Learning distributed representations of knowledge that preserve deductive reasoning
Knowledge-Based Systems(2024)
摘要
Deep neural networks have shown promise in various fields including image processing, natural language processing and speech processing. Despite their proven usefulness in both theory and empirical studies, significant difficulties in their application still persist. More specifically, enabling deep neural networks to reason over data for knowledge acquisition is arguably challenging. Neural networks have the ability to learn patterns and generalize over data but often lack model interpretability, i.e., it is not always clear how they reason over data. Enabling neural networks to symbolically reason is one way to bring explainability and improve knowledge acquisition. In this paper, we introduce a Deduction-Preserved Embedding (DPE) model designed to learn a distributed representation that integrates symbolic deductive reasoning. Our approach effectively incorporates symbolic rules, resulting in enhanced link prediction performance for knowledge graph completion. Our experimental results highlight that the proposed model consistently outperforms baseline methods, demonstrating superior overall performance despite having a relatively small number of parameters. Notably, the version of the model that incorporates symbolic rules surpasses the majority of compared models, particularly in scenarios characterized by data sparsity.
更多查看译文
关键词
Visual and textural representation,Knowledge representation,Reasoning
AI 理解论文
溯源树
样例
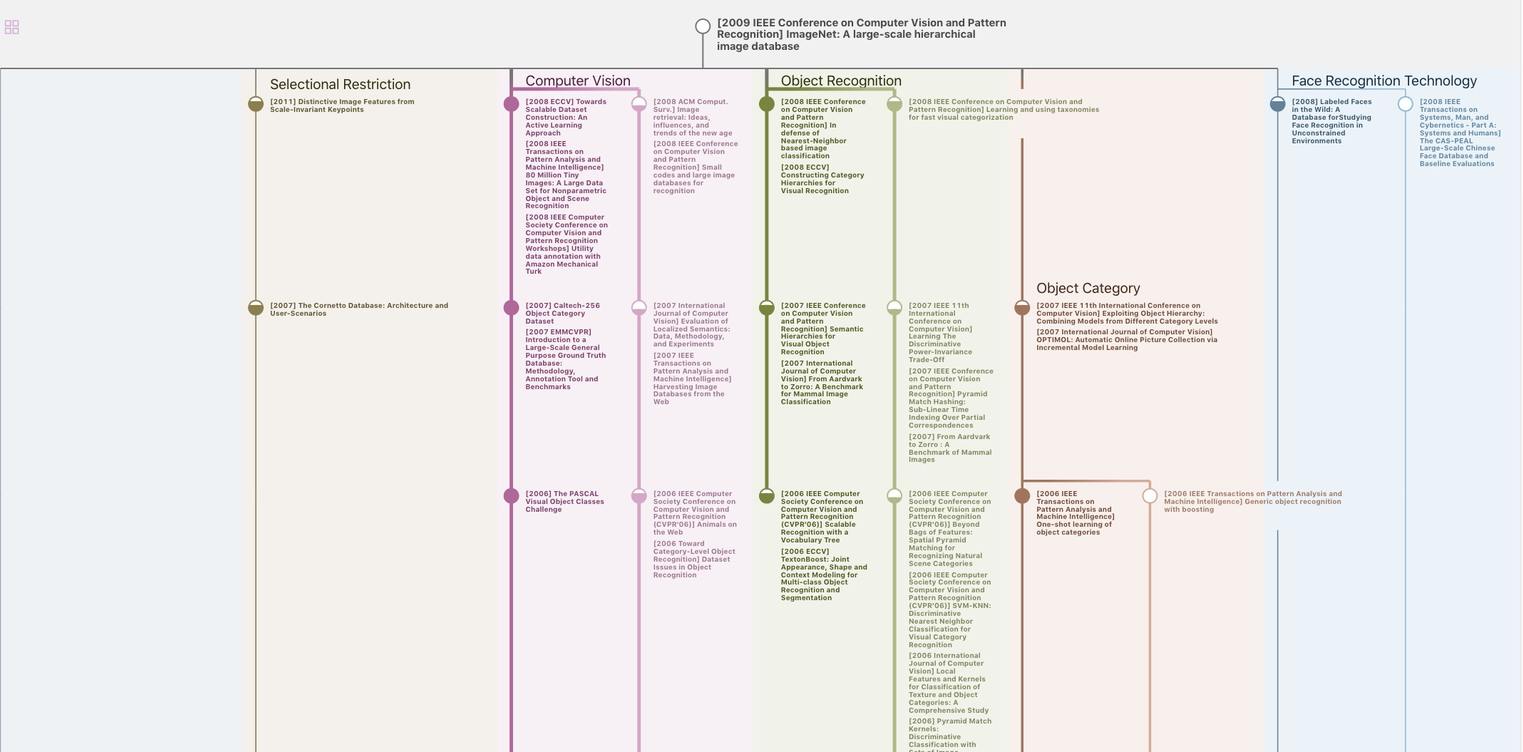
生成溯源树,研究论文发展脉络
Chat Paper
正在生成论文摘要