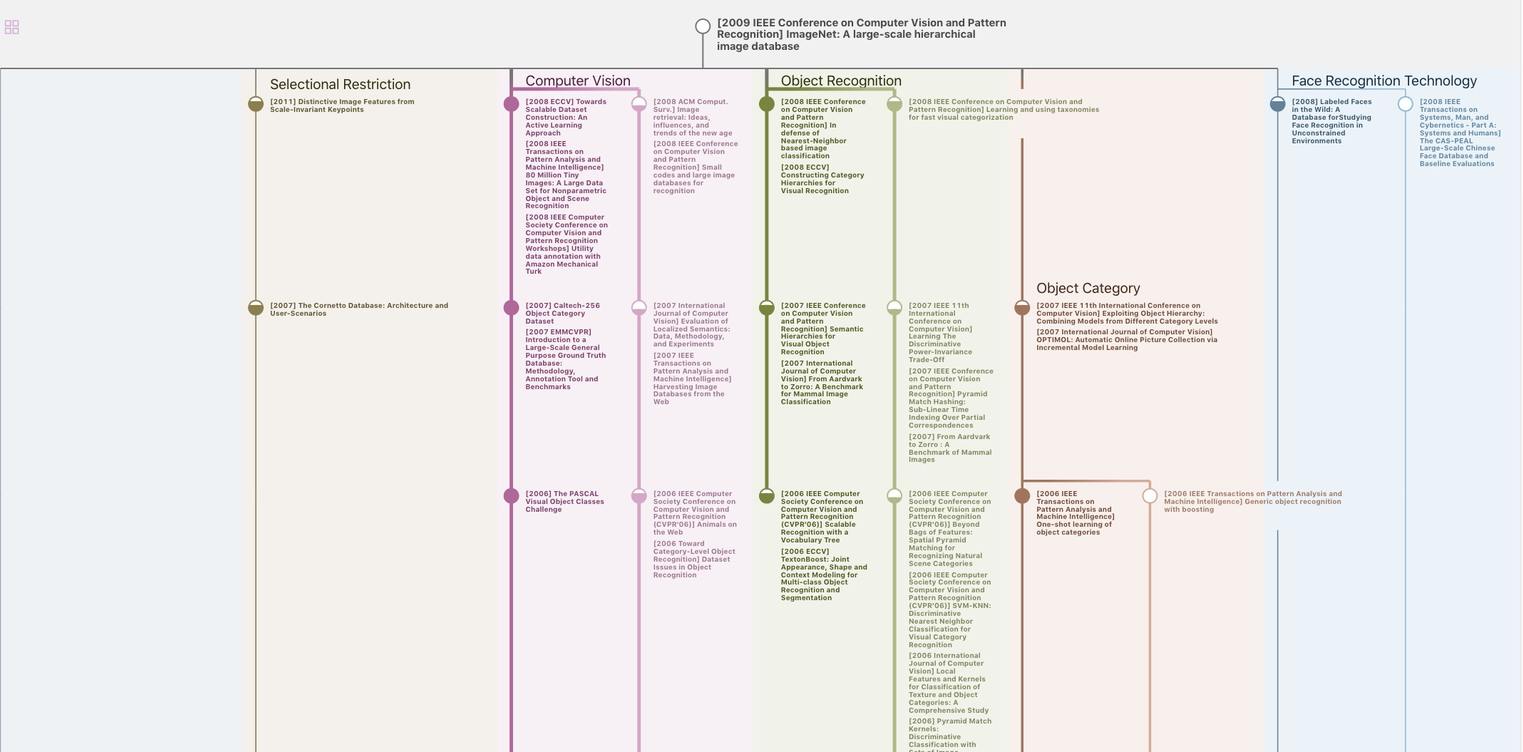
生成溯源树,研究论文发展脉络
Chat Paper
正在生成论文摘要