LEC-MTNN: a novel multi-frame infrared small target detection method based on spatial-temporal patch-tensor
Earth and Space: From Infrared to Terahertz (ESIT 2022)(2023)
摘要
Recently, many state-of-the-art methods have been proposed for infrared (IR) dim and small target detection, but the performance of IR small target detection still faces with challenges in complicated environments. In this paper, we propose a novel IR small target detection method named local entropy characterization prior with multi-mode weighted tensor nuclear norm (LEC-MTNN) that combines local entropy characterization prior (LEC) and multi-mode weighted tensor nuclear norm (MTNN). First, we transform the original infrared image sequence into a nonoverlapping spatial-temporal patch-tensor to fully utilize the spatial and temporal information in image sequences. Second, a nonconvex surrogate of tensor rank called MTNN is proposed to approximate background tensor rank, which organically combines the sum of the Laplace function of all the singular values and multi-mode tensor extension of the construct tensor without destroying the inherent structural information in the spatial-temporal tensor. Third, we introduce a new sparse prior map named LEC via an image entropy characterization operator and structure tensor theory, and more effective target prior can be extracted. As a sparse weight, it is beneficial to further preserve the targets and suppress the background components simultaneously. To solve the proposed model, an efficient optimization scheme utilizing the alternating direction multiplier method (ADMM) is designed to retrieve the small targets from IR sequence. Comprehensive experiments on four IR sequences of complex scenes demonstrate that LEC-MTNN has the superior target detectability (TD) and background suppressibility (BS) performance compared with other five state-of-the-art detection methods.
更多查看译文
关键词
Small target detection, multi-mode weighted tensor nuclear norm, local entropy characterization prior, target detectability, background suppressibility
AI 理解论文
溯源树
样例
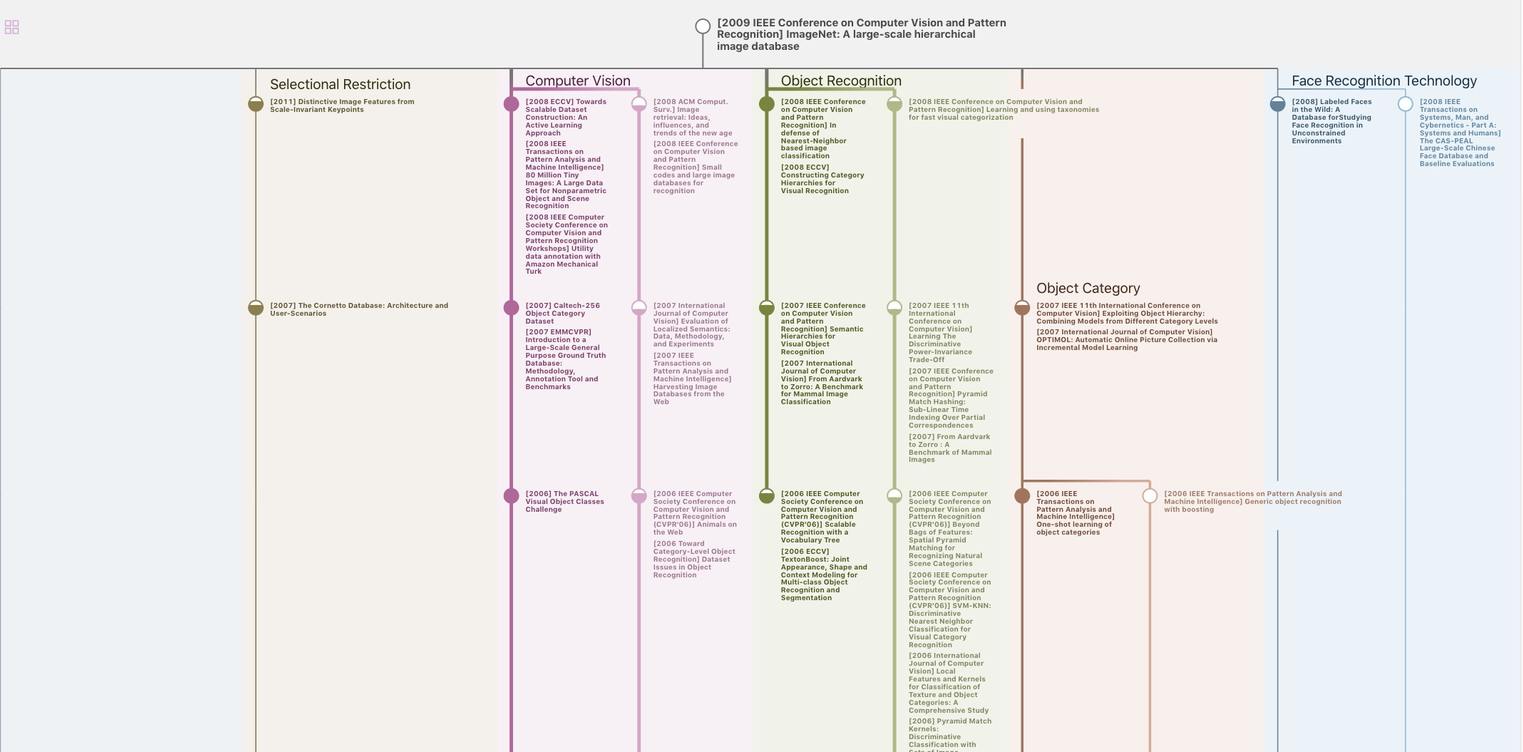
生成溯源树,研究论文发展脉络
Chat Paper
正在生成论文摘要