Subtyping Social Determinants of Health inAll of Us: Opportunities and Challenges in Integrating Multiple Datatypes for Precision Medicine
crossref(2023)
摘要
A.Project BackgroundSocial determinants of health (SDoH), such as financial resource strain and housing instability, account for between 30-55% of people’s health outcomes. While many studies have identified strong associations among specific SDoH and health outcomes, most people experience multiple SDoH in their daily lives. Analysis of this complexity requires the integration of personal, clinical, social, and environmental information from a large cohort of individuals that have been traditionally underrepresented in research, which is only recently being made available through theAll of Usresearch program. However, little is known about the range and response of SDoH inAll of Us, and how they co-occur to form subtypes, which are critical for designing precision medicine interventions.Research Questions(1) What is the range and response to survey questions related to SDoH? (2) How do SDoH co-occur to form subtypes, and what are their risk for adverse health outcomes?MethodsFor Question-1, we analyzed the range of SDoH questions across the surveys with respect to the 5 domains inHealthy People 2030(HP-30), and analyzed their responses across the fullAll of Uscohort (n=372,397, V6). For Question-2, we used the following steps: (1) due to the missingness across the surveys, selected all participants with valid and complete SDoH data, and used inverse probability weighting to adjust their imbalance in demographics, compared to the full cohort; (2) asked three domain experts to group the SDoH questions into SDoH subdomains, for enabling a more consistent granularity; (3) used bipartite modularity maximization to identify SDoH biclusters, their significance, and their replicability; (4) measured the association of each bicluster to three outcomes (depression, delayed medical care, emergency room visits in the last year) using multiple data types (surveys, electronic health records, and zip codes mapped to Medicaid expansion states); and (5) asked three domain experts to infer the subtype labels, in addition to the potential mechanisms that precipitate their adverse health outcomes and interventions to prevent them.ResultsFor Question-1, we identified 110 SDoH questions across 4 surveys, which were categorized into 18 SDoH subdomains, and covered all 5 domains in HP-30. However, the results also revealed a large degree of missingness in survey responses (1.76%-84.56%), with later surveys having significantly fewer responses compared to earlier ones, and significant differences in race, ethnicity, and age of participants when compared to the full cohort. For Question-2, the subtype analysis (n=12,913, d=18) identified 4 biclusters with significant biclusteredness (Q=0.13, random-Q=0.11, z=7.5,P<0.001), and significant replication (Real-RI=0.88, Random-RI=0.62,P<.001). Furthermore, there were significant associations between specific subtypes and the outcomes and with Medicaid expansion, each with meaningful interpretations and potential precision interventions. For example, the subtypeSocioeconomic Barriersincluded the SDoH subdomainsemployment, food security, housing, income, literacy, andeducation attainment, and had a significantly higher odds ratio (OR=4.2, CI=3.5-5.1,P-corr<.001) for depression, when compared to the subtypeSociocultural Barriers. Individuals that match this subtype profile could be screened early for depression and referred to social services for addressing combinations of SDoH such as housing and income. Finally, the identified subtypes spanned one or more HP-30 domains revealing the difference between the current knowledge-based SDoH domains and the data-driven subtypes. These results reflect the complexity of how SDoH co-occur in the real world, and their potential use in the design of models to predict adverse health outcomes, and the design of interventions.Community ImpactWhile several SDoH models including the Dahlgren-Whitehead conceptual model have identified SDoH domains, they have emphasized that real-world SDoH span multiple domains with complex interactions and feedback loops. However, this phenomenon has been difficult to analyze given the lack of large cohorts of individuals that have been traditionally underrepresented in biomedical research, and characterized by a wide range of SDoH and datatypes. The results from analyzing SDoH using theAll of Uscohort provided direct evidence for this real-world phenomenon by showing that data-driven SDoH subtypes span one or more of the SDoH domains defined by HP-30. This result provides testable hypotheses for future studies that SDoH models based on data-driven subtypes will be more accurate and interpretable for predicting adverse health outcomes, when compared to models that do not use those subtypes. Furthermore, the characterization of the range and response to SDoH across the entireAll of Uscohort using over one hundred SDoH, should enable researchers to use the approach for characterizing other cohorts to identify and address missingness. Finally, our workbench which focuses on subtyping SDoH, provides generalizable and scalable machine learning methods that can be used to periodically rerun the analysis as theAll of Uscohort continues to evolve.
更多查看译文
AI 理解论文
溯源树
样例
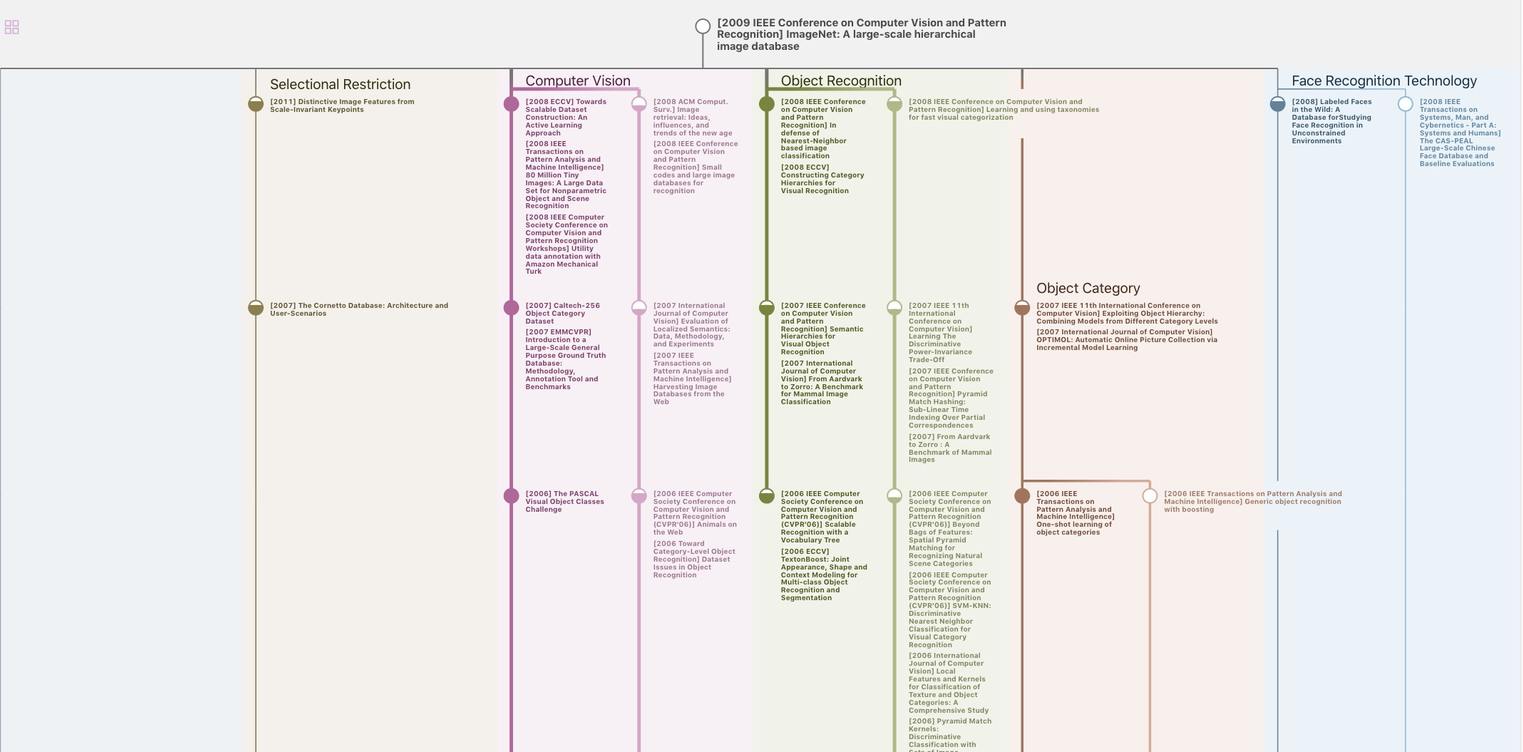
生成溯源树,研究论文发展脉络
Chat Paper
正在生成论文摘要