Potential for Using Deep Learning for Digital-Twin System Validation Testing
2022 IEEE/AIAA 41st Digital Avionics Systems Conference (DASC)(2022)
摘要
One of the challenges in designing and operating systems composed of interacting components is validating that the emergent behavior of the system does not cause one or more components to migrate, over time, into a hazardous operating state. Many modern airline accidents can be characterized as Interaction Accidents – no component failed, but the interaction of components resulted in a hazardous state.Due to the dependence of time, emergent behavior cannot be evaluated by analysis of the design. In theory it can be evaluated by Digital-Twin agent-based simulations. However, the running these simulations to uncover rare event emergent hazardous states is prohibitive due to: (1) the combinatorics of initial states of each of the components, and the (2) combinatorics of the time dimension (i.e. small variations in timing can result in very different outcomes). Deep Learning Neural Networks (DLNN) have shown promise to capture the underlying combinatoric behavior as well as compress the time dimension.This paper demonstrates the application of DLNN to identify emergent behavior from components with hybrid moded/continuous behavior that plays out over time. DLNNs were trained and tested for three systems with increasing behavioral complexity. The DLNNs accurately were able to represent the time dependent behavior for which they were trained/tested. The DLNNs were also able to learn and predict emergent behavior for behaviors that were not included in the training/testing data (up to 63% of the missing cases). The implications of these results are discussed.
更多查看译文
关键词
Deep Learning Neural Networks,Validation Testing,Emergent behavior,agent-based modeling. Digital-twin
AI 理解论文
溯源树
样例
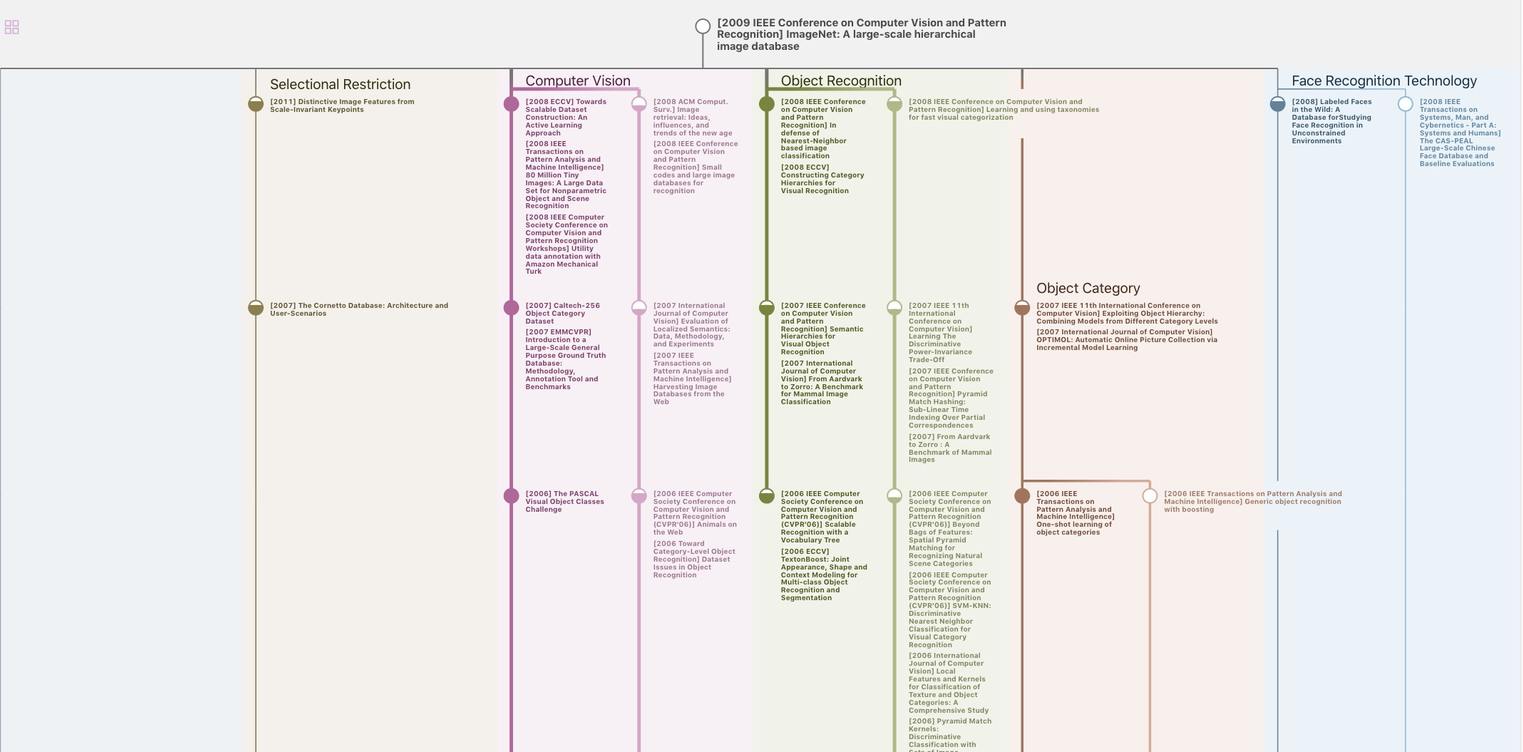
生成溯源树,研究论文发展脉络
Chat Paper
正在生成论文摘要