An embedded deep learning model discrepancy for computational combustion simulations
Journal of the Brazilian Society of Mechanical Sciences and Engineering(2024)
摘要
In combustion simulations, model discrepancies result from employing simplified physics or chemistry closure models for achieving a balance between easiness of computation and accuracy. Here, the focus is devoted to errors introduced by modeling the reactive process chemistry by reduced kinetics. Key questions involving modeling the error rely on where to introduce it within the model and on assuming its functional form. In this work, an embedded deep learning model error approach is proposed. Specifically, a deep neural network is embedded as an additive function to model the temporal evolution of chemical species concentrations. Embedding the model error naturally allows consistency with the underlying model physics. The deep neural network is supposed to capture an appropriate functional form for the model correction. The neural network is trained using data produced by numerical simulation of detailed mechanisms, in a model-to-model Bayesian calibration. The proposed strategy is evaluated in benchmark combustion scenarios, corresponding to the application cases of homogeneous combustion during autoignition and flame propagation, widely used to evaluate the role played by chemical kinetics on the main physicochemical properties characterizing combustion systems. The results demonstrate the ability of the adopted approach for model calibration in chemical kinetics, able to predict well the temporal evolution of thermochemical states for different ignition scenarios. Furthermore, the enhanced model has been successfully extended to describe the flame properties for the freely propagating laminar premixed flames.
更多查看译文
关键词
Combustion simulations,Biogas combustion,Deep learning,Model error
AI 理解论文
溯源树
样例
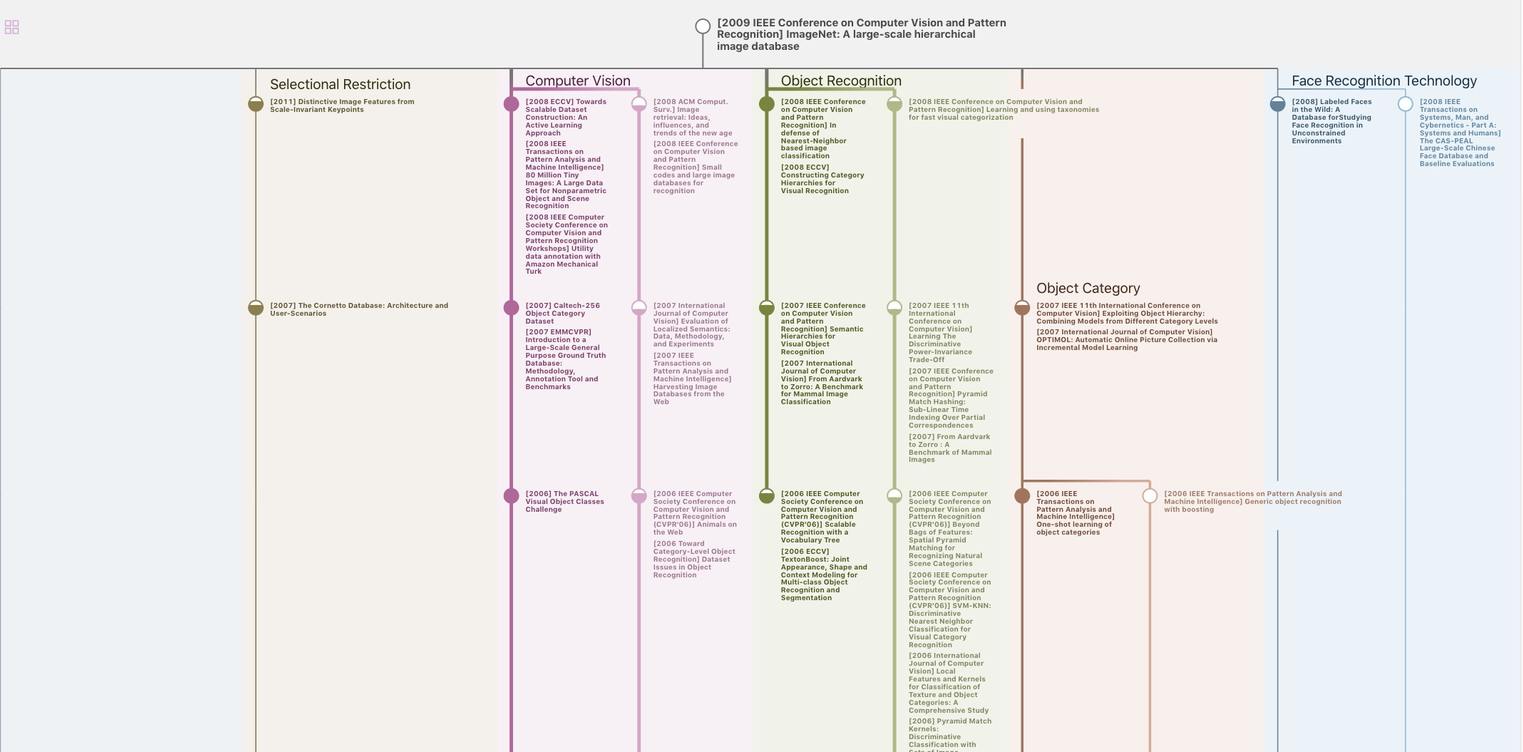
生成溯源树,研究论文发展脉络
Chat Paper
正在生成论文摘要